AMD releases Instella, an open source language model trained on AMD GPUs, outperforming comparable models
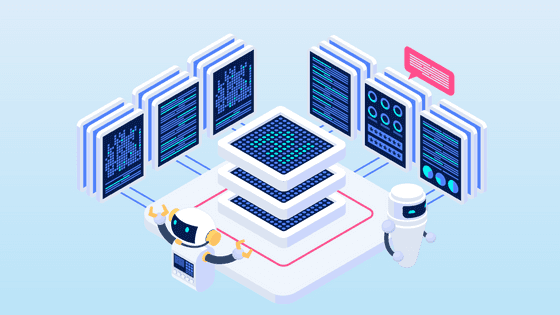
AMD has announced the open source language model ' Instella '. Instella is a 3 billion parameter model trained using the AMD Instinct MI300X GPU, and is said to perform competitively against state-of-the-art open weight models such as Llama-3.2-3B, Gemma-2-2B, and Qwen-2.5-3B.
Introducing Instella: New State-of-the-art Fully Open 3B Language Models — ROCm Blogs
https://rocm.blogs.amd.com/artificial-intelligence/introducing-instella-3B/README.html
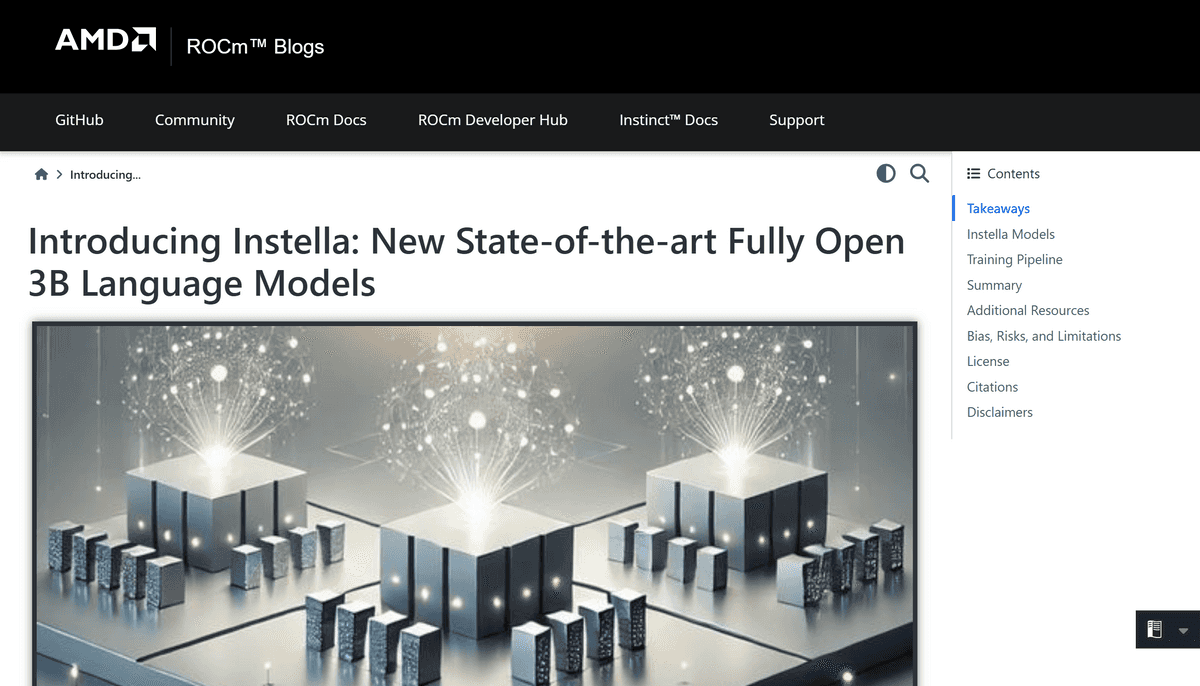
Instella is a text-only Transformer-based language model with 3 billion parameters, 36 decoder layers, and 32 attention heads per decoder layer. It can handle sequences of up to 4096 tokens and has a vocabulary size of approximately 50,000 tokens.
Instella was trained on 4.15 trillion tokens of data using 128 AMD Instinct MI300X GPUs. It outperformed existing fully open models in terms of the balance between the number of tokens used for training and performance, and also performed comparable to state-of-the-art open weight models.
The figure below compares Instella with state-of-the-art open weight models such as Llama-3.2-3B, Gemma-2-2B, and Qwen-2.5-3B, with the vertical axis representing the average benchmark score and the horizontal axis representing the number of tokens used in training. Instella outperforms existing models in the comparison of pre-trained models on the left, and Instella is on par with existing models in the comparison of instruction-adjusted models on the right.
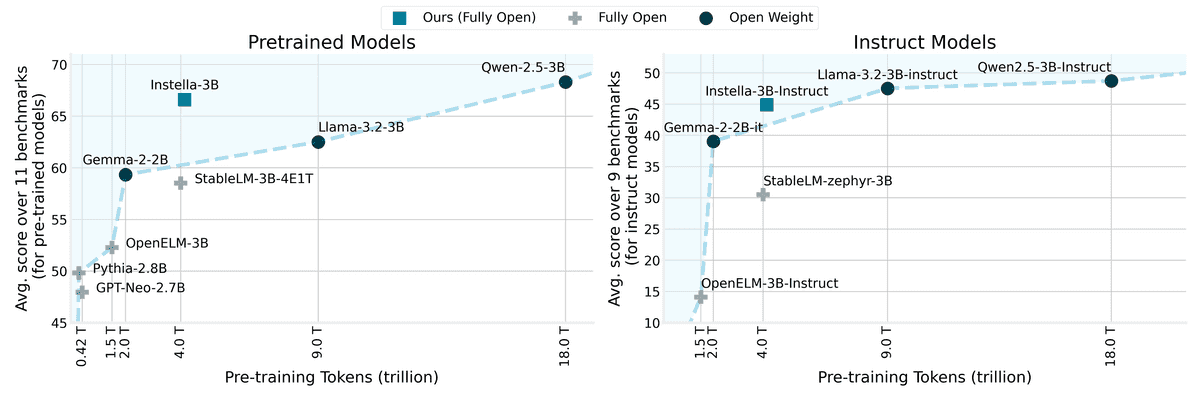
Specific benchmark results for the instruction-adjusted model are below, with the best number in each benchmark in bold and the second best number underlined.
Models | Size | Training Tokens | Avg | MMLU | TruthfulQA | BBH | GPQA | GSM8K | Minerva MATH | IFEval | AlpacaEval 2 | MT-Bench |
---|---|---|---|---|---|---|---|---|---|---|---|---|
Open Weight Models | ||||||||||||
Gemma-2-2B-Instruct | 2.61B | ~2T | 39.04 | 58.35 | 55.76 | 42.96 | 25.22 | 53.45 | 22.48 | 55.64 | 29.41 | 8.07 |
Llama-3.2-3B-Instruct | 3.21B | ~9T | 47.53 | 61.50 | 50.23 | 61.50 | 29.69 | 77.03 | 46.00 | 75.42 | 19.31 | 7.13 |
Qwen2.5-3B-Instruct | 3.09B | ~18T | 48.72 | 66.90 | 57.16 | 57.29 | 28.13 | 75.97 | 60.42 | 62.48 | 22.12 | 8.00 |
Fully Open Models | ||||||||||||
StableLM-zephyr-3B | 2.8B | 4T | 30.50 | 45.10 | 47.90 | 39.32 | 25.67 | 58.38 | 10.38 | 34.20 | 7.51 | 6.04 |
OpenELM-3B-Instruct | 3.04B | ~1.5T | 14.11 | 27.36 | 38.08 | 24.24 | 18.08 | 1.59 | 0.38 | 16.08 | 0.21 | 1.00 |
Instella-3B-SFT | 3.11B | ~4T | 42.05 | 58.76 | 52.49 | 46.00 | 28.13 | 71.72 | 40.50 | 66.17 | 7.58 | 7.07 |
Instella-3B-Instruct | 3.11B | ~4T | 44.87 | 58.90 | 55.47 | 46.75 | 30.13 | 73.92 | 42.46 | 71.35 | 17.59 | 7.23 |
The training pipeline is as follows: First, the first stage of pre-training was performed on 4 trillion tokens of data, followed by a second stage of pre-training on 58 billion tokens of data to enhance multi-stage reasoning and mathematical capabilities. After that, 26.7 billion tokens of instruction-response pair data were used to improve the ability to respond to user queries, and finally, 760 million tokens of data were used for training to improve the usefulness, accuracy, and safety of the output.
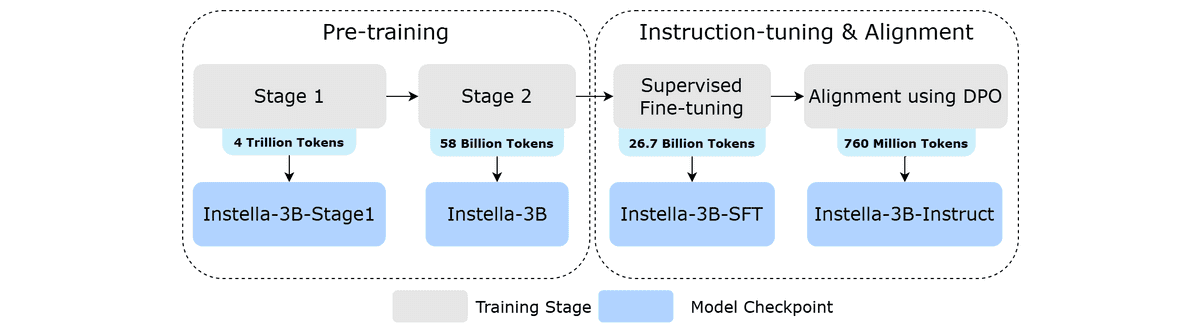
Instella is a 'fully open and accessible model,' with training hyperparameters, datasets, and code used
publicly available. Checkpoints for each stage of training are also available for download from Hugging Face .
AMD commented that through its work with Instella, 'we have demonstrated the feasibility of training language models on AMD GPUs.' The company plans to continue improving the model in multiple aspects, including context length, inference capabilities, and multimodal capabilities.
Related Posts:
in Software, Posted by log1d_ts