Large-scale language models such as GPT-4 and PaLM may suddenly bloom unexpected abilities as the scale grows
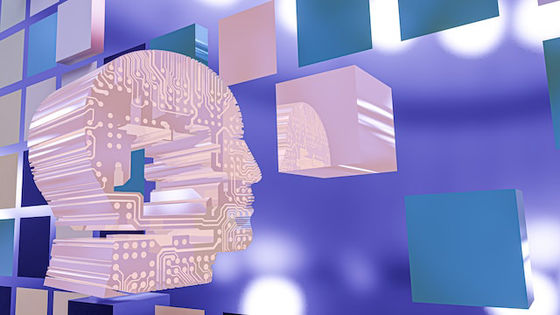
When AI develops unexpected abilities, it is called 'emergent.' In the world of biology, 'emergence' means self-organization and collective action in which a large number of objects function as one. It means that you will be able to complete the tasks you used to do. The emergence of large-scale language models has become a hot topic among recent experts who study large-scale language models.
Characterizing Emergent Phenomena in Large Language Models – Google AI Blog
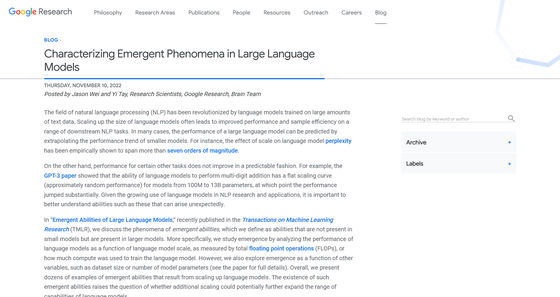
The Unpredictable Abilities Emerging From Large AI Models | Quanta Magazine
https://www.quantamagazine.org/the-unpredictable-abilities-emerging-from-large-ai-models-20230316/
It is said that the larger the scale of the large-scale language model, the more computational resources are required, but the more complex inferences can be made, and the accuracy of sentence generation will increase. Until now, it was thought that increasing the scale would improve known task performance, but not only that, but it became clear that ``it can also handle tasks that were previously thought to be impossible'' I'm here. However, AI researchers and developers still do not know why this emergence of AI is occurring.
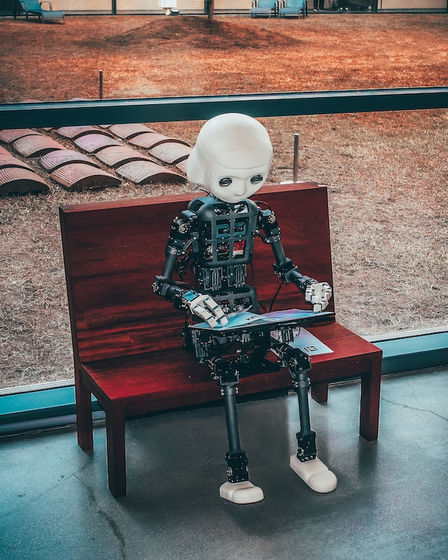
In 2017, Google researchers introduced a new architecture to the field of natural language processing called Transformer. Previously introduced
With the introduction of Transformer, we were able to increase the complexity and scale of the language model by increasing the number of parameters in the model. The more parameters the model has, the more accurately it is possible to connect words and create natural sentences with accuracy close to that of humans.
The number of parameters in large-scale language models is steadily increasing as daily research and development progresses, with Google PaLM having 540 billion parameters and OpenAI's GPT-4 reaching 100 trillion. However, a large-scale language model that boasts such a huge number of parameters can not only 'generate more accurate sentences quickly', but also 'be able to do tasks that were impossible before'. It is said that it has become.
Jonas Degrave, an engineer at AI company DeepMind, wrote on his blog that a simple program that builds a Linux virtual machine using ChatGPT, an interactive AI, and calculates the first 10 prime numbers on it. is reported to have been successfully executed. ChatGPT is originally an AI that only generates sentences in a conversational format, and it can be said that the fact that it was able to perform the task of emulating a computer is exactly the event that can be said to be the `` emergence of ChatGPT ''.
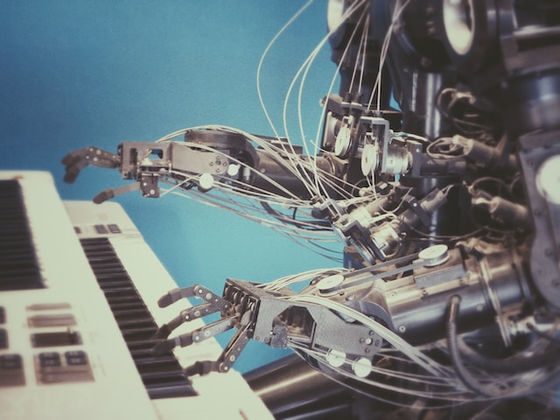
In 2020, Google Research researcher Ethan Dyer and others predicted that large-scale language models would bring about major changes, and started a project called 'Beyond the Imitation Game Benchmark.' This project was to give various large-scale language models 204 types of challenges and check how they were cleared.
For example, one of the assignments was ''???? ???? ???? ????' What movie is this?' In order to clear this task, it is necessary to decipher the meaning of the pictograms and then combine them with the contents of countless movies to make inferences. The simplest model answered, 'This movie is a movie about a human being, a human being,' but the most complicated model seems to have hit 'Finding Nemo' in one shot.
In addition, a relatively small model with a few million parameters could not correctly clear the problem of 3-digit addition or 2-digit multiplication, but a large model with tens of billions of parameters showed a sharp increase in response accuracy. He said he did. The phenomenon in which the accuracy of answers increases sharply as the number of parameters in a large-scale language model increases can be seen in other complex problems such as deciphering the International Phonetic Alphabet, deciphering sentences that combine Hindi and English, and translating Swahili proverbs into English. It seems that it was also seen in the task.
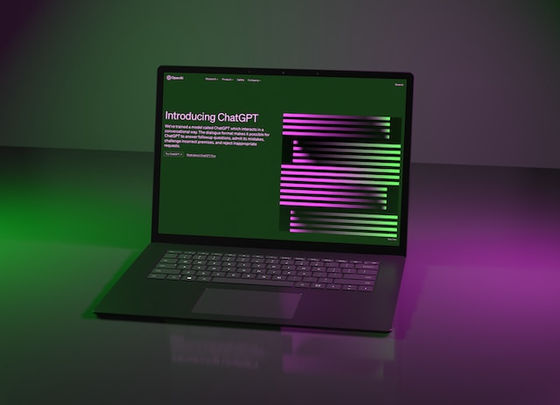
Dyer et al. argue that model complexity is not the only cause of emergence. This is because if the data quality is high, emergence can be observed even from a small model with a small number of parameters. In addition, the query expression method also affected the response accuracy of the model.
However, the unpredictability of AI also has the potential to produce harmful content. A study by AI company Anthropic reports that social prejudice appears as the number of model parameters increases. However, when the model was instructed not to include stereotypes or social prejudices, the model's predictions and responses were unbiased. The research team sees this as suggesting that emergent properties may be working to reduce model bias. Anthropic's research team refers to an attempt to install a 'moral self-correction mode' in a large-scale language model from this research result.
“We study how people actually use large language models,” said Deep Ganguly, a computer scientist at Anthropic. We spend a lot of time chatting with large language models.”
Related Posts:
in Software, Posted by log1i_yk