Image generation AI ``Stable Diffusion'' announces a method to generate ``specific image-like ○○'' from just one image in just a few tens of seconds
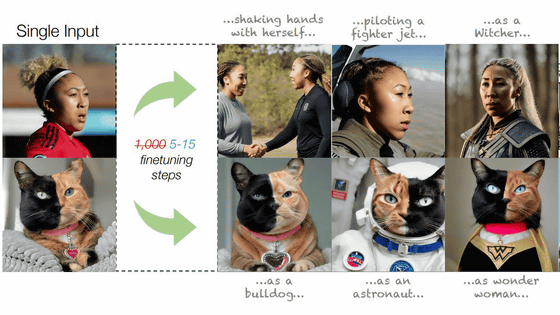
By instructing an image generation AI such as Stable Diffusion to compress a specific image or style into specific words, it is possible to 'optimize' the image you want to generate to closely resemble any image. A team led by Linon Gal, a computer scientist at Tel Aviv University, has announced a method to optimize images with just one image and 5 to 15 steps of adjustment.
[2302.12228] Designing an Encoder for Fast Personalization of Text-to-Image Models
Encoder-based Domain Tuning for Fast Personalization of Text-to-Image Models
https://tuning-encoder.github.io/
One of the techniques that allows Stable Diffusion to optimize images is Textual Inversion. Textual Inversion is a technology also called 'Embeddings' that allows you to generate an image that closely resembles a specific image by simply preparing data learned from the image separately from the Stable Diffusion model data. Become. Textual Inversion simply updates the 'weighting' in keyword vectorization, so the advantage is that it requires relatively little memory for learning.
Explaining the advantages and disadvantages of ``Textual Inversion'', which fine-tunes the image generation AI ``Stable Diffusion'' using several images, with examples - GIGAZINE
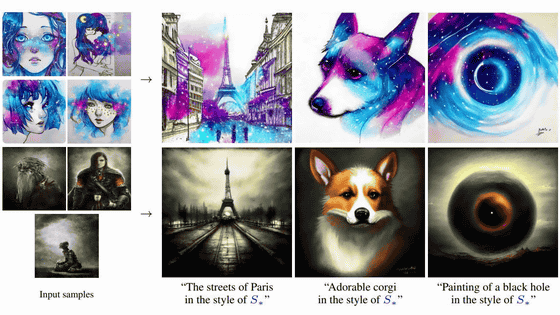
In addition, 'Dream Booth' is an image optimization technology developed for Google's image generation AI ' Imagen '. Unlike Textual Inversion, Dream Booth performs additional training on the model itself to update parameters. A method has been developed to apply this Dream Booth to Stable Diffusion, and for example, anyone can easily execute Dream Booth by using the following tools.
A review of ``Dreambooth Gui'' that allows you to easily use ``Dream Booth'' which can additionally learn picture patterns and painting styles from just a few illustrations on the image generation AI ``Stable Diffusion'' - GIGAZINE
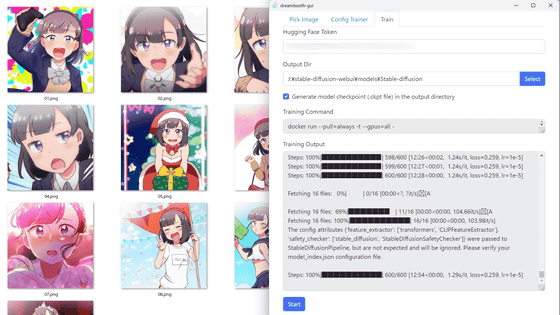
However, Gall et al. pointed out that ``traditional image optimization approaches suffer from problems such as long learning times and high storage requirements,'' and proposed an ``encoder-based domain tuning approach'' to solve these problems. doing.
Stable Diffusion uses a 'text encoder' to output the input text into a 768-dimensional token embedding vector, a 'U-NET encoder' to convert the token embedding vector into noise image information in a latent space, and a 'decoder' to convert the token embedding vector into noise image information in a latent space. ' generates an image by outputting the noise image information to a pixel image. The detailed mechanism is summarized in the article below.
A detailed illustration of how the image generation AI 'Stable Diffusion' generates images from text - GIGAZINE
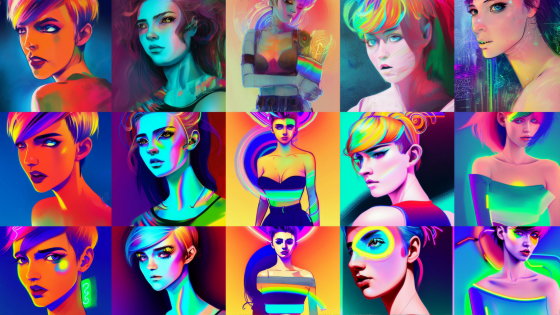
Gal et al.'s approach consists of two steps: adding a single input image and a combination of words representing that image to a text encoder, and then updating the U-NET encoder to change the weighting of the vector. Masu.
Below is a summary of the results of actually loading a researcher's face photo into Stable Diffusion and generating a similar image. The image columns are, from the far left, ``Imported image'', ``Stable Disffusion'', ``Textual Inversion (1 image)'', ``Textual Inversion (5 images)'', ``Dream Booth (1 image)'', Dream Booth (5 images loaded),' 'Textual Inversion + Dream Booth,' and 'Gal et al.'s approach.' It is clear that the images generated by Mr. Gal et al.'s approach are able to reproduce the faces of the people loaded quite faithfully.
In addition, it has been confirmed that Gall et al.'s approach works not only for people's faces, but also for instructing to change the subject without changing the image, or conversely, changing the style of the image without changing the subject. I am.
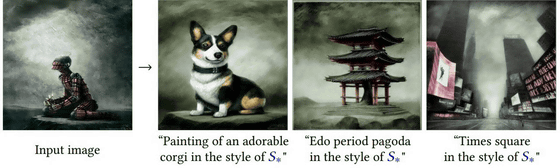
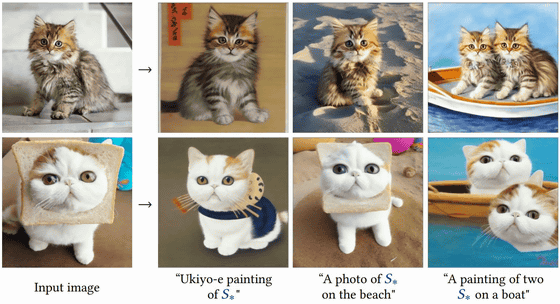
However, according to Gal et al., this encoder-based approach significantly increases the amount of VRAM required. Also, since it is necessary to tune both the text encoder and U-NET encoder at the same time, it seems to require a lot of memory.
At the time of writing, Gal et al. have not released the code for this approach, but they say they plan to release it soon on GitHub.
Related Posts:
in Software, , Posted by log1i_yk