NVIDIA B200 and Google Trillium appear on MLPerf benchmark charts, B200 achieves 2x performance over H100
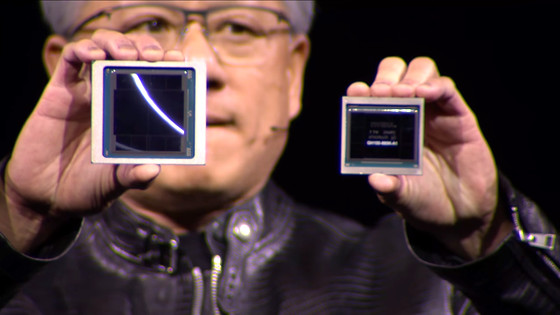
The AI benchmark MLPerf Ver.4.1 has been released, revealing the results of new AI chips such as NVIDIA B200 and Google Trillium . According to the report, NVIDIA's next-generation GPU, B200, achieved twice the performance in some tests compared to the current H100, while Google's new accelerator, Trillium, showed about four times the performance improvement compared to the chips tested in 2023.
New MLPerf Training v4.1 Benchmarks Highlight Industry's Focus on New Systems and Generative AI Applications - MLCommons
https://mlcommons.org/2024/11/mlperf-train-v41-results/
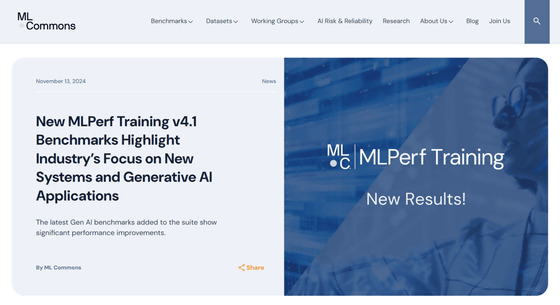
AI Training:Newest Google and NVIDIA Chips Speed AI Training - IEEE Spectrum
https://spectrum.ieee.org/ai-training-2669810566
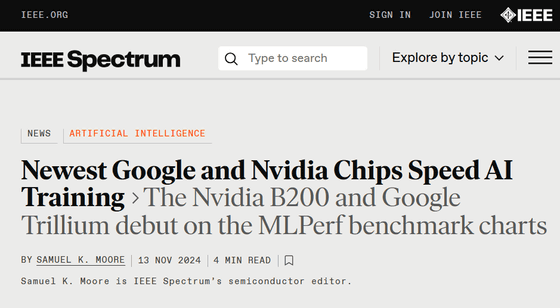
MLPerf v4.1 is an AI benchmark consisting of six tasks: recommendation, pre-training with GPT-3 and BERT-large, fine-tuning with Llama 2 70B, object detection, graph node classification, and image generation. MLPerf v4.1 introduces a new benchmark based on a model architecture called ' Mixture of Experts (MoE)' for the first time. MoE is designed to use multiple small 'expert' models instead of a single large model, and uses the open source Mixtral 8x7B model as a reference implementation.
The benchmark received 964 performance results from 22 organizations, among which six processors appeared in the benchmark test for the first time: AMD's MI300x, AMD's EPYC Turin, Google's Trillium TPUv6e, Intel's Granite Rapids Xeon, NVIDIA's Blackwell B200, and UntetherAI's SpeedAI 240 series.
NVIDIA's Blackwell B200 uses a method to improve processing speed by reducing the calculation precision from 8 bits to 4 bits, and it has achieved about twice the performance of the H100 in GPT-3 training and fine-tuning of LLM on a GPU basis. It also achieved a performance improvement of 64% and 62% in recommender systems and image generation, respectively.
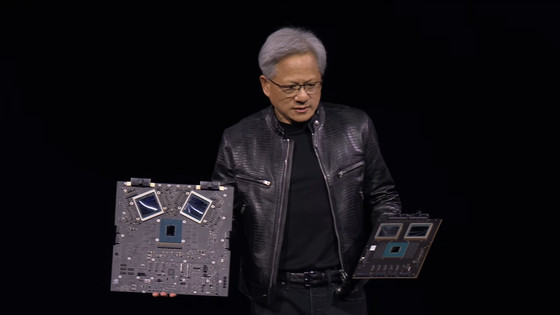
Meanwhile, Google's sixth-generation TPU, Trillium, showed up to 3.8x performance improvement on GPT-3 training tasks compared to its predecessor, but struggled to compete with NVIDIA: It took about 3.44 minutes for a 11,616-core NVIDIA H100 system to reach a checkpoint in GPT-3 training, compared to 11.77 minutes for a 6,144-core TPU v5p system.
In terms of power consumption, Dell's system was measured running a Llama 2 70B fine-tuning task, using eight servers, 64 NVIDIA H100 GPUs, and 16 Intel Xeon Platinum CPUs, and consumed 16.4 megajoules over a five-minute period, translating to an average U.S. electricity cost of about 75 cents.
In response to these benchmark results, the Institute of Electrical and Electronics Engineers ( IEEE ) argued that 'AI training performance is improving at about twice the pace of Moore's Law, but the rate of performance improvement has slowed compared to before. This is because companies have already achieved optimization of benchmark tests on large-scale systems, and advances in software and network technology have made it possible to linearly reduce processing time by increasing the number of processors,' and stated that in the future, emphasis will likely be placed on efficiency and optimizing power consumption.
Related Posts: