Massachusetts Institute of Technology develops a method to speed up image generation by AI by 30 times
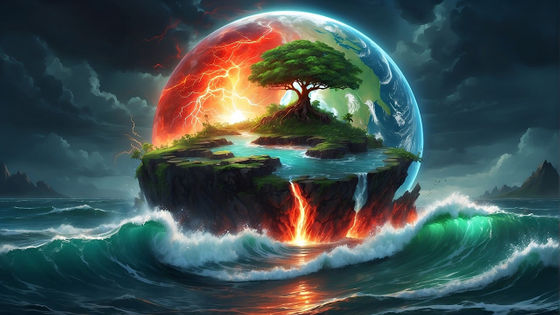
The Massachusetts Institute of Technology (MIT) in the United States has developed a technology that simplifies the diffusion model of popular image generation AI such as DALL-E 3 and Stable Diffusion and accelerates the generation speed by up to 30 times while maintaining the quality of the generated images. Published by the research team.
[2311.18828] One-step Diffusion with Distribution Matching Distillation
AI generates high-quality images 30 times faster in a single step | MIT News | Massachusetts Institute of Technology
https://news.mit.edu/2024/ai-generates-high-quality-images-30-times-faster-single-step-0321
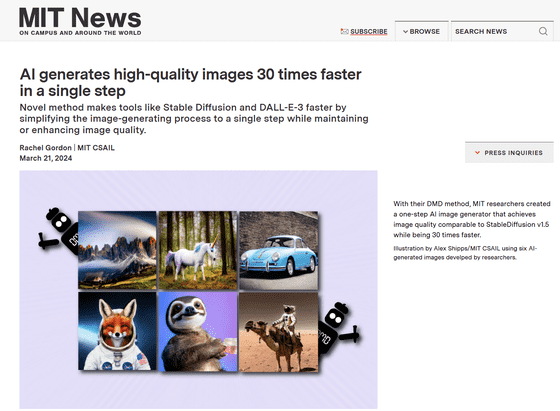
MIT scientists have just figured out how to make the most popular AI image generators 30 times faster | Live Science
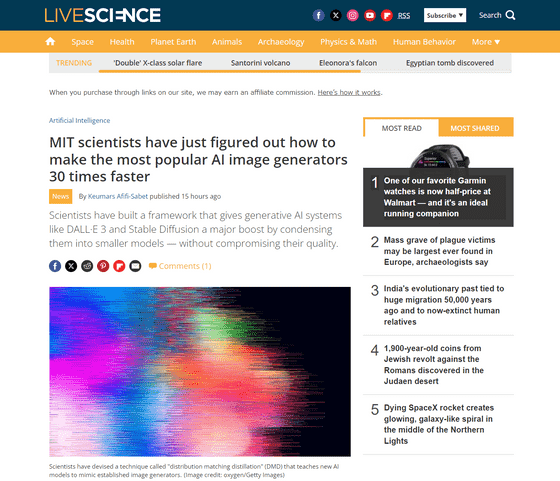
Diffusion models used in many image generation AIs can accurately generate images from text prompts by using images with captions and metadata that describe the images as training data. has been trained.
In this process, the diffusion model first converts a random image into noise, and then performs a noise removal process called ``de-diffusion'' for up to 100 steps, making it possible to generate a clear image.
The MIT research team recently announced a method called ``distribution matching distillation (DMD)'' that succeeded in significantly shortening the time it takes to generate images by reducing the ``back-diffusion'' step to one step.
In one test using Stable Diffusion v1.5, image generation time was reduced by a factor of 30 from 2590 ms to 90 ms.
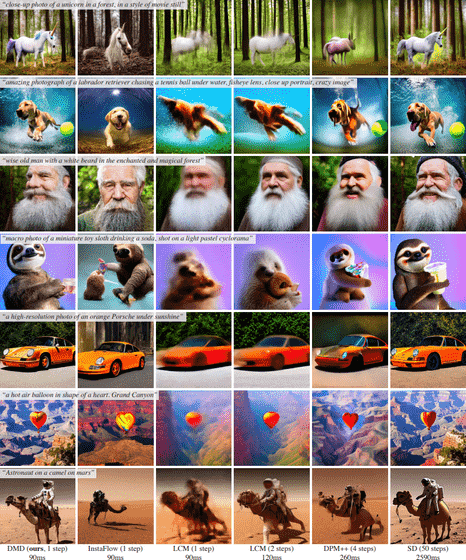
MIT's Tianwei Yin said, ``Our research combines the principles of
DMD has two elements that are important in reducing the number of iterations required to generate an image. The first is called 'regression loss,' which speeds up the AI by organizing images based on similarity during training. The second is 'distribution matching loss,' which corresponds to the probability of generating a particular image with the probability in the real world. By combining these technologies, the possibility of strange parts appearing in images generated by the new AI model is minimized.
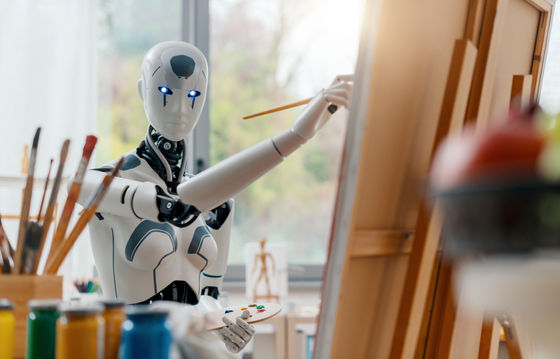
This new approach is expected to greatly benefit the AI industry, which requires fast and efficient image generation, as it dramatically reduces the computational power required to generate images, leading to faster content generation.
MIT's Fredo Durand said, ``Ever since the diffusion model was invented, there has been a holy grail search for a way to reduce the number of iterations. 'We are very excited about the dramatic reduction in computational costs and acceleration of the generation process.'
Related Posts:
in Software, Posted by log1l_ks