It turns out that the algorithm of machine learning is also implemented in the brain by dopamine nerves
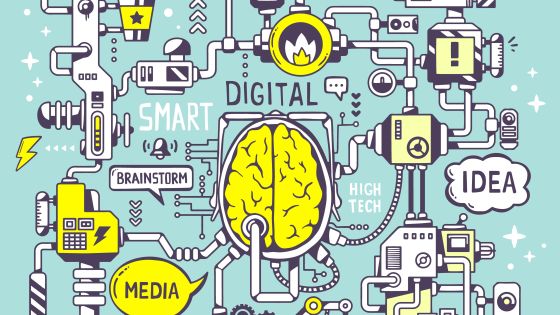
Scientists have long been studying the brain and nervous structures of living organisms, such as
A distributional code for value in dopamine-based reinforcement learning | Nature
https://www.nature.com/articles/s41586-019-1924-6
Dopamine and temporal difference learning: A fruitful relationship between neuroscience and AI | DeepMind
https://deepmind.com/blog/article/Dopamine-and-temporal-difference-learning-A-fruitful-relationship-between-neuroscience-and-AI
Reinforcement learning is the most classical idea of linking neuroscience and artificial intelligence, and even in the 1980s, attempts were made to develop artificial intelligence that could judge itself and take complex actions using rewards and punishments. The breakthrough in such reinforcement learning is called TD learning (temporal difference learning) . Whereas artificial intelligence was trained from the difference between the reward prediction obtained by the conventional learning method and the reward result actually received, TD learning is a method of learning from the difference between temporally continuous predictions is.
In contrast, it has been found that the brains of living organisms also perform TD learning. In the mid-1990s, it was discovered that the organism's dopamine nerves fired when there was an error in reward prediction. It has been shown that the brain learns by sending prediction error information into the brain using dopamine as a signal. The brain's TD learning has been verified by thousands of experiments and has become known as the most successful quantitative theory in neuroscience.
In recent years, in the field of artificial intelligence, research on reinforcement learning by deep learning using neural networks has been advanced, but a method that has greatly contributed to the development of reinforcement learning is a method called ' distributive reinforcement learning '. The amount of rewards that result from a particular action is difficult to quantify completely and is random. For example, in a situation where a computer-controlled avatar jumps a cliff, the reward is represented as a probability distribution with the option of jumping or not jumping the cliff. However, conventional TD learning was trying to predict the expected value of the reward, so it was not possible to incorporate a peak or bias in the probability distribution. To solve such problems, distribution reinforcement learning predicts the entire probability distribution.
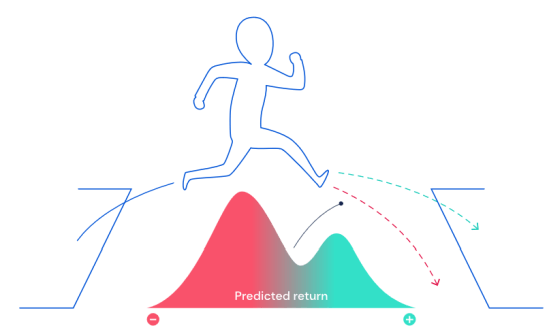
In distributed TD learning that combines distribution reinforcement learning and TD learning, different sets of
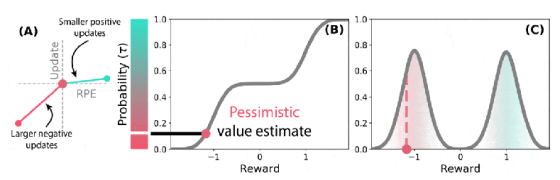
Since the distributed TD algorithm is known to be very compatible with artificial intelligence neural networks, it is inferred that the distributed TD algorithm is used in the brain of living organisms, DeepMind and Harvard University lab Collaborated on research. Previous studies have shown that dopamine cells show error in reward prediction due to changes in firing rate, so dopamine cell activity is standard when mice receive unexpected rewards in learned tasks. We are evaluating whether it is closer to the TD algorithm or the distributed TD algorithm, and we are experimenting with how we can observe the diversity of different reward predictions in the brain.
The graph is color-coded according to the amount of water given, the dopamine cells are divided on the vertical axis, and the error of the reward prediction is shown on the horizontal axis. If it is close to the standard TD algorithm, the error of reward prediction should be the same for each dopamine cell as shown in the graph on the left. Conversely, if you are closer to the distribution type TD algorithm, the error of reward prediction should be different for each dopamine cell as shown in the graph on the right.
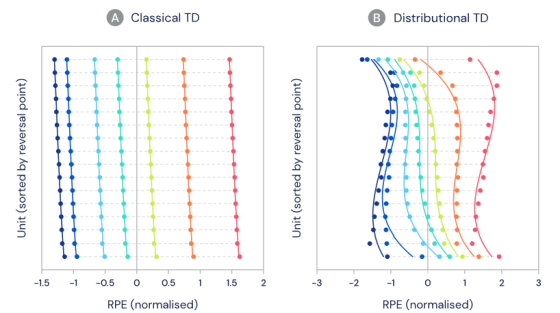
The experiment showed significant differences between the different dopamine cells that could not be explained by noise. The distribution of the firing rate of dopamine cells is similar to the distribution type TD algorithm.
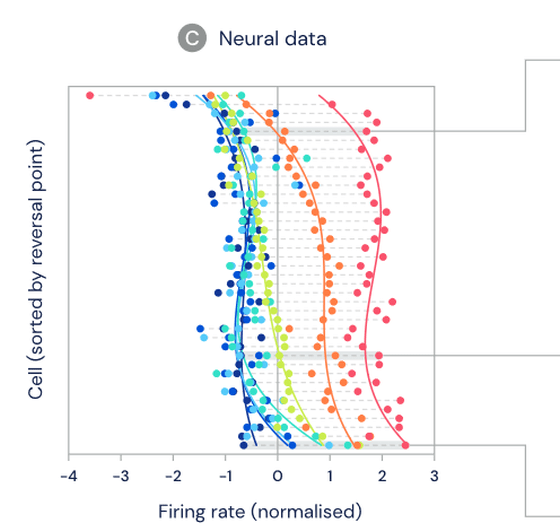
If the dopamine cells respond according to the TD algorithm from the distribution of rewards, the distribution of rewards should be able to be calculated from the firing rate of dopamine cells. Decoding the TD algorithm and calculating it, we found that the result was very close to the actual reward distribution. The gray part in the figure is the actual distribution of rewards, and the dark blue is the average value of the calculation results.
The study showed that the dopamine cells in the brain were regulated at different levels, each being pessimistic and optimistic, and that they were harmonized as a whole. In artificial intelligence reinforcement learning, this adjustment of diversity is a factor that increases the learning speed in neural networks, and the brain may have secured diversity for the same reason.
The discovery is interesting for both artificial intelligence and neuroscience. The fact that the machine learning algorithm was also in the brain suggests that machine learning research is on the right path, and for neuroscience, if the brain is optimistic dopamine nerve and pessimistic dopamine When responding selectively to nerves, mental health and motivation, such as whether depression may occur, and how dopamine cell diversity is related to other brain varieties. It is said to give new insights into understanding.
Related Posts:
in Science, Posted by darkhorse_log