Although Google publishes a technical report of the large-scale language model 'PaLM 2', the information of the essential part is not described
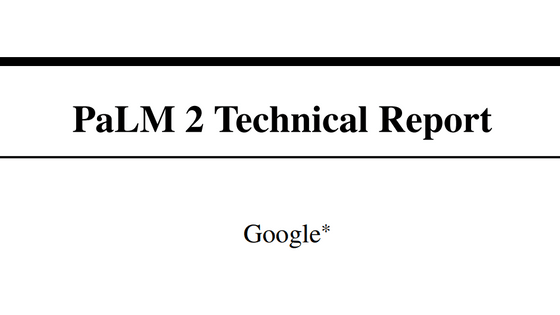
At
PaLM2 Technical Report (PDF)
https://ai.google/static/documents/palm2techreport.pdf
You can read more about what is possible with PaLM 2 in the article below.
Google announces large-scale language model 'PaLM 2', already installed in 25 Google services - GIGAZINE
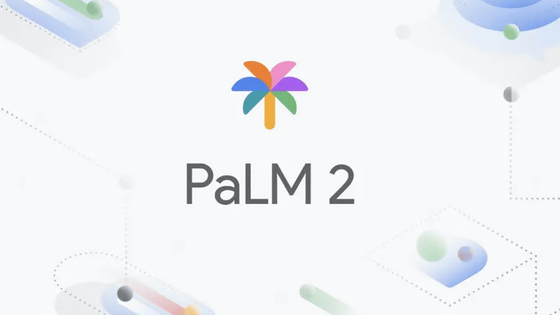
The PaLM 2 technical report is 92 pages in total, but only half of it describes the data used for training. According to its half-page, the training data for PaLM 2 included 'web documents,' 'books,' 'code,' 'mathematical formulas,' and 'conversational sentences,' on a larger scale than when PaLM was trained. It is said that the proportion of languages other than English has increased at the same time, but it is not stated how specifically the collected data was used.
Google isn't the only company keeping data private. In the technical report (PDF) of GPT-4, which is a competitor of PaLM 2, OpenAI, which developed GPT-4, said, ``Considering both the competitive environment and safety of large-scale models such as GPT-4, this report does not include additional details regarding architecture, hardware, training computations, dataset construction, training methods, or the like, including model size. The trend continues.
Regarding the secrecy of such information, Hacker News commented that ``the detailed information of the model has become so important that it affects the survival of the company, and the era of open information has ended.''
However, not all information is kept secret, and in the technical report of PaLM 2, 'the optimal relationship between the amount of computation, the size of the model, and the amount of data used for training,' 'efforts to protect privacy,' and 'human Investigation on the appearance of harmful responses such as species discrimination” etc. The horizontal axis in the figure below both represents the amount of computation, the vertical axis in the left graph is the optimal number of parameters (=model size), and the vertical axis in the right graph is the optimal number of tokens (=data used for training). amount). In this technical report, it is stated that the most efficient training can be achieved by increasing the model size and the amount of training data at the same rate as the amount of computation increases.
From the viewpoint of privacy protection, PaLM 2 said that the amount of verbatim memorization that ``exactly the same word as the trained word is generated'' was reduced compared to PaLM. It is stated that verbatim memory is less likely to occur even in languages with a small proportion of training data.
But the PaLM 2 technical report also reveals that more work remains to be done regarding adverse reactions across languages. For example, PaLM 2 produces more than 30% of adverse responses when given particularly harmful prompts, and in certain languages such as English, German, and Portuguese, racial identity, religion, etc. The prompt resulted in PaLM 2 having a 17% or greater chance of having an adverse response.
If you want to actually try interacting with PaLM 2, it is easy to use Google's AI chat service '
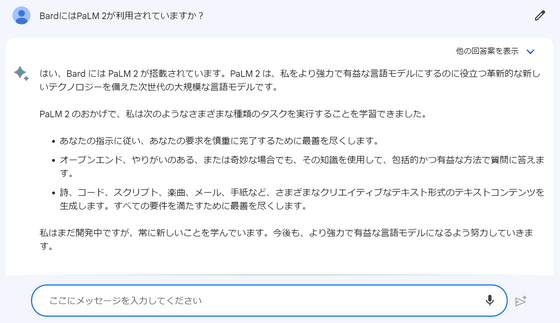
Related Posts:
in Software, Posted by log1d_ts