Why did 'Generative AI', which generates images and sentences, develop suddenly?
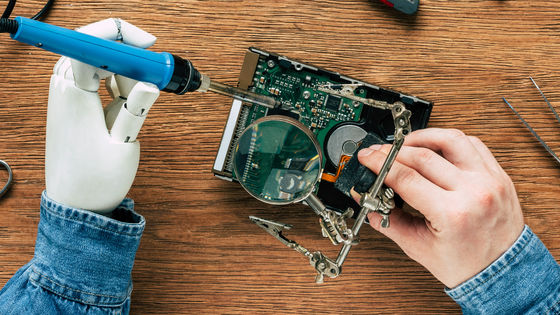
So-called ' generative AI ' such as ' Stable Diffusion ' that can generate highly accurate images simply by inputting sentences (prompts) and ' ChatGPT ' that creates highly accurate sentences interactively are often talked about. Investor and entrepreneur Haomiao Fan explains how generative AI, which seems to have developed rapidly in recent years, is a mechanism and why it is spreading rapidly.
I got interested in how Generative AI actually works, and where the tech came from, so I wrote an article about it. Tl;dr - we are at another of those inflection points where model+data+compute come together to make an ML revolution https://t.co/JzKSJY51DC
— Haomiao Huang (@haomiaoh) January 30, 2023
The generative AI revolution has begun—how did we get here? | Ars Technica
https://arstechnica.com/gadgets/2023/01/the-generative-ai-revolution-has-begun-how-did-we-get-here/
While computers that run artificial intelligence (AI) progress according to Moore's law , which refers to the tendency that the number of transistors on an integrated circuit doubles every 1.5 years, a former engineer at NVIDIA said, ``In fact, Moore AI is progressing at a pace that is five to 100 times faster than the law of AI.'
Artificial intelligence is growing at a pace dozens of times faster than Moore's law - GIGAZINE
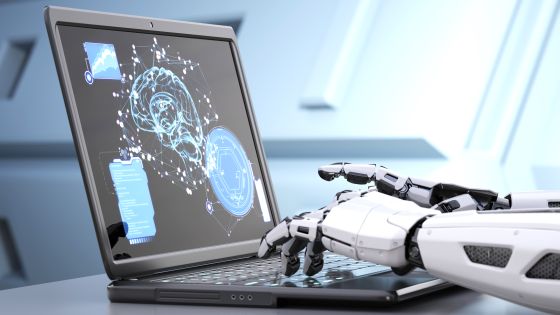
Among such developments in AI, generative AI such as image generation AI and text/program generation AI have been the subject of much discussion in recent years. Mr. Huang points out the importance of large-scale language models (LLM) as a factor in the rapid spread of generative AI as a practical tool beyond research fields. LLM is so flexible and adaptable that it is called the 'basic model' and served as a breakthrough for commercialization of AI systems.
Further tracing the origins of these basic models reveals the explosive spread of AI due to the development of deep learning, and the use of models such as recurrent neural networks (RNN) and long-short-term memory (LSTM) to process and analyze data. I will start with what I was doing. From training as a programming language, RNN and LSTM were used at the stage of transitioning to a learning method that uses natural language processing (NLP) to ``understand the language rather than the code'', but until recently, ``language Fan explains that it was not possible to process long sentences because the order of words makes an important difference in processing 'and 'it is difficult to obtain suitable training data'.
The breakthrough was Google's ' Transformer ', which succeeded in high-quality translation as a neural network architecture that excels at language understanding tasks than RNN. Word order for each language is important for translation, and it was naturally built into Transformer, so it seems that the processing methods used by Transformer, `` positional encoding '' and `` multi-head attention '', functioned as breakthroughs in language processing. .
Google's 'Transformer' that realizes higher quality translation surpasses RNN and CNN - GIGAZINE
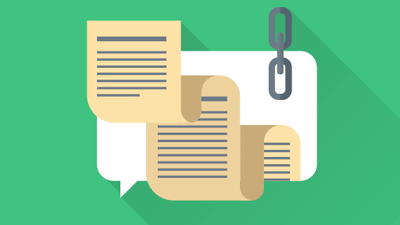
Regarding the breakthrough brought about by Transformer, Mr. Huang said, ``The big turning point in language modeling is the use of an amazing model made for translation, transforming the problem of the language processing task into a translation problem. I found a way to do it,” he said.
OpenAI promotes an approach based on a translation model, and in 2019 released the sentence generation tool `` GPT-2 '', which was said to be ``too dangerous'' because it can create too advanced sentences. GPT-2 was able to generate surprisingly realistic and human-like text, but had some issues, such as the system breaking down quickly as the text got longer and the prompts not being very flexible.
A model of AI's automatic sentence creation tool 'GPT-2', which was said to be 'too dangerous', will be newly released - GIGAZINE
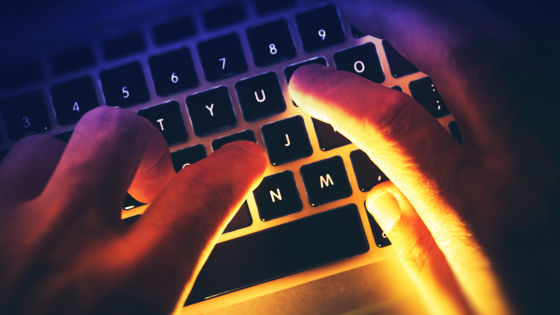
by duallogic
And in 2020, `` GPT-3 '', the successor to GPT-2, appeared, but Mr. Fan points out that ``the innovation from GPT-2 to GPT-3 is the biggest breakthrough.'' . GPT-2 was said to have about 1.5 billion parameters, but GPT-3 has about 175 billion parameters, which is more than 100 times larger, making it possible to write blogs and news that are almost indistinguishable from human text. It became a hot topic that I could write in a consistent sentence.
Furthermore, the advantage of GPT-3 is that it uses a huge number of models, so it can be used in simple sentences such as ``Please summarize the next paragraph'' and ``Please rewrite this paragraph in Hemingway's style''. It was to learn new movements without the need for separate training, just by commanding. And this model will soon spread beyond the field of language processing to other fields.
In the original computer vision research, for example, in order to recognize a 'human face', the process of 'looking at the eyes, mouth, and nose, and recognizing their shape and arrangement pattern' that we actually do. It recognized people's faces by trying to reproduce them. Deep learning has changed this significantly, allowing AI models to learn features and determine whether they correspond to objects such as 'human faces,' 'cars,' and 'animals,' instead of manually manipulating image features as parameters. I made it Deep learning was a powerful model capable of processing a large amount of data, but it was limited to a model that could make judgments as simple fixed phrases, such as ``the image of a cat because it matches the characteristics of a cat.'' Mr. Fan said that he could not correctly understand how to translate between and 'language'.
Transformer was invented as a translation model that converts from one language to another, but if we could figure out how to express other parameters such as images in a similar way to languages, we could find the rules there and translate them into another language. You can train Transformer's ability to map and do translations. In this way, the approach of 'extracting features from an image, compressing them into a low-dimensional representation called a latent representation, and expressing them in coordinates using fine points as axes' has become possible. In addition to the 'image language' and the tool (Transformer) that translates it, OpenAI uses the parameters of GPT-3 to perform natural language processing by building a huge dataset for converting images and text. and image generation to create AI ' DALL・E '.
AI 'DALL E' that automatically generates illustrations and photos from words such as 'Pikachu riding a motorcycle' and 'avocado chair' - GIGAZINE
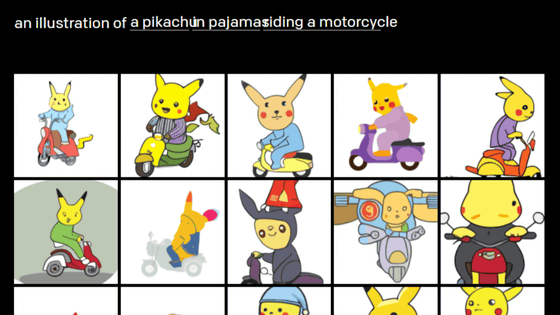
In summary, image generation AI developed as a combination of two AI advances: ``deep learning to learn the language for expressing images'' and ``translation function to switch between the world of text and the world of images''. And while these AI models are also characterized by being data-dependent, unpredictable, and probabilistic, they are fairly powerful and flexible, making them almost universal and applicable to a wide variety of cases, so they can be used in daily life. Fan explains that it has spread to use.
Huang concludes, “Perhaps the only thing we can say is that these AI tools will become more powerful, easier to use, and cheaper. We are in the early stages of the revolution and I am both excited about what is to come and at the same time afraid of what will happen,” he said of his expectations and concerns about the development of AI.
Related Posts:
in Software, Web Service, Posted by log1e_dh