Researchers warn that a ``loop in which AI learns AI-generated content'' has occurred due to the rapid increase in AI artifacts, and ``model collapse'' is occurring
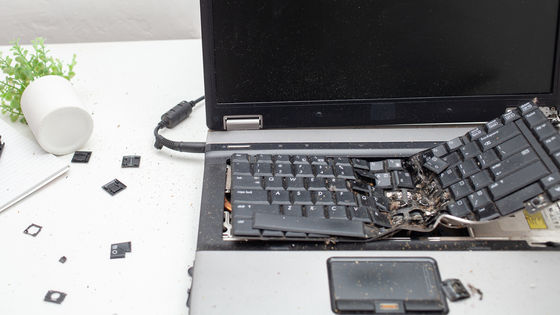
Adobe has announced the image generation AI '
[2305.17493] The Curse of Recursion: Training on Generated Data Makes Models Forget
https://doi.org/10.48550/arXiv.2305.17493
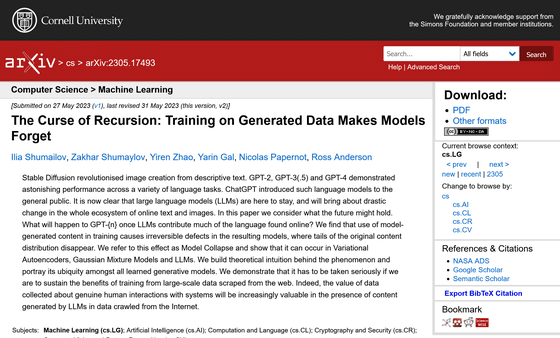
The AI feedback loop: Researchers warn of 'model collapse' as AI trains on AI-generated content | VentureBeat
https://venturebeat.com/ai/the-ai-feedback-loop-researchers-warn-of-model-collapse-as-ai-trains-on-ai-generated-content/
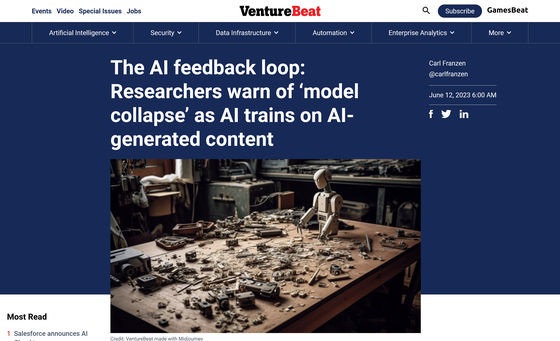
The rapid spread of “generative AI” such as “ Stable Diffusion ” that can generate highly accurate images simply by entering sentences (prompts) and “ ChatGPT ” that creates highly accurate sentences in an interactive format is due to the large scale. A language model (LLM) plays an important role. Combined with the flexible and adaptable LLM, by collecting a large amount of training data, the AI learns the structure of images and sentences.
Why did 'Generative AI', which generates images and sentences, develop suddenly? -GIGAZINE
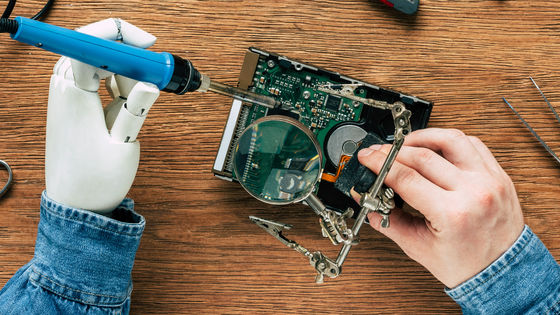
Originally, the data used for LLM and other training was created by humans in the past without the help of artificial intelligence, such as books, internet articles, photographs and illustrations. However, with the development of generative AI, there are concerns that the number of people who create content with AI and publish it on the Internet has increased, affecting the training data that is the basis of learning. At the end of May 2023, a group of British and Canadian researchers published a paper titled ``The Curse of Recursion'' in the open access journal arXiv . The paper states, ``The future of generative AI technology reveals alarming facts.''
Ilya Shmaylov, one of the paper's lead authors, said, 'We looked at the probability distributions of the text-to-text generative AI model and the image-to-image generative AI model and found that conditions were almost ideal for long-term learning. Even so, the process of 'data dispersion' is inevitable: over time, the errors in the generated data accumulate, and eventually AI learns from the generated data. will further misperceive reality.” According to Shumailov, a learning model can quickly forget the original data it was originally trained on, saying, ``We were surprised to observe how quickly the model collapsed. 'I'm talking.
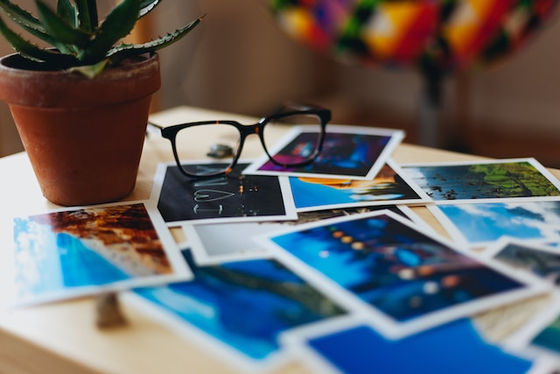
Also, one of the authors of the paper, Ross Anderson, a professor of security engineering at the University of Cambridge and the University of Edinburgh , talks about the research on his blog . Anderson describes the recursive and inaccurate state of generative AI's learning models, saying, ``Just as we littered the oceans with plastic trash and filled the atmosphere with carbon dioxide, what should we do with the internet? We are trying to fill it with information that doesn't exist. LLM is like fire, it's a useful tool, but it pollutes the environment.'
Anderson also said that as more content on the internet is generated using AI, it will become more difficult to scrape the web and train new models, resulting in companies and humans who already have enough training data. It points out that companies that can manage the content generated by will have a unilateral advantage. In fact, the Internet Archive blog reveals that an AI startup has made a large-scale access request to the Internet Archive in search of clean training data.
Shumailov explains that the mechanism by which AI content causes learning data to collapse is due to 'data bias'. According to Shumaylov, while human-generated raw data is a fairer representation of the world, generative AI models tend to over-prioritize popular data and misinterpret less popular data. , It is said that there are many things to express by mistake.
For example, when trained on 100 pictures of cats, 90 with yellow fur and 10 with blue fur, the model learns that yellow cats are more common. In addition to 'blue cats are expressed yellowish', 'green cats' may be output when new data is output. When the training cycle to further learn the 'yellowish blue cat' and 'green cat' generated by AI, the blue cat gradually increases the yellow color, and finally all the cats turn yellow. To go. In this way, the research group expresses 'data collapse' as distortion occurs in the progress process and the eventual loss of the characteristics of the minority data.
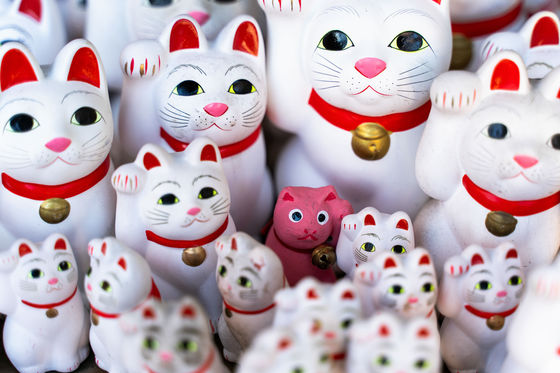
Furthermore, we found that even if we trained the model to avoid many iterations of the training cycle to avoid this, model collapse still occurred as the model began to invent false responses to avoid frequent iterations of the data. I'm here. As a countermeasure to model collapse, the paper states, 'Keep an exclusive, nominally human-generated, high-grade copy of the original dataset, and periodically retrain or completely refresh it. The idea is to avoid contamination by AI-generated data and to introduce a new, clean dataset generated by humans for training.
Shumailov points out that in order to prevent data collapse, it is important to ensure the amount of learning, accurately depict the features, and set the minority group in the dataset so that it is fairly represented. doing. Shumailov told VentureBeat, ``If you include 10% human-generated data in training, even if you use AI content recursively, model collapse will not occur so quickly. No, but collapse will still occur.'
Related Posts:
in Software, Web Service, Posted by log1e_dh