OpenAI announces 'sCM', an approach that can complete the diffusion process used for generative AI in just two super-fast steps
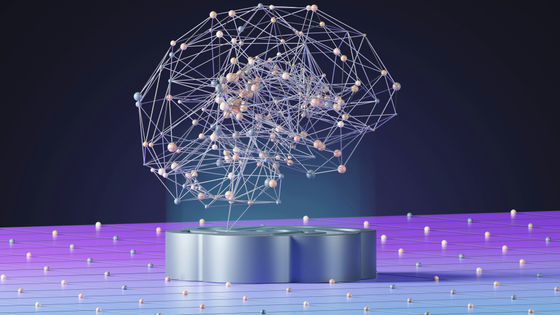
Generative AI models such as the image generation AI 'Stable Diffusion' use an approach called the 'diffusion model.' OpenAI has devised an alternative to this diffusion model, an approach called ' sCM ' that simplifies and speeds up the diffusion process. While it would normally take dozens to hundreds of sampling steps, sCM requires just two steps.
[2410.11081] Simplifying, Stabilizing and Scaling Continuous-Time Consistency Models
Simplifying, stabilizing, and scaling continuous-time consistency models | OpenAI
https://openai.com/index/simplifying-stabilizing-and-scaling-continuous-time-consistency-models/
The diffusion model is a model that learns by repeatedly adding noise to data and removing the noise, and the number of times the noise is removed is called the sampling step. Generally, increasing the sampling step moderately improves the quality of the generated results, but at the same time increases the processing time. For this reason, AI researchers are developing 'technology that maintains high quality of generated results even with fewer sampling steps,' but OpenAI points out that 'existing approaches often involve constraints such as high computational costs, complex training, and reduced sample quality.'
OpenAI has developed previous research on consistency models , known as a fast alternative to traditional diffusion models, to simplify and improve stability. As a result, unlike diffusion models that gradually generate samples through multiple noise removal steps, OpenAI has completed a new approach to consistency models called sCM, which aims to directly convert noise into noise-free samples in one step.
sCM makes it possible to extend the learning of continuous time consistency models to 1.5 billion parameters on ImageNet with a resolution of 512 x 512. For example, the largest sCM model with 1.5 billion parameters generates one sample in just 0.11 seconds on a single NVIDIA A100 GPU without inference optimization. OpenAI explains that further speedups can be easily achieved by optimizing the system, expanding the possibility of real-time generation in various domains such as images, audio, and video.
The Fréchet inception distance, which compares real images with generated images and evaluates the quality of images created by generative AI, has been shown to generate samples of quality comparable to conventional methods while using less than 10% of the effective sampling computational effort.
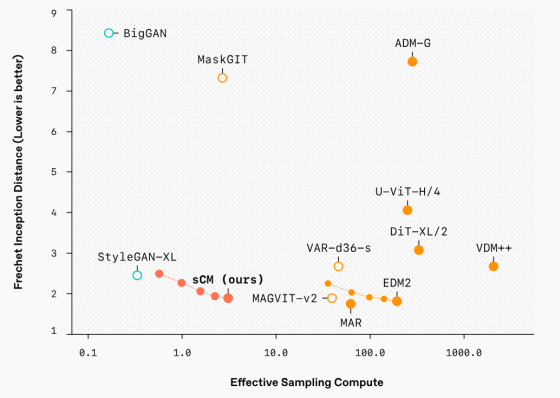
A sample of the image generated in the two steps is as follows.
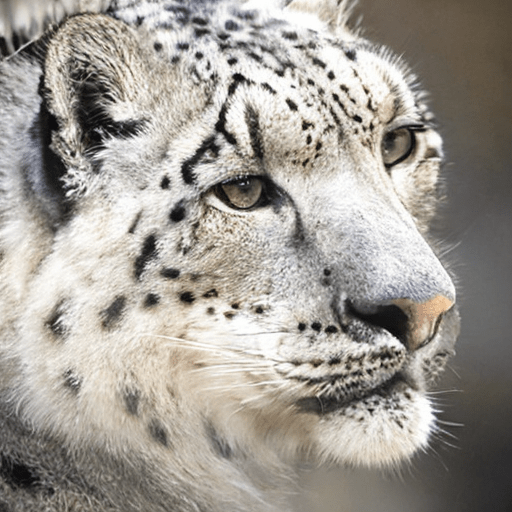
'We will continue to work toward developing better generative models with improved inference speed and sample quality. We believe these advances will open up new possibilities for real-time, high-quality generative AI in a wide range of domains,' OpenAI said.
Related Posts:
in Software, Posted by log1p_kr