Open source GPT-based large-scale language model 'Cerebras-GPT' seven types can be downloaded by anyone at once
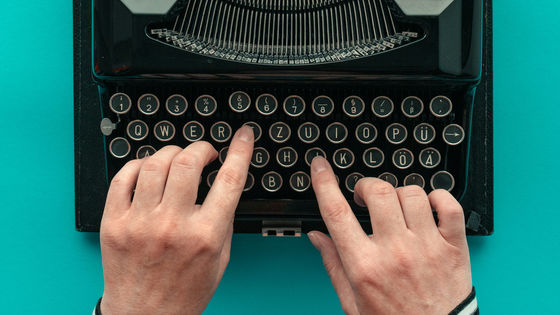
Cerebras, an AI company, has released seven types of open source large-scale language models ' Cerebras-GPT ' with 111 million to 13 billion parameters. Cerebras-GPT is a model trained by
Cerebras-GPT: A Family of Open, Compute-efficient, Large Language Models - Cerebras
https://www.cerebras.net/blog/cerebras-gpt-a-family-of-open-compute-efficient-large-language-models/
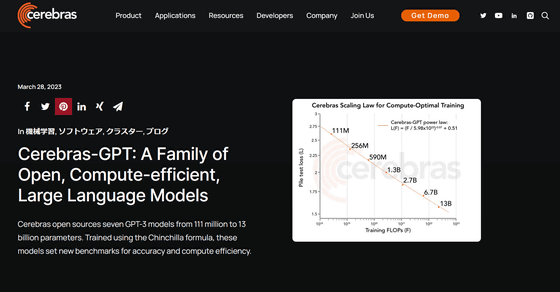
Cerebras (Cerebras)
https://huggingface.co/cerebras
GitHub - Cerebras/modelzoo
https://github.com/Cerebras/modelzoo
Below is a table summarizing open access and licenses for major large-scale language models. OpenAI's GPT-4 is not open including the model structure, and DeepMind's Chinchilla can only openly access the model structure. Meta's OPT is mostly open, but model weights are limited to researchers and licenses are limited to non-commercial use. On the other hand, Cerebras-GPT has all models, weights and checkpoints published on Hugging Face and GitHub under the Apache 2.0 license .
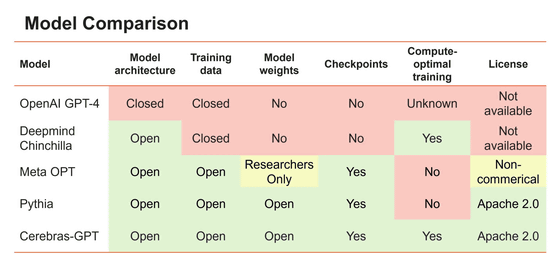
“For LLM to be an open and accessible technology, we believe that access to state-of-the-art models that are open, reproducible and royalty-free are critical for both research and commercial applications,” said Cerebras. I am.”
Cerebras-GPT is said to be learning in a few weeks on the CS-2 system, which is part of
Below is a graph showing the computational efficiency of Cerebras-GPT (orange) and Pythia (green), a large-scale language model of EleutherAI . The vertical is the data value lost during learning, and the horizontal is the computational complexity (logarithmic display) in learning. The smaller the slope of this graph, the higher the learning efficiency.
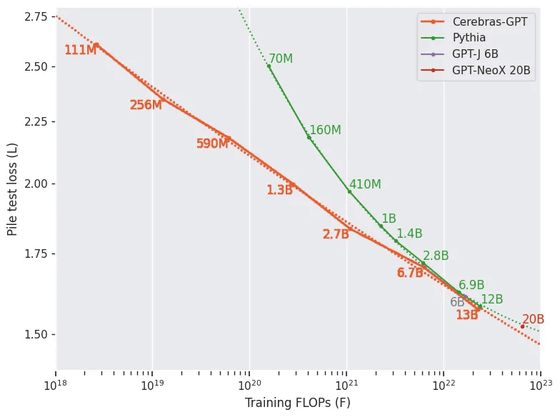
Cerebras also appealed that Cerebras-GPT maintains high learning efficiency in
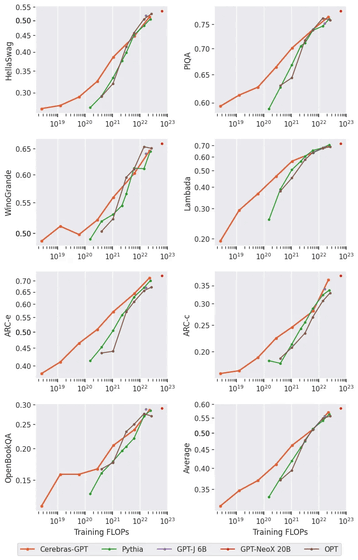
“We hope that Cerebras-GPT will serve as the first public large-scale GPT model family with state-of-the-art learning efficiency, as a recipe for efficient learning, and as a reference for further community research,” said Cerebras. In addition, through Cerebras AI Model Studio, we are making both the infrastructure and models available on the cloud.We will further develop the large-scale generative AI industry through better training infrastructure and community sharing. I believe we can do it,' he commented.
Related Posts:
in Software, Posted by log1i_yk