Experts explain what kind of processing is performed by the text generation AI 'GPT-3' developed by OpenAI
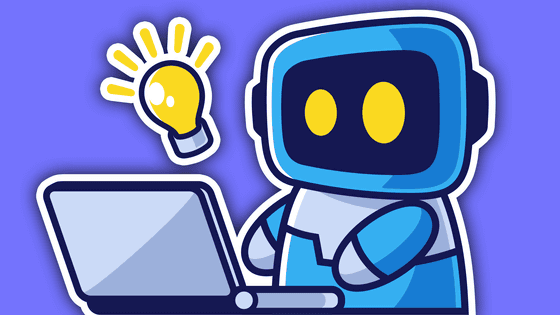
The conversational AI '
The GPT-3 Architecture, on a Napkin
https://dugas.ch/artificial_curiosity/GPT_architecture.html
◆Input/output
First, GPT's 'input' is a series of N words, also called '(input) tokens.' And GPT's 'output' is the word it infers is most likely to be placed at the end of the input token. For example, if you enter 'not all heroes wear', GPT will output 'capes'. At this time, the words 'pants' and 'socks' have also been guessed, but capes is the most likely.
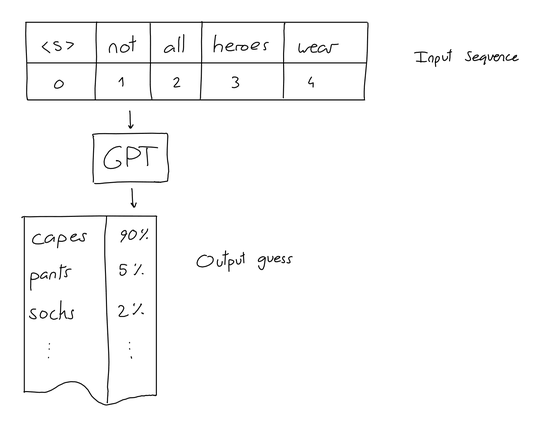
As the input is repeated, the output will change as shown below. If this continues, the length of the input sequence will become infinitely long as more input is made, so in the case of GPT-3, the length of the input sequence is limited to 2048 words.
input | output |
not all heroes wear | capes |
not all heroes wear capes | but |
not all heroes wear capes but | all |
not all heroes wear capes but all | villans |
not all heroes wear capes but all villans | do |
◆Encoding
However, GPT is just an algorithm and does not understand words like humans. In order for the algorithm to recognize a word, it must convert it into a vector of numbers. There are 50,257 tokens registered in GPT and assigned IDs.

In order to improve efficiency, GPT-3 uses '
All tokens are converted to 50,257-dimensional row vectors. For example, in the case of the token [not], the ID is 3673, so it is expressed as a vector in which only the 3673rd component is 1 and the rest are 0. When 2048 tokens are input, they are converted into 2048 50,257-dimensional row vectors, and are finally synthesized into a ``2048 rows x 50,257 columns matrix consisting of 0s and 1s'' as shown below. That is to say. This is the token encoding.
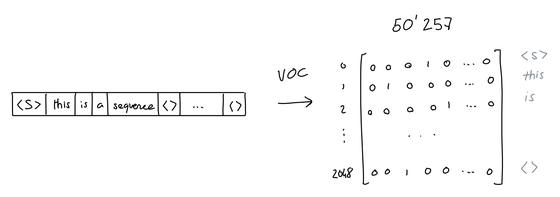
◆Embedding
However, most of the components of the transformed vector are made up of 0. Therefore, instead of a matrix composed of 0 and 1 components, we convert it into a vector whose components are the lengths when
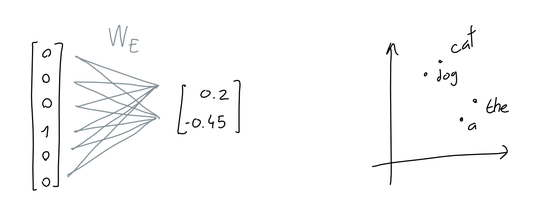
Of course, the actual projected dimension is not 2 dimensions, but GPT uses 12,288 dimensions. Each token vector is multiplied by the trained embedding network weights and converted into a 12,288-dimensional embedding vector.
In other words, multiply the 'matrix of 2,048 rows x 50,257 columns' by the 'matrix of 50,257 rows x 12,288 columns' to convert to 12,288 dimensions, and convert it to an embedding matrix of 2,048 rows x 12,288 columns. Masu.
◆Position encoding
To transform the position of the tokens in the sequence, we order the tokens from 0 to 2047 and pass them through
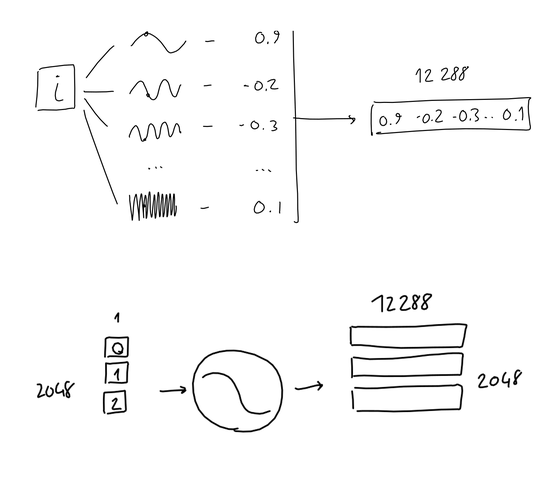
The matrix after position conversion can be added to the token embedding matrix, generating an encoded matrix of 2048 rows and 12,288 columns.
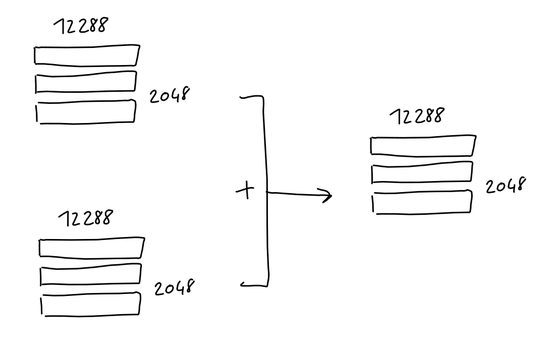
◆Attention
Attention refers to ``which part of the input data do you pay attention to'' and is a very important mechanism in deep learning.
For example, let's say you have a matrix of 3 rows and 512 columns that encodes 3 tokens, and your AI model is learning 3 linear projections. These three linear projections generate three types of 3-by-64 matrices from the three encoding matrices.
Two of these matrices are called query (Q) and key (K). A 3-row x 3-column matrix (Q·K T ), which is generated by multiplying Q and K's
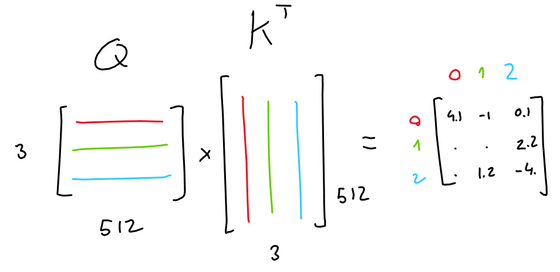
Then, by multiplying Q K T by the third matrix of 3 columns x 512 rows, Value (V), a matrix of 3 rows x 512 columns weighted by the importance of each token is generated. Masu.
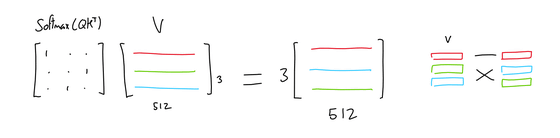
The GPT model uses a mechanism called 'multi-head attention.' Specifically, 'Q, K, and V of 2048 rows and 128 columns are generated from the encoded matrix of 2048 rows and 12,288 columns by three types of linear projection, and (Q, K T ), and V = 2048 rows. By repeating the process of 'generating a matrix of ×128 columns' 96 times and concatenating them, a matrix of 2048 rows × 12,288 columns is generated. This matrix is further linearly projected to generate a new attentioned matrix with 2048 rows and 12,288 columns.
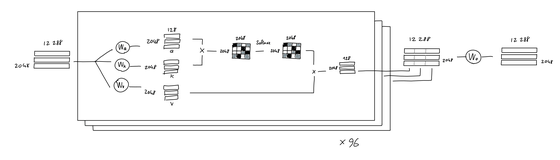
◆Feedforward
Feetforward is
In the case of GPT-3, it has a middle layer with a size of 4 x 12,288 = 49,152, but if you input a matrix of 2,048 rows x 12,288 columns, the output is the same 2,048 rows x 10,000. This is column 2248.
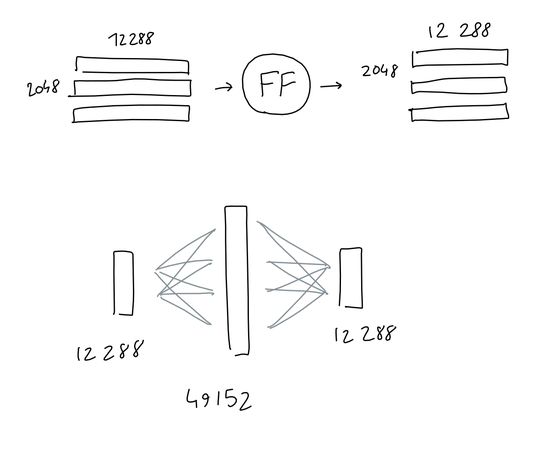
After multi-head attention and feedforward, respectively, we add each block's input to its output and
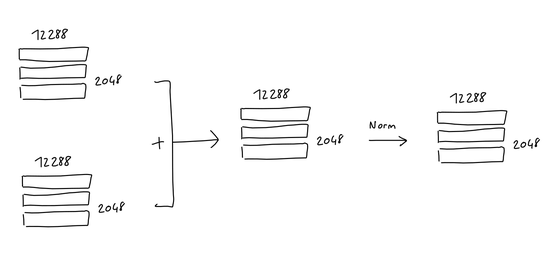
◆Decryption
A matrix of 2048 rows and 12,288 columns that has been regularized through attention and feedforward contains information about which word should appear at each of the 2048 output positions as 12288 vectors. There should be. Here, perform the reverse of the process performed for 'embedding' and convert it to a matrix of 2048 rows x 50,257 columns.
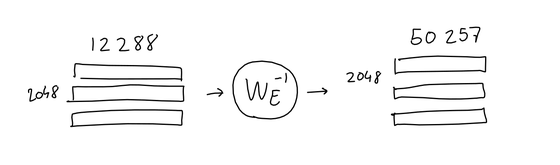
Although this method does not yield a matrix consisting only of 0s and 1s like the matrix derived from the initial input sequence, it can be converted into a probability distribution by
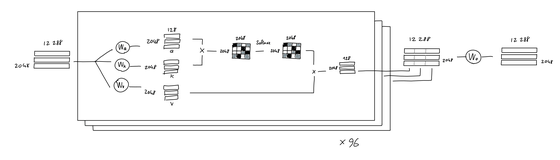
As mentioned above, GPT is able to process languages by going through a complex mathematical process. In addition, Mr. Dugas' diagram summarizing the GPT processing process can be seen below.

Related Posts: