Technical report of improved version of Stable Diffusion 'Stable Diffusion XL (SDXL)' released
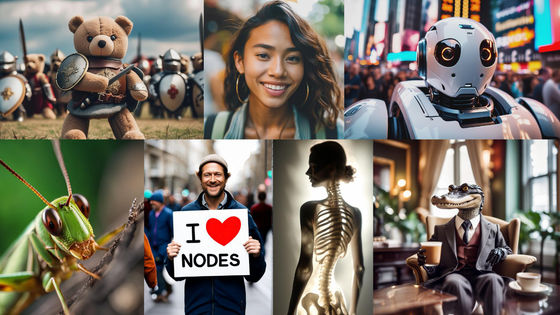
A technical report of the new model ``
generative-models/assets/sdxl_report.pdf at main Stability-AI/generative-models GitHub
https://github.com/Stability-AI/generative-models/blob/main/assets/sdxl_report.pdf
Stable Diffusion XL technical report [pdf] | Hacker News
https://news.ycombinator.com/item?id=36586079
SDXL has a UNet backbone that is three times larger than traditional Stable Diffusion. The increase in model parameters allows SDXL to take advantage of attention blocks and large cross-attention contexts, which allow SDXL to train in multiple aspect ratios.
In addition, to improve the visual fidelity of the samples generated by SDXL, the model is modeled using the technique 'image-to-image (img2img)', in which images are input as prompts to generate new images. It seems to be improving.
The graph below summarizes how well the images created by the image generation AI match the user's preferences. It means that the higher the vertical axis, the more favorable the user's image was generated. SDXL has already demonstrated significantly better performance than Stable Diffusion 1.5 and 2.1, and we have shown that adding refinement stages (SDXL w/Refiner) can further improve performance. rice field.
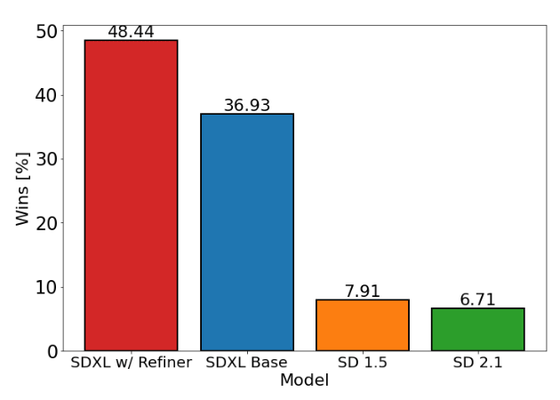
Also, previous versions of Stable Diffusion often produced choppy images where the subject's head and feet were cropped out of the frame. This is because the training data includes an arbitrary trimmed image (an image in which part of the subject does not fit in the frame correctly), so that the AI can create an ``image in which the subject's head and feet are cut out of the frame''. This is because I remembered
In order to solve this problem, SDXL uses random cropped images during training and makes the AI model recognize that ``the image used for training has been partially cropped''. As you can see, I'm adding coordinates to the model. By adding coordinate information, the AI model seems to be able to generate images of various sizes while keeping the subject in the center.
Below is a comparison of the images generated by Stable Diffusion versions 1.5, 2.1, and SDXL with the same prompt. On the left, I generated an image with the prompt 'a propaganda poster with a cat dressed as French Napoleon holding cheese', and on the right, I generated an image with the prompt 'fire-breathing dragon up, cinematic shot'. if generated. In Stable Diffusion versions 1.5 and 2.1, there are cases where cats and dragons are cut off, but in SDXL you can see that cats and dragons are neatly contained in the frame.
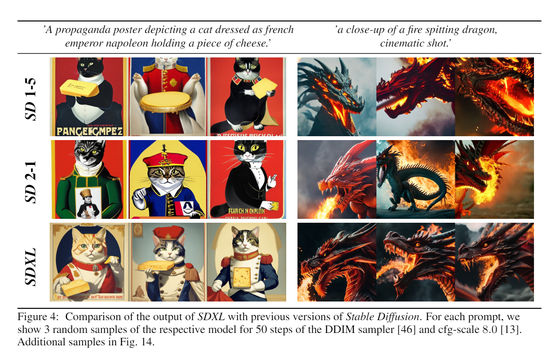
SDXL employs a two-stage model rather than a single monolithic model. One of the two-stage models is ``a model trained to obtain the ``most part of the image'', and the other is ``improving the output of the first model, correcting textures and details. model'. These models can be trained separately, which also improves learning efficiency.
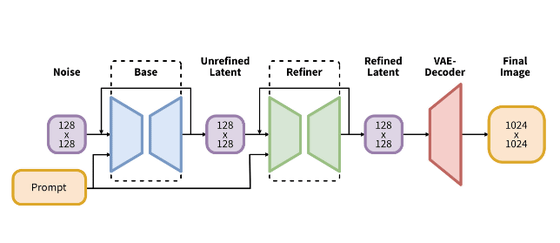
In addition, the technical details of SDXL are summarized in the report, so please check it out if you are interested.
generative-models/assets/sdxl_report.pdf at main Stability-AI/generative-models GitHub
https://github.com/Stability-AI/generative-models/blob/main/assets/sdxl_report.pdf
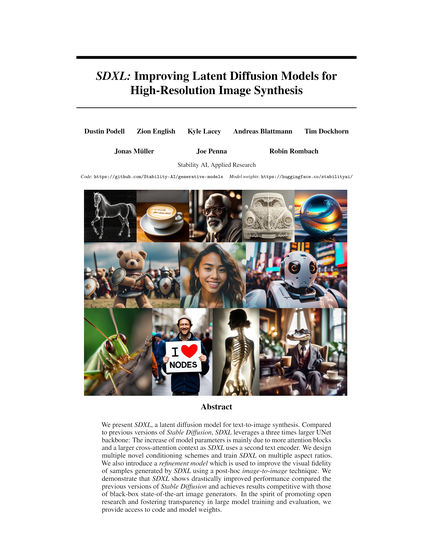
Related Posts:
in Software, Posted by logu_ii