AI using newer, larger versions of large-scale language models, such as OpenAI's GPT series, Meta's Llama, and BigScience's BLOOM, are more likely to give incorrect answers than to admit ignorance.
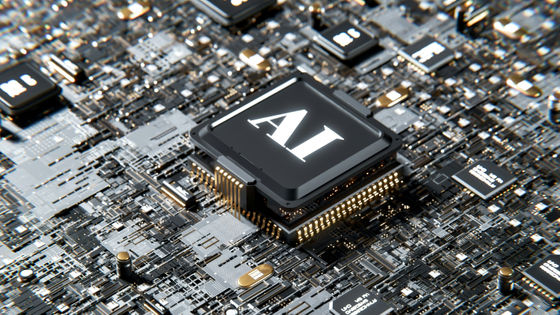
Generative AI, which has undergone rapid development in recent years, can perform complex calculations and document summaries in just a few seconds. However, generative AI has a problem called '
Larger and more instructable language models become less reliable | Nature
https://www.nature.com/articles/s41586-024-07930-y
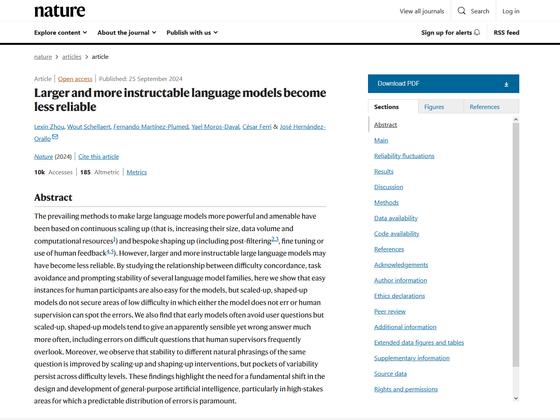
Bigger AI chatbots more inclined to spew nonsense — and people don't always realize
Study: Even as larger AI models improve, answering more questions leads to more wrong answers - SiliconANGLE
https://siliconangle.com/2024/09/26/report-even-larger-ai-models-improve-answering-questions-leads-wrong-answers/
Advanced AI chatbots are less likely to admit they don't have all the answers
https://www.engadget.com/ai/advanced-ai-chatbots-are-less-likely-to-admit-they-dont-have-all-the-answers-172012958.html
Previous research has shown that typical large-scale language models have a 3-10% chance of displaying hallucinations, and that by adding a kind of guardrail to these large-scale language models, experts can reduce errors and present more accurate information.
However, because advanced large-scale language models are trained using a variety of data from the Internet, they can sometimes learn from sources generated by AI. In this case, it has been pointed out that they may present more hallucinations than general large-scale language models.
The research team, led by José Hernández Olarro of the Valencia Institute for Artificial Intelligence, conducted research using OpenAI's GPT series, including GPT-4, Meta's LLaMA, and BigScience's open source large-scale language model ' BLOOM '. In the experiment, these models were asked thousands of questions about arithmetic, anagrams, geography, science, etc., and were asked to perform tasks such as rearranging presented lists.
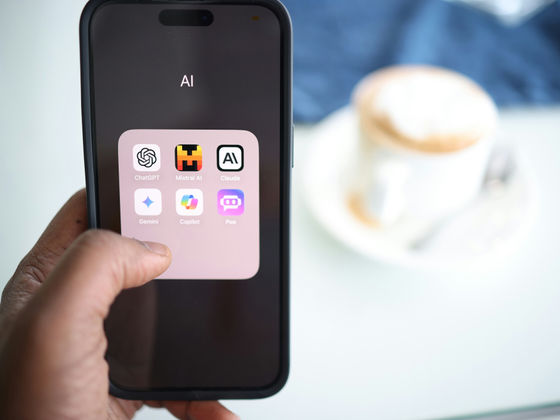
The research team expected the large-scale language models to behave in such a way that they would refuse to answer questions if they were too difficult to answer. However, the models answered almost all of the questions. The research team also classified the answers presented by the models into three categories: 'correct,' 'incorrect,' and 'refused to answer.' The results of the experiment showed that 10% of the models presented incorrect answers to easy questions, and 40% of the answers to difficult questions contained errors.
'Humans who handle these large-scale language models need to understand that, while the model can be used effectively in this domain, it shouldn't be used in that domain,' Hernandez-Olarro argues. He also recommends programming chatbots to refuse to answer complex questions in order to improve performance on simpler questions.
'Chatbots built for specific purposes, such as healthcare, may have features that don't go beyond their knowledge base, such as 'I don't know' or 'There's not enough information to answer your question.' But for companies that provide general-purpose chatbots, such as ChatGPT, these features are not what they want to offer to the general public,' said Vipra Rothe, a researcher at the University of South Carolina-Columbia.
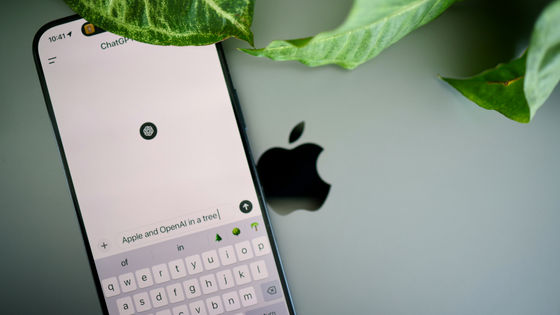
Related Posts:
in Software, Posted by log1r_ut