Apple is 'Do HomePod are using any technology to accurately recognize the user's voice?' The commentary
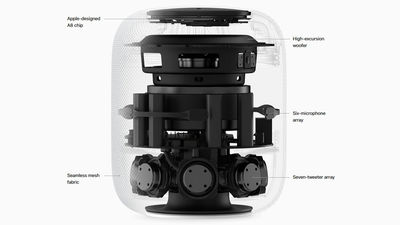
Apple's speech recognition assistant "Siri" is also installed in mobile terminals such as iPhone and iPad, and HomePod of smart speakers. The iPhone etc is relatively used near the mouth of the user "Hey Siri" is used, but since the HomePod of the smart speaker is fixedly used in one place, talk to the user from a distance far from the iPhone etc. There are more cases to be encountered, and there is a need to accurately recognize the command "Hey Siri" and the speaker's remarks from environmental sounds and others. What kind of method Apple uses is used to detect exactly "voice spoken to HomePod" from "TV out sound" and "voice not talking to HomePod" It is revealed on the Learning Journal .
Optimizing Siri on HomePod in Far-Field Settings - Apple
https://machinelearning.apple.com/2018/12/03/optimizing-siri-on-homepod-in-far-field-settings.html
Since Siri-equipped HomePod also has a smart home function as a speaker, "When playing music with loud volume", "When users are far away from HomePod", and "TV and Even when other sound sources such as household electric appliances are active ", it is necessary to be able to recognize the voice of the user such as" Hey Siri "correctly.
So, Apple's audio, software and engineering team and Siri team worked together to integrate deep learning and online learning algorithms, and built a system that can recognize spoken words far away from multiple microphone signals. The multichannel signal processing system built by the development team is mainly composed of "mask based multichannel filtering function to remove echo and background noise using deep learning" and "simultaneous sound source and trigger phrase using unsupervised learning The function of separating the stream selection of the base and excluding interfering sound sources "seems to be combined.
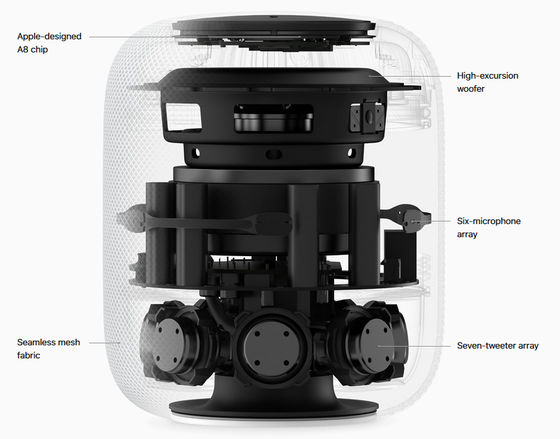
The multi-channel signal processing system uses six microphones of HomePod and A8 chip, and performs multi-channel signal processing continuously. As Homepod operates in the minimum power state to conserve energy, multi-channel signal processing is also performed, so HomePod responds appropriately to "constantly varying noise conditions" and "moving speaker" It will be possible.
Although it seems that multi-microphone processing is being carried out even in state-of-the-art systems other than HomePod, in most cases "echo cancellation", "noise suppression", etc. are only being done. Although a speech enhancement system is used to suppress echo and noise, in this system it is necessary to learn characteristics of unnecessary speech signals using "supervised learning" or "unsupervised learning".
In recent years, the appearance of deep learning seems to greatly improve the performance of this speech enhancement system. On a system with a high level, it is said that "a method to learn voice existence probability by using a deep neural network driving a multi-channel noise suppression filter" is adopted. However, these systems are generally built on the assumption that a complete voice utterance is available at runtime and the system performs batch processing to utilize all voice samples during voice commands It seems to be stuff. Therefore, it has the disadvantage that "waiting time gets longer" and furthermore it hinders the voice reinforcement function of the home listening mode of the home assistant device. Also, because it is impossible to predict speech conditions, it seems that it was one factor that HomePod deemed inappropriate to be able to use start and end points of voice commands in advance.
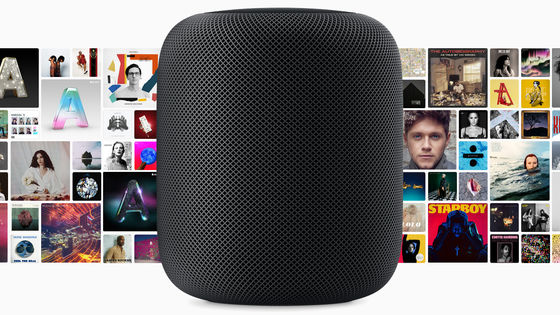
When recognizing speech from a distant place, it becomes an obstacle when another active speaker like people or TV is in the same room as the target speaker. If the voice commands such as "Hey Siri" are not separated from the interfering audio elements, the accuracy of points such as voice trigger detection, voice decoding and end point conversion may be greatly reduced.
Traditionally, researchers seem to have tried separating sound sources using independent component analysis and clustering, unsupervised learning, deep learning, etc. However, in long distance voice command drive interfaces such as smart speakers, these The effectiveness of the process is very limited. For this reason, a multichannel signal processing system has been developed so that only target streams containing voice commands can be selected and decoded with low delay. As a result, Apple's developed multichannel signal processing system is able to separate competing audio signals online.
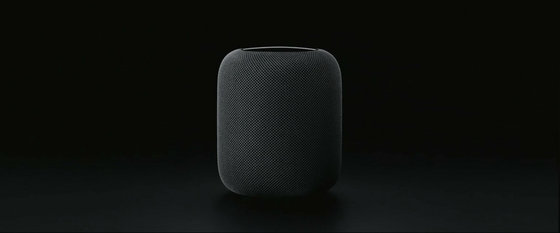
The developed multichannel signal processing system is "an environment where music and podcasts are played at various sound volumes" and "environment where continuous background noise including rain noise is generated" "vacuum cleaner, hair dryer, electronic In an environment where household appliances such as ranges emit directional noise, "environment with interference from external conflicting sources of speech" is tested and its accuracy is verified.
The following graph shows the false detection rate of "Hey Siri" for each of the four acoustic conditions "Reverberation", "Echo", "Noise", and "Competing Talker" . "No processing" for blue, "When only basic digital signal processing is performed" for "green", "when processing green processing + mask based noise reduction processing" for orange, "orange processing + stream selection for yellow" It shows the false detection rate of "When I went". It is understood that the false detection rate of "Hey Siri" in a complicated acoustic environment decreases with each processing.
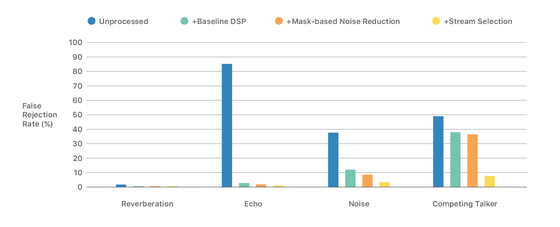
The following graph shows the word error detection rate (WER) when the same processing is performed under the same acoustic condition as the above graph. The blue color of the graph shows a case where the speech is erroneously detected, and the green color shows the erroneous detection caused by missed listening.
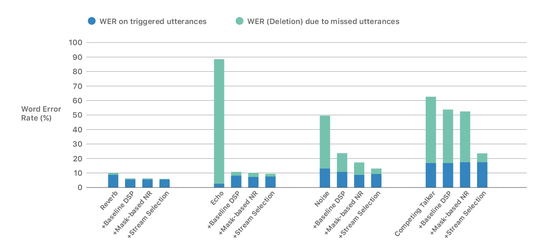
From the two results, it is shown that even if there are loud sounds, echoes, noise, competing speakers, etc., the user can easily interact with HomePod.
Related Posts: