Hugging Face releases visual language models 'Smolvlm-256m' and 'Smolvlm-500m' that run on laptops with less than 1GB of memory
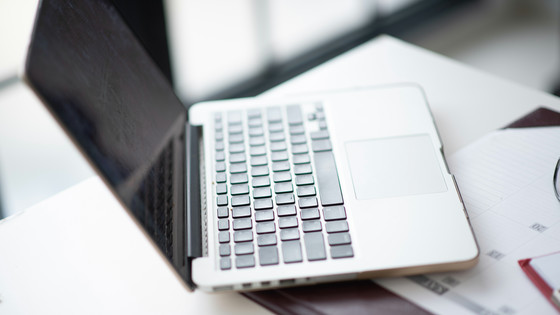
AI development platform Hugging Face has released the smallest AI models capable of analyzing images, short videos, and text: ' SmolVLM-256M ' and ' SmolVLM-500M '. According to Hugging Face, these two models are designed to work properly even on laptops with less than 1GB of memory.
SmolVLM Grows Smaller – Introducing the 256M & 500M Models!
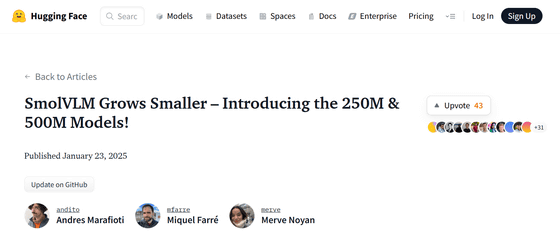
Hugging Face claims its new AI models are the smallest of their kind | TechCrunch
https://techcrunch.com/2025/01/23/hugging-face-claims-its-new-ai-models-are-the-smallest-of-their-kind/
According to Hugging Face, SmolVLM-256M has 256 million parameters, making it the world's smallest visual language model (VLM). SmolVLM-500M also has 500 million parameters, making it an ultra-lightweight VLM.
The SmolVLM-256M is the smallest VLM available, but it can handle many multimodal tasks, such as image captioning, text responses, and basic visual reasoning. The larger SmolVLM-500M, despite being smaller than the 2B model, outperforms a variety of tasks and provides more robust responses to prompts.
According to Hugging Face, a comparative experiment with SigLIP 400M SO (400 million parameters) used in the conventional SmolVLM 2B and other large-scale VLMs showed only slight performance improvement, so the smaller SigLIP base patch-16/512 (93 million parameters) encoders in SmolVLM-256M and SmolVLM-500M are used. In addition, inspired by Apple's VLM research and Google's PaliGemma , it is now possible to process images at higher resolutions.
Below is a table comparing the benchmark results of SmolVLM-256M and SmolVLM-500M with SmolVLM-2.2B and Idefics 80B developed by Hugging Face. Hugging Face claims that the smaller SmolVLM-256M outperforms Idefics 80B in some tasks, such as 52.6% in OCRBench and 49.9% in TextVQA .
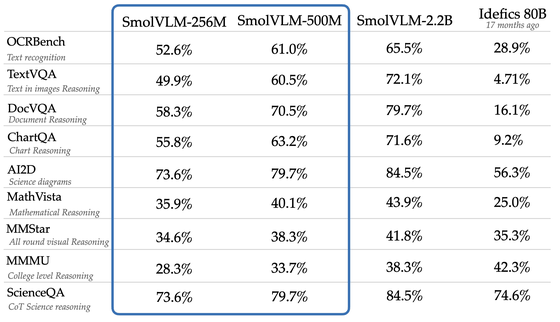
In addition, Hugging Face has revealed that a model called 'ColSmolVLM' has been developed by a team developing a ColBERT -like search model. ColSmolVLM has performance comparable to models 10 times larger than itself, achieving state-of-the-art multimodal search speeds. Hugging Face says that ColSmolVLM is easy to fine-tune and experiment with, making it possible to build searchable databases faster and at lower cost.
Hugging Face is also working with IBM to develop a model for Docking , and early experimental results using the 256M model have shown impressive results. It is expected that future updates will be available.
The SmolVLM 256M and SmolVLM 500M models can be downloaded below.
SmolVLM 256M & 500M - a HuggingFaceTB Collection
https://huggingface.co/collections/HuggingFaceTB/smolvlm-256m-and-500m-6791fafc5bb0ab8acc960fb0
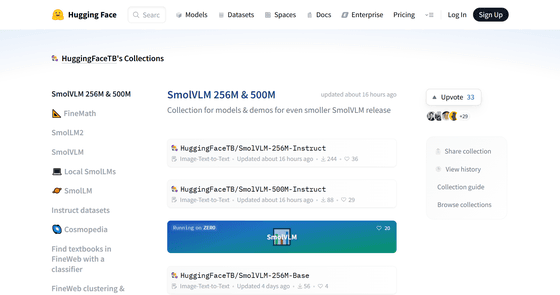
In addition, a demo site where you can try out SmolVLM 256M and SmolVLM 500M from your browser is available at the following link. This demo site uses WebGPU, which enables high-speed graphics processing on the browser, for inference calculations.
SmolVLM 256M Instruct WebGPU - a Hugging Face Space by HuggingFaceTB
https://huggingface.co/spaces/HuggingFaceTB/SmolVLM-256M-Instruct-WebGPU
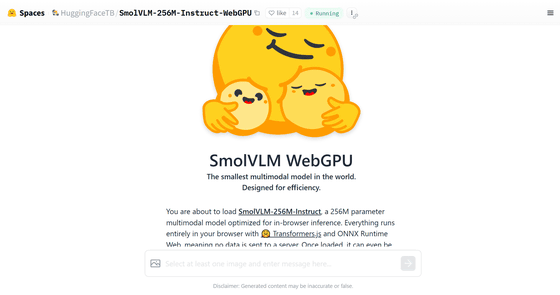
SmolVLM 500M Instruct WebGPU - a Hugging Face Space by HuggingFaceTB
https://huggingface.co/spaces/HuggingFaceTB/SmolVLM-500M-Instruct-WebGPU
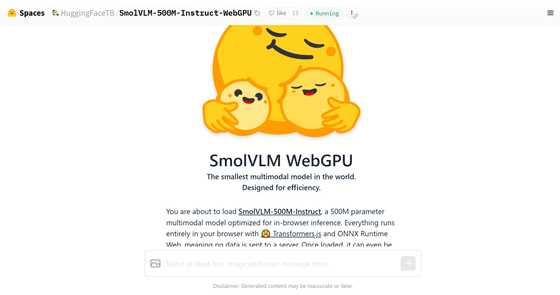
In addition, IT news site TechCrunch points out that 'Small models like the SmolVLM-256M and SmolVLM-500M may be cheap and have a variety of features, but they may have defects that are not as noticeable in larger models.'
Related Posts:
in Software, Posted by log1i_yk