What is the strategy taken by the distribution app Lyft to automate marketing?
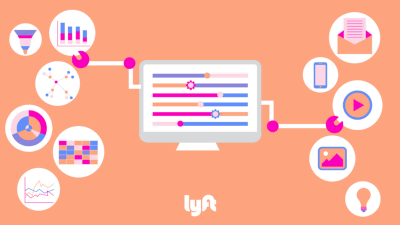
Building Lyft's Marketing Automation Platform-Lyft Engineering
https://eng.lyft.com/lyft-marketing-automation-b43b7b7537cc
Although Lyft's growth is due to “raising awareness in regional campaigns” and “ thinking multimodal methods”, it takes a lot of time to gain new customers in such campaigns. Because of that, Lyft has come to the idea of 'automating marketing'.
Acquisition of new customers usually involves multiple data-based departments working together to address issues such as scale, measureability and predictability. The new acquisition is where the consumer is aware of the product and the purchase funnel is the end of the purchase , and there are various channels leading up to here. Each channel is different in terms of partner, technology, strategy, etc, so one division of Lyft's strategy team needs to launch an advertising campaign in conjunction with the channel. For example, below is the driver's ad on the left, and the consumer ad on the right.
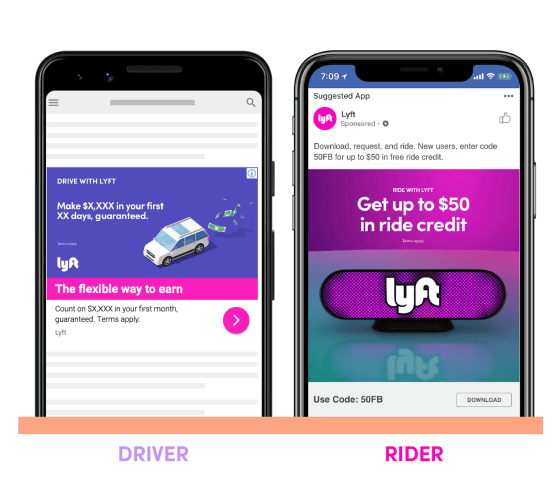
And at the same time, another strategic team is thinking about the campaign, focusing on the broader 'user experience'.
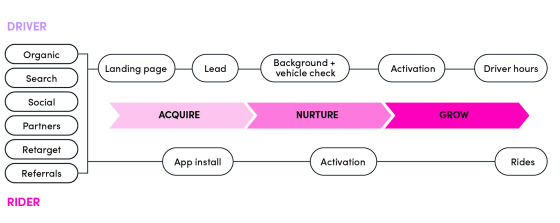
To get new customers on a large scale, you need to make various decisions every day. However, deciding the contents such as 'price making', 'budget', '
The requirements of the marketing automation platform that Lyft needed are:
1. To have the ability to predict the likelihood that new customers will use Lyft's products
2. Measurement mechanism for allocating marketing budgets across different internal & external channels
3. Ways to develop these budgets in thousands of advertising campaigns
And the problems in automation are as follows.
・ Update bids across thousands of search words
・ Turning off display ads with poor performance
・ Change customer introduction value by market
Identify high-value user segments
・ Share findings from different strategies of one campaign
As a way to solve these problems, Lyft created ' Symphony ', a system to achieve business objectives, forecast future user value, allocate budget, and create a campaign to attract new customers.
Symphony's architecture mainly consists of three components: Life Time Value (LTV) Forecasting, Budget Allocation, and Bidder.
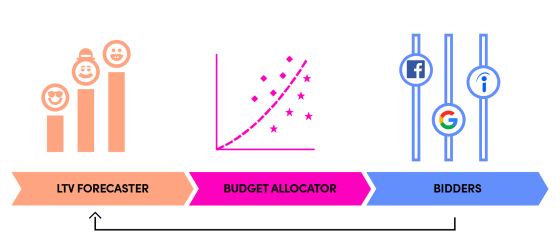
Details of the three components are as follows.
◆ LTV forecast
Life-time value is a calculation of how much profit a customer or customer brings in the period between the beginning and the end of trading with a particular company or brand. Understanding the value of users in a business is very important, and the component of LTV forecasting predicts the efficiency of customer acquisition channels based on the value of users flowing from various channels. And the budget is allocated based on the channel efficiency found here. LTV helps to set medium- and long-term strategic goals, so accurate forecasts are required.
At this time, it is difficult to predict the user's ownership rate, usage frequency, and transaction price at the initial stage of the user's life cycle, so past data are used for the first forecast. As the frequency with which users use the service increases, the predictions become more accurate.
The graph below shows that LTV increases as users use Lyft. The pink line shows the benchmark, which represents the LTV predicted from the user's
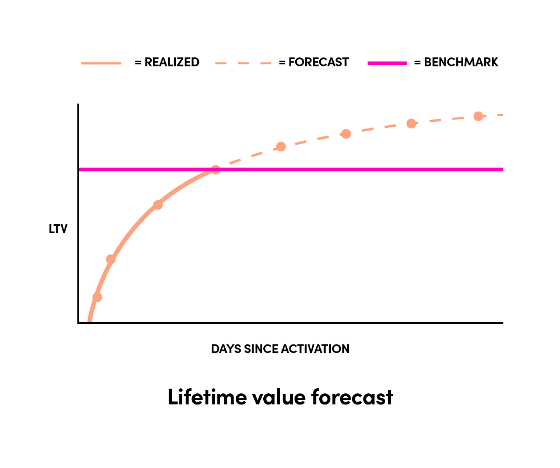
Budget allocation
Budget allocation is based on data gathered from LTV forecasts and marketing performance data. Here, the Markov chain Monte Carlo method is used, and values are calculated with some degree of randomness based on the curve “LTV = a * (spend) ^ b”. In the graph below, the vertical axis represents LTV, the horizontal axis represents actual expenditure, and the central curve represents 'LTV = a * (spend) ^ b', and the dark pink '95% of expenditure falls within this range' Is shown.
'It seems that such randomness is useless, but in the long run there is a gradual exploration.' Because Lyft is deployed worldwide, it seems that it is possible to find out the unexpected possibilities by giving it a width.
◆ Bidder
Bidders (bidders) make the final adjustments needed to launch the ad at the target price point. Bidder is divided into two parts, 'Tuner' and 'Actor'. First, the tuner develops capital based on levers such as keyword, title, and
Lyft has worked with various partners to display products to the right audience. Each channel seems to have different strategies depending on the feature.
This is an example of a strategy. 'Maximize clicks,' 'maximize conversion,' 'maximize earnings,' and 'maximize ad visibility.'
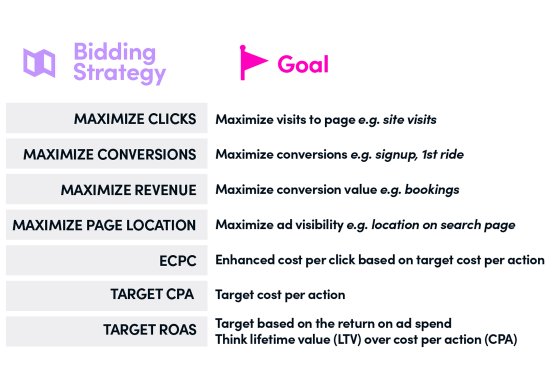
Since Lyft's marketing automation is done by machine learning, it is thought that it will be important how human feedback can be fed into the machine learning platform in the future. This kind of human participatory (human-in-the-loop) machine learning makes it possible for people to focus on problems that need to be concentrated by using the power of automation. By letting go of routine tasks such as bidding and budgeting, Lyft believes that marketing teams can spend more time with creatives and audiences.
Related Posts:
in Software, Web Service, Web Application, Posted by darkhorse_log