What is the difference between AI and human “hallucinations”?
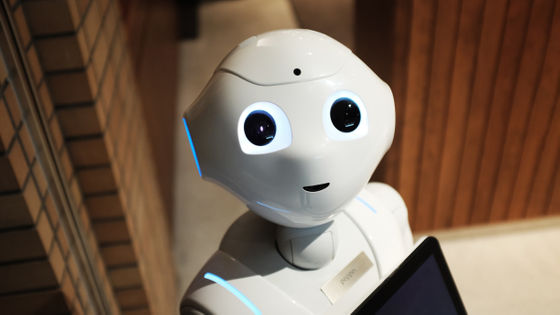
With the spread of AI, the problem of hallucinations has become common, with ``hallucination'', in which AI generates false information, being
Both humans and AI hallucinate — but not in the same way
https://theconversation.com/both-humans-and-ai-hallucinate-but-not-in-the-same-way-205754
◆What exactly are human hallucinations?
Hallucinations can mean a variety of things, including hallucinations and visual hallucinations, but if hallucinations are defined as the phenomenon of 'generating false information' used in the field of AI, human hallucinations can be intentional or unconscious. It can be divided into things. Of these, those that occur unconsciously are caused by cognitive biases , or rules of thumb.
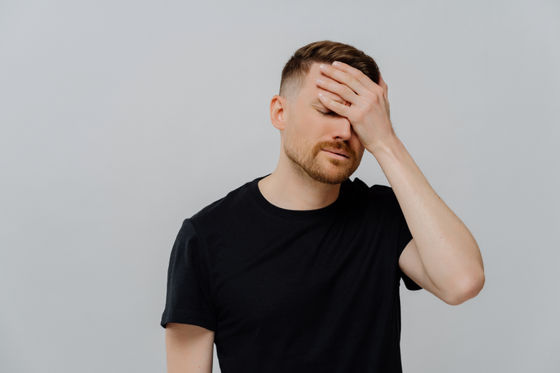
According to researchers Claire Naughtin and Sarah Vivian Bentley from the Australian Commonwealth Scientific and Industrial Research Organization (CSIRO), much of this bias stems from a need to do so.
Humans cannot process all of the information that is provided to them in large quantities by the five senses, so the brain fills in the gaps between pieces of information by making associations based on previous learning in order to quickly respond to the problems it is facing. I am.
In other words, the human brain infers the correct answer based on limited information, but if the lack of information is compensated for by other information, the person may say things that are not true without realizing that they are wrong. There will be cases where this happens. In psychiatry, this is called
Other biases that pose problems for modern people when dealing with AI include ' automation bias ,' where people place too much trust in automated decision-making systems like ChatGPT. The `` halo effect ,'' which affects subsequent evaluations, and the ``fluency heuristic ,'' in which when something is processed more fluently and smoothly than others, the value of that object is perceived to be higher than others. Heuristic) .
The important point here is that human thinking is dramatized by cognitive biases and distortions, and these hallucinations mainly occur outside of human consciousness.
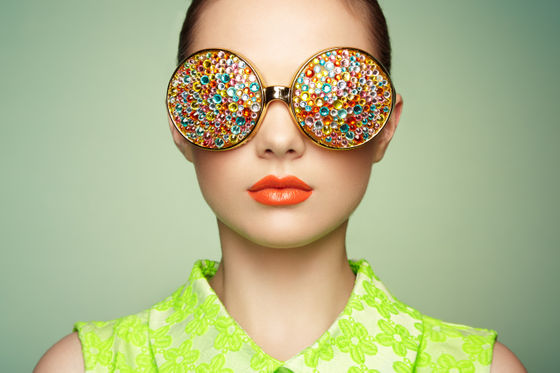
◆How does AI see hallucinations?
Unlike humans, large-scale language models do not need to conserve limited mental resources. Therefore, the AI's hallucinations simply indicate a failure in its 'attempt to predict an appropriate response to the input.'
On the other hand, there are also similarities between AI hallucinations and human hallucinations. That is the point where AI's illusions also arise from trying to fill in the gaps.
Based on the input word and the associations the system has learned through training, a large-scale language model predicts the next word likely to appear in a context and generates an answer based on this. In the process, like humans, they try to predict the most likely answers, but unlike humans, large-scale language models do not understand what they are generating. This is why incorrect answers are often output as illusions.
Large language models can hallucinate for a variety of reasons, but the main one is that they were trained on insufficient or flawed data. Other cases include cases where there is a problem with the learning system's program, or cases where the problem is exacerbated by human training.
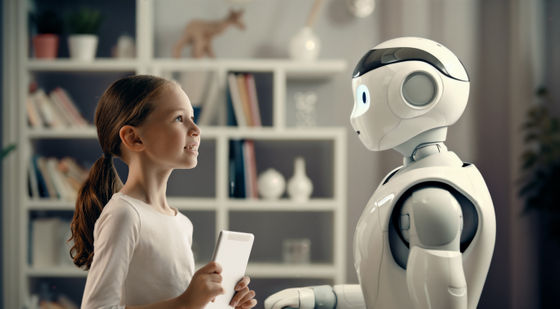
◆How to successfully interact with AI that causes hallucinations
Mr. Naughtin et al. said, ``Our failures and technology failures are two sides of the same coin, and if we fix one, we can fix the other,'' as a way to minimize the effects of AI illusions. We proposed the following three points.
・Responsible data management
As mentioned above, bias in AI is often due to lack of data or bias. This can be addressed by ensuring that training data is diverse and representative, building algorithms with bias in mind, and implementing techniques to filter out bias and discriminatory trends. Masu.
・Transparency and “explainable AI”
Even if you take the measures mentioned above, there are cases where bias remains in AI and it is difficult to identify it. By examining how bias enters the AI and propagates through the system, we can trace the source of the bias in the answers it generates. This is the concept of '
・Prioritizing public interest
Addressing the biases that exist in AI requires incorporating “human accountability and human values,” and achieving this requires stakeholders involved in AI to come from diverse backgrounds, cultures, and perspectives. We must ensure that we are representative of the people, Naughtin et al.
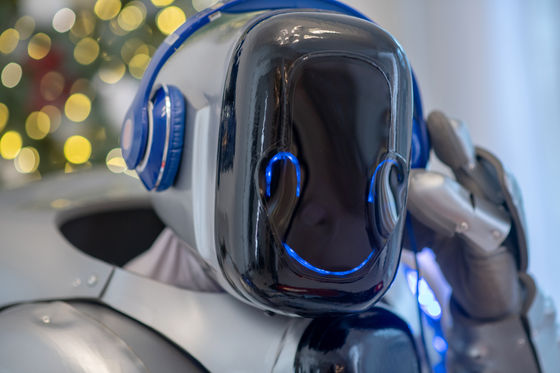
The realization of a system in which AI and humans cooperate while suppressing the influence of each other's 'hallucinations' has already begun. For example, in healthcare, machine learning systems are emerging that detect inconsistencies in human data and alert clinicians, allowing AI to improve diagnostic decisions while maintaining human accountability. I did.
Other projects include Amnesty International's Troll Patrol project , which uses AI to train human moderators to combat harassment of women on social media, and AI-based analysis of satellite images, which uses AI to help reduce the risk of low night lighting. There are also academic studies that identify poverty areas.
At the end, Nortin et al. conclude, ``It is important not only to do the essential work of improving the accuracy of LLM, but also not to ignore that the flaws in LLM serve as a mirror to ourselves.'' That's what I mean.''
Related Posts:
in Software, Posted by log1l_ks