Researchers at Osaka University announce research to generate images from brain activity data obtained by fMRI with image generation AI 'Stable Diffusion'
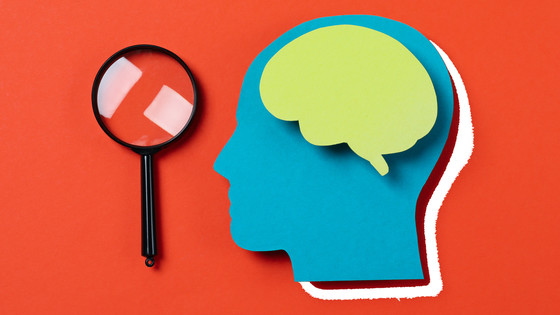
High-resolution image reconstruction with latent diffusion models from human brain activity | bioRxiv
https://doi.org/10.1101/2022.11.18.517004
Stable Diffusion with Brain Activity
https://sites.google.com/view/stablediffusion-with-brain/
High-resolution image reconstruction with latent diffusion models from human brain activity | bioRxiv
https://doi.org/10.1101/2022.11.18.517004
When humans perceive or imagine something with their eyes or ears, their brain cells become more active. When brain cells become more active, blood flow to that area increases. fMRI is a device that can visualize active areas of the brain by capturing slight magnetic changes due to this increase in blood flow, and enables non-invasive measurements that do not require surgery.
Previous studies have attempted to recreate the visual experience of a person who has captured something visually from the functional activity of the brain of that person. The visual experience consists of 'image features' handled by the early visual cortex and 'textual semantic features' handled by the higher visual cortex. is used to create an image.
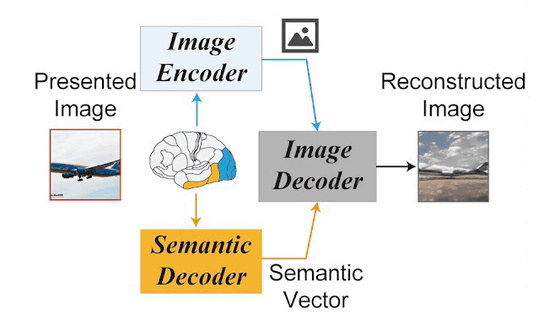
The mechanism of Stable Diffusion is summarized in the following article.
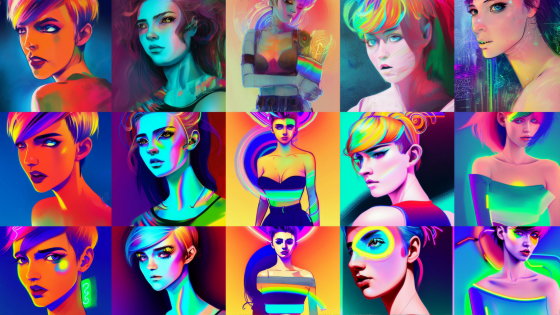
Below is a diagram showing the mechanism of Stable Diffusion. An image (X) is read by an image encoder (ε), converted to a latent representation (z), and converted to zT by a noise-adding diffusion process. We pass this zT through a UNET encoder to reverse the diffusion process, removing the noise. At this time, the input text is converted to a feature value (c) with CLIP (τ) of the text encoder. A new latent representation (z c ) is generated by adjusting the directionality of noise removal with this c, and a new image (X') is generated by reading this z c into the image decoder (D). increase.
And the research of Assistant Professor Takagi is below. A latent representation (z) is obtained from the activity (video features) of the early visual cortex measured by fMRI, and an image (X z ) is generated by loading it into an image decoder. A resized copy of this image is loaded into Stable Diffusion's image encoder and undergoes a diffusion process to generate a latent representation (z T ). In addition, the feature value (c) is obtained with a text encoder from the text expression obtained by the linear model from the activity of the higher visual cortex (sentence-like semantic features). A UNET encoder generates a latent representation (z c ) from z T and c, which is passed through a decoder to generate an image (X zc ). This X zc is the image reconstructed using Stable Diffusion from the image seen by the subject.
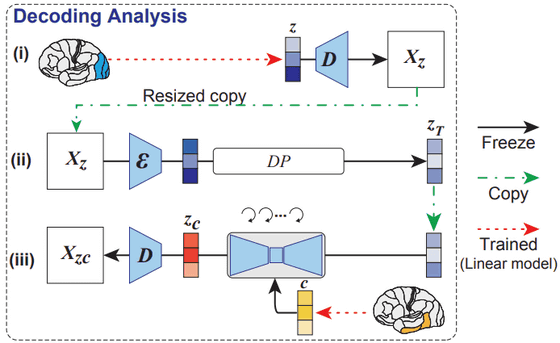
The image presented to the subject in the experiment is the image surrounded by the leftmost red frame. Z is the image generated from the subject's initial visual cortex, C is the image generated by the text encoder from brain activity in the higher visual cortex, and Zc is the final image generated by Stable Diffusion. Comparing the image in the red frame with Zc, you can see that the image seen by the subject can be reconstructed.
However, since the shape and structure of the brain differs from person to person, it is not possible to directly apply the model made by individuals to others. Below is an image generated through Stable Diffusion after showing the leftmost image to four subjects (subj01, subj02, subj05, subj07). There are some things that seem to be common, but you can see that different images are generated. Even so, several methods have been proposed to correct for individual differences, and Assistant Professor Takagi expects that it will be possible to apply the model across subjects with a certain degree of accuracy.
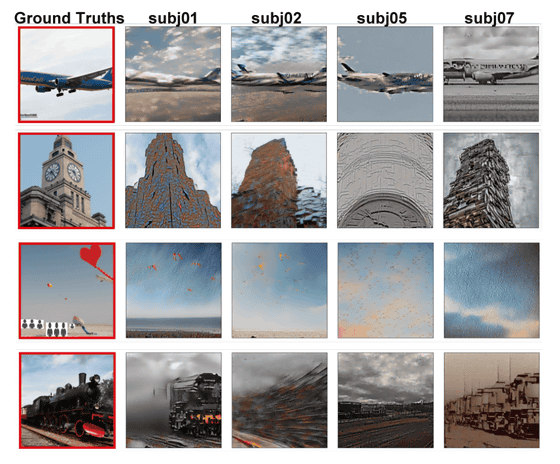
Furthermore, Assistant Professor Takagi and his colleagues are also attempting to quantitatively interpret each component of the latent diffusion model from the perspective of neuroscience by mapping specific components of the latent diffusion model to brain activity regions.
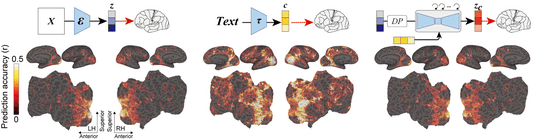
Previous research also aimed to generate images from fMRI data using machine learning, but training and fine-tuning were necessary. However, the method of Assistant Professor Takagi et al. only trains a linear model for creating latent representations from fMRI measurement results, and for Stable Diffusion, the version 1.4 model is used as is.
Assistant Professor Takagi's linear model training dataset used the ' Natural Scenes Dataset ', which summarizes the brain activity measurement data of subjects who have viewed up to 10,000 images multiple times.
According to Assistant Professor Takagi, 'It is known that there is a certain degree of homology between brain activity when experiencing (perceiving) something and brain activity when recollecting or dreaming of something. It is known that this property can be used to decipher the contents of recollections or dreams with a certain degree of accuracy,' suggesting the possibility of visualizing dreams as images. However, Assistant Professor Takagi says, ``(This research) examines the relationship between perceived content and brain activity, and is not mind reading.'' I'm pretty sure it's not technical.
Furthermore, Assistant Professor Takagi added, ``As a premise, it is difficult to imagine that technology that can sufficiently decode information in the brain will be put into practical use immediately. We have to spend hours in an fMRI scanner, and there is still room for improvement in the accuracy of our decoding models.”Decoding brain activity can raise serious ethical and privacy issues. We believe that the brain is extremely sensitive personal information, and we strongly believe that no form of brain activity analysis should be performed without informed consent.'
Related Posts: