Introducing 'Sakuga-42M,' an animation-focused dataset that can be used to train AI models
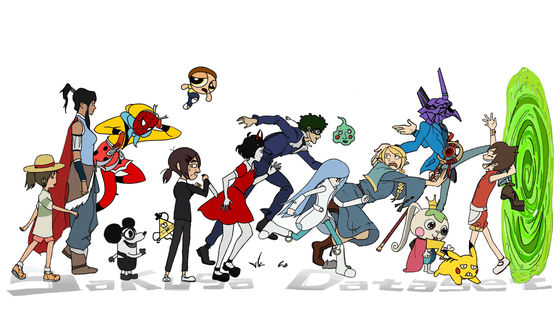
There are many tools that use AI models to generate videos, but there are very few that can generate two-dimensional animations rather than photorealistic videos. In order to overcome this situation, ' Sakuga-42M ' was created as a large-scale dataset specialized for animation.
[2405.07425] Sakuga-42M Dataset: Scaling Up Cartoon Research
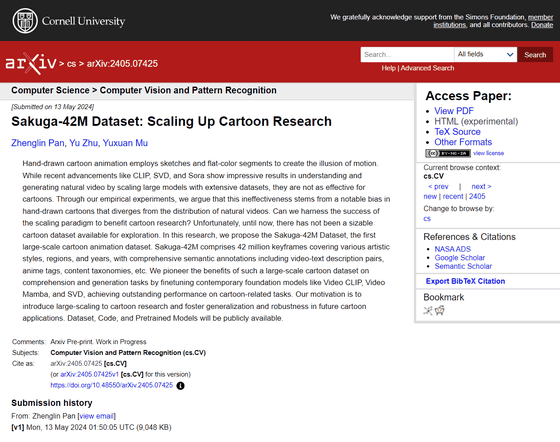
Sakuga-42M Dataset: Scaling Up Cartoon Research
https://arxiv.org/html/2405.07425v1
With the advent of video generation AI such as Stable Video Diffusion (SVD) and Sora , efforts to 'train AI models using large datasets to understand and generate natural videos' have made remarkable progress. However, this only applies to 'real-life-like videos', and the research team of Zhenglin Pan and others at the University of Alberta points out that there has been little progress in the animation field. This is because the datasets used to train AI models do not contain large-scale animation-only data.
Therefore, 'Sakuga-42M' was created as a large-scale animation-only dataset. Sakuga-42M contains animation videos of various styles, regions, and eras, with a total of 42 million keyframes. The video data not only includes text descriptions, but also tags for content classification.
Below is an example of the content classification. It is categorized by animation type, such as rough sketches, Western style, Asian style, cell look, illustration style, etc.
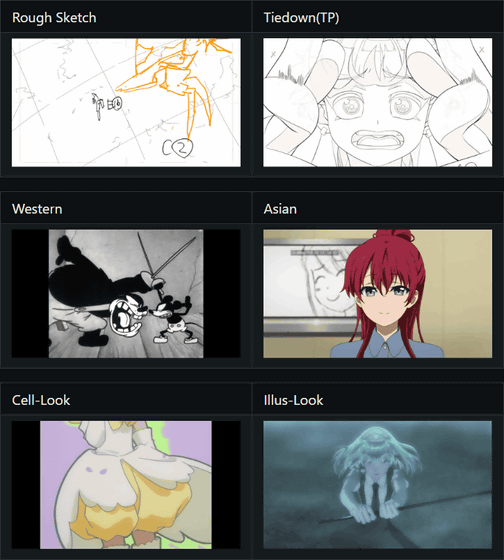
In addition, the video also includes text to explain the content, such as, 'Blonde, red-haired, and brown-haired girls are wearing idol costumes and dancing in a line on stage.'
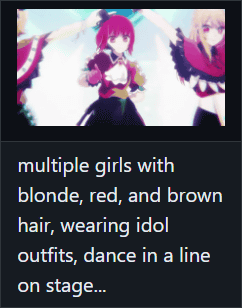
The research team divided the long animated videos collected as data for Sakuga-42M into short videos using boundary detection, video segmentation, and keyframe detection techniques. Then, by automatically outputting the explanations of the short videos using a large-scale language model (LLM), it seems that the amount of information available for training the AI model is increased.
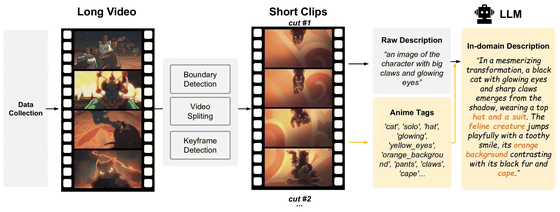
The following is a diagram summarizing the breakdown of data contained in Sakuga-42M. 'CLIP DURATIONS' (length of video) is '23.02%' for short (12 or less keyframes), '27.51%' for medium (12 to 24 keyframes), '27.00%' for long (24 to 48 keyframes), '15.99%' for extra (48 to 96 keyframes), and '6.48%' for infinite (96 or more keyframes). 'DESCRIPTION LENGTH' (length of description) is '9.32%' for short (less than 20 words), '29.27%' for medium (20 to 40 words), '54.20%' for long (40 to 60 words), and '7.22%' for infinite (60 or more words). In addition, data on 'ASTHETIC SCORE', 'DYNAMIC SCORE', and 'TAXONOMY' are also included. According to the research team, Sakuga-42M is a dataset that exceeds the combined size of all existing animation-related datasets.
Pan et al. explained their motivation for creating Sakuga-42M was to 'introduce large-scale to animation research and promote generalization and robustness in future animation applications.' They further stated, 'We hope that this will somehow alleviate the data shortage problem that has long plagued researchers in this field, leading to the introduction of large-scale models and approaches that will lead to the emergence of more robust and portable applications, and ultimately to the creation of animators.'
Sakuga-42M is available on GitHub under the Creative Commons 4.0 Attribution-NonCommercial-ShareAlike License, with a disclaimer that it may only be used for academic research purposes, and that the copyright of images and videos included in the dataset belongs to their respective authors.
GitHub - zhenglinpan/SakugaDataset: Official Repository for Sakuga-42M Dataset
https://github.com/zhenglinpan/SakugaDataset
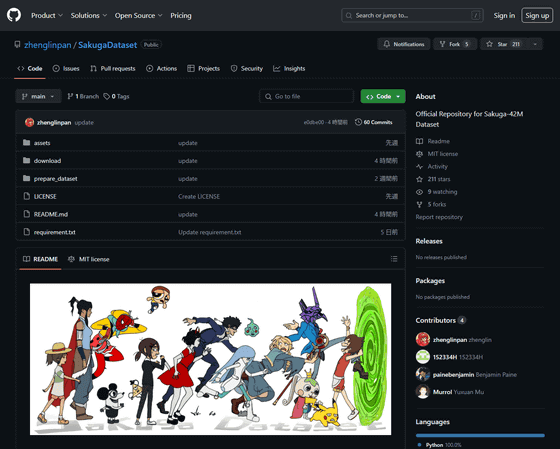
Related Posts:
in Software, Posted by logu_ii