Google improves the performance of 'AI model that converts low resolution images of Gabigabi to high resolution images', to a level that humans can not distinguish
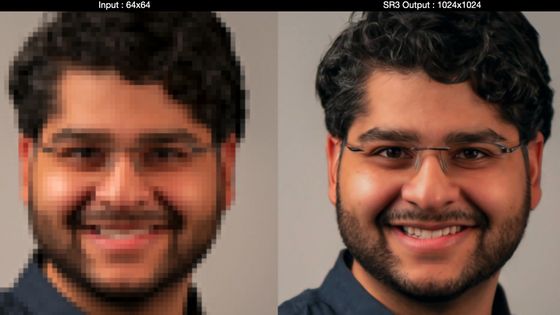
Google's AI research team
Google AI Blog: High Fidelity Image Generation Using Diffusion Models
https://ai.googleblog.com/2021/07/high-fidelity-image-generation-using.html
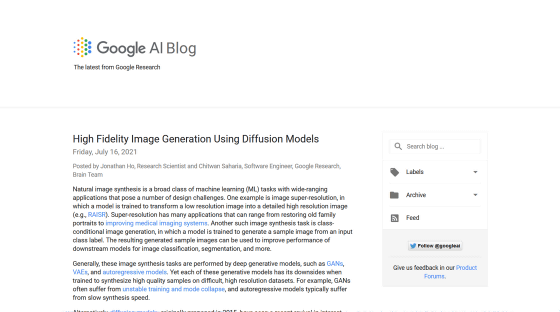
Enhance! Google researchers detail new method for upscaling low-resolution images with impressive results: Digital Photography Review
https://www.dpreview.com/news/0501469519/google-researchers-detail-new-method-upscaling-low-resolution-images-with-impressive-results
Google's New AI Photo Upscaling Tech is Jaw-Dropping | PetaPixel
https://petapixel.com/2021/08/30/googles-new-ai-photo-upscaling-tech-is-jaw-dropping/
In general, the task of restoring high-resolution images from low-resolution images uses generative models such as hostile generation networks (GANs) , variational auto-encoders ( VAEs), and autoregressive models. However, GANs have some drawbacks, such as mode collapse in which many of the generated images are duplicated, and autoregressive models have problems such as slow synthesis speed. And that.
On the other hand, the generative model called 'diffusion model' announced by Google AI in 2015 seems to have been reviewed in recent years due to its stability in training and the high quality of generated images and sounds. And newly, Google AI will improve the image composition quality of diffusion models by using two new diffusion model approaches, 'Super-Resolution via Repeated Refinements (SR3) ' and ' Cascaded Diffusion Models (CDM)'. It states that it was successful.
First, SR3 gradually adds Gaussian noise to the low-resolution image and damages it until it becomes a 'pure noise image'. After that, the trained neural network reverses the image corruption process to remove noise and generate a high-resolution image that exceeds the original resolution.
The left is a low-resolution image of 64 x 64 pixels, which is the input data, and the right is a 'pure noise image' by adding Gaussian noise to the low-resolution image.
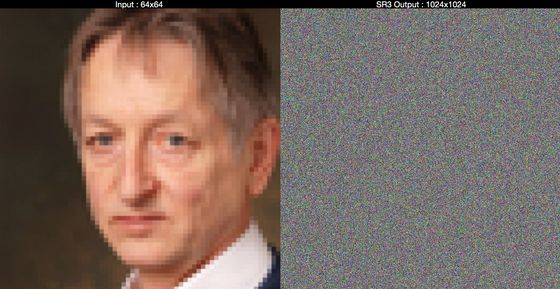
Gradually remove noise from the 'pure noise image' ...
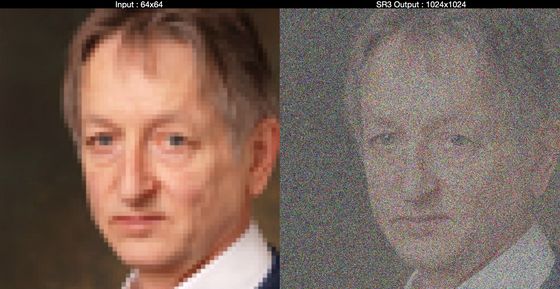
Finally, a much finer facial photo than the original image was generated.
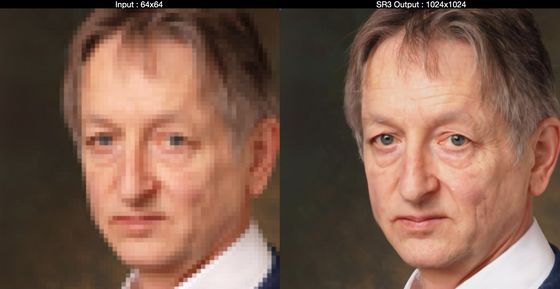
It seems that it works in the same way for female images.
This is the result of the research team actually showing a human subject 'the original image' and 'an image generated from a low-resolution image by various methods' and having them determine which is the original image. The closer the subject's error rate (confusion rate) is to 50%, the more difficult it is to tell whether the AI-generated image or the original image is genuine.
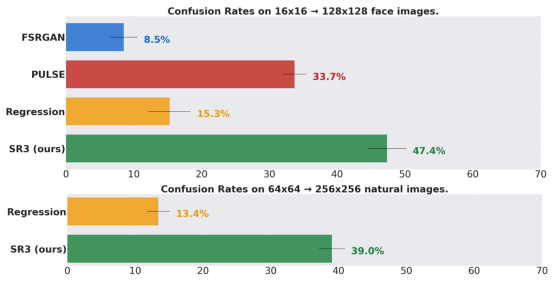
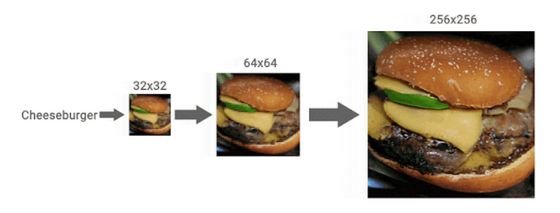
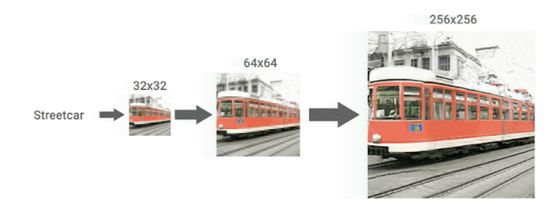
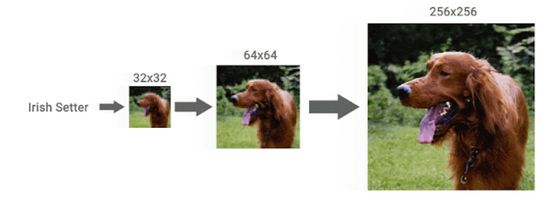
Related Posts: