What did we find out by comparing the performance of the chips 'H100', 'H200' and 'MI300X' used in AI training?
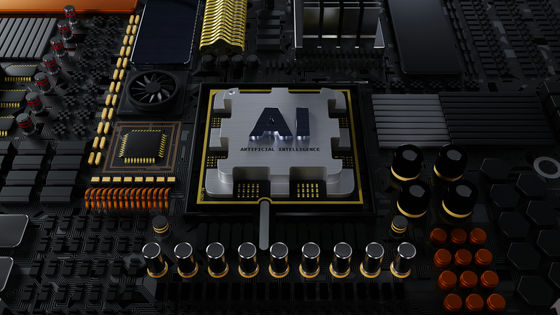
In the market for AI infrastructure used for AI learning and inference, NVIDIA's AI-specialized chips such as 'H100' and 'H200' have a large share. Meanwhile, AMD, a rival of NVIDIA, also announced the '
MI300X vs H100 vs H200 Benchmark Part 1: Training – CUDA Moat Still Alive – SemiAnalysis
https://semianalysis.com/2024/12/22/mi300x-vs-h100-vs-h200-benchmark-part-1-training/
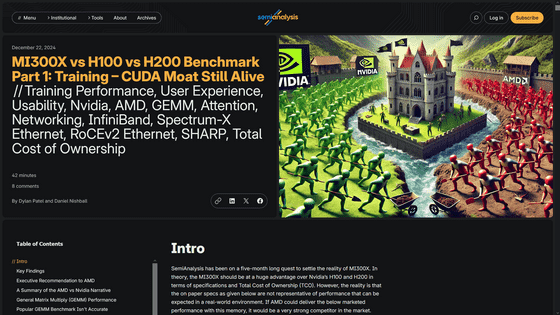
AMD's 'MI300X', announced in December 2023, has approximately 40% more computing units, 1.5 times the memory capacity, and 1.7 times the peak theoretical memory bandwidth than the previous generation Instinct MI250X, and has been touted as having an advantage over competing products such as NVIDIA's H100.
In fact, the following table compares the thermal design power (TDP), watts per GPU, memory capacity, memory bandwidth, FP16, BF16, and processing performance in FP8, FP6, and Int8 for the H100, H200, and MI300X. The TDP of the H100 and H200 is 700, the TDP of the MI300X is 750, and the watts per GPU are the same. The memory capacity of the H100 is 80GB, the memory capacity of the H200 is 141GB, and the memory capacity of the MI300X is 192GB. The memory bandwidth of the H100 and H200 is 3352GB/s and 4800GB/s, respectively, and the MI300X is 5300GB/s. Furthermore, the FP16 and BF16 processing performance of the NVIDIA chip is 989 TFLOPS, the MI300X is 1307 TFLOPS, and the FP8, FP6, and Int8 processing performance of the H100 and H200 is 1979 TFLOPS, and the MI300X is 2615 TFLOPS, showing that the MI300X has higher performance.
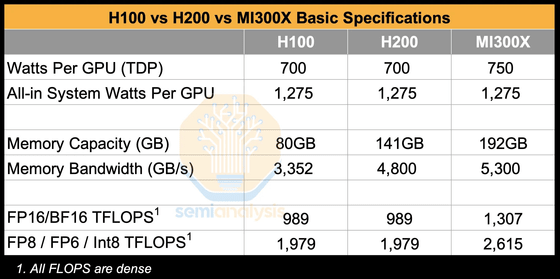
Semianalysis conducted various benchmark tests using these chips. For example, in the GEMM performance test, which is an index to see how cutting-edge chat AI using
Meanwhile, it is widely known that the MI300X has a bandwidth of 5.3TB/s compared to 4.8TB/s for the H200 and 3.35TB/s for the H100. Tests using Tensor.copy_ in Pytorch confirmed that the MI300X has much better memory bandwidth than the H100 and H200.
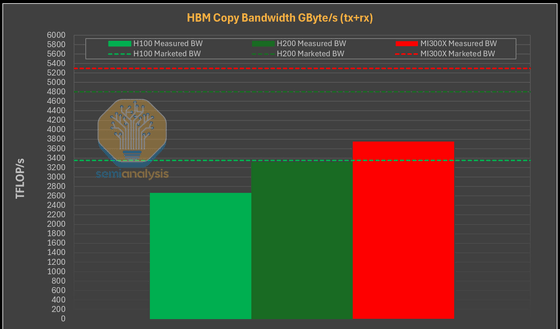
However, single-node training performance tests on these chips reveal that the H100 and H200, shown in green, outperform multiple versions of the MI300X, shown in red and brown.
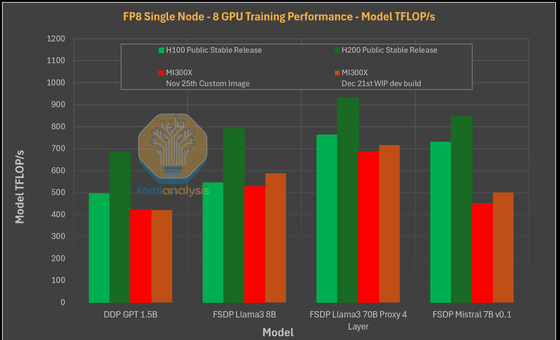
It has also been pointed out that the MI300X performed relatively poorly on small models such as GPT-2 1.5B and models such as Mistral 7B v0.1. According to Semianalysis, while FlexAttention , which optimizes performance, worked on NVIDIA AI chips, FlexAttention did not work fully on AMD chips at the time of writing. Therefore, it has been reported that the H100 and H200 performed more than 2.5 times faster than the MI300X build as of November 25, 2024.
Additionally, a comparison of multi-node training performance showed that the H100 is about 10-25% faster than the MI300X, with Semianalysis stating that 'this difference widens as more nodes collaborate on a single training workload.'
Regarding the results of this benchmark test, Semianalysis said, 'The reason why the MI300X benchmark results, which should have higher performance than NVIDIA chips, were poor is that AMD's software is impeding the performance of the MI300X,' and 'AMD needs to focus on improving its software in order to truly challenge NVIDIA in the field of training workloads.'
Related Posts: