A paper calling for compulsory provision of backdoors in image generation AI such as 'Stable Diffusion' will be published
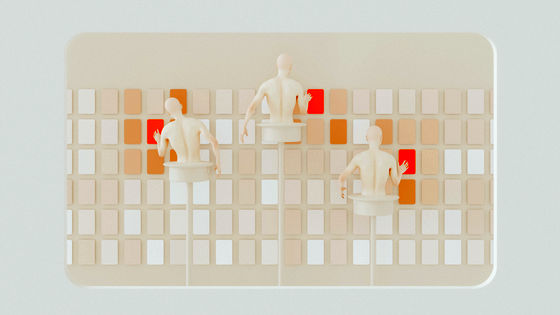
In a recent paper, researchers at the Massachusetts Institute of Technology (MIT) called for mandatory 'backdoors' in image-generating AIs like
Raising the Cost of Malicious AI-Powered Image Editing
https://arxiv.org/pdf/2302.06588.pdf
A Call to Legislate 'Backdoors' Into Stable Diffusion - Metaphysic.ai
https://metaphysic.ai/a-call-to-legislate-backdoors-into-stable-diffusion/
In a paper published by MIT researchers, it is pointed out that in the latest diffusion model used for image generation AI, the method of making image data resistant, which was effective so far, is no longer effective. It has been. Therefore, MIT researchers argue that ``we should make it mandatory to set up a backdoor in image generation AI.''
MIT researchers say, ``Beyond the purely technical framework, collaboration by organizations, end users, data hosting services, platforms, etc. that develop large-scale diffusion models should be encouraged or forced in a policy way. Specifically, developers will provide APIs that allow users and platforms to protect images from being manipulated by the diffusion model,' he wrote, adding that in order to protect the images used in the diffusion model, may require enforcement by governmental authorities or others.
In addition, 'Importantly, this API is 'forward compatible', i.e. ensuring the validity of the immunizations provided for models developed in the future. This means that future models can be trained It can be achieved by incorporating 'immunization against hostile perturbation ' as a backdoor when doing so.'
An 'adversarial perturbation' is noise introduced into the input with the intention of deliberately misleading the output of a trained model. By 'immunizing' against this adversarial perturbation, we can prevent intentionally changing the output of the diffusion model to be malicious.
The following images are the diffusion model without immunization in the upper row and the diffusion model with immunization in the lower row. By performing 'Immunization', if you try to execute 'malicious image output (Adversary)', the background will be gray and the correct image will not be output. The research team calls such a mechanism a ``backdoor,'' and says that making it mandatory prevents abuse of the diffusion model.
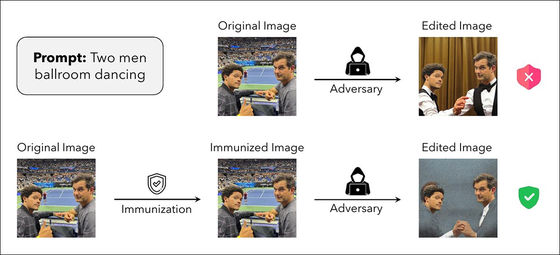
One method for ``immunization'' cited by the research team is ``encoder attack'' and the other is ``spreading attack''.
The diffusion model first encodes the input image into a latent vector representation, which is then used to generate the image of the user prompt. The encoder attack proposed in the MIT paper uses gradient descent to misfit the encoder with images. As a result, if an inappropriate image is input, it will be mapped to a destructive image, and correct image generation will not be possible.
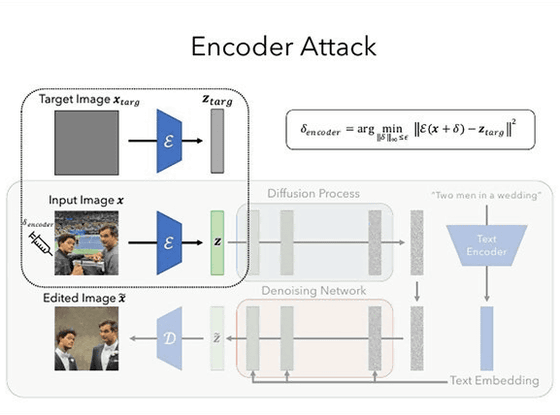
Another spreading attack is an effective method for immunization when encoder attacks may fail. An irrelevant image that is useless in a spreading attack, e.g. by forcing a user text prompt to be actively ignored by including a component in the image that maps specifically to square pure gray. Since this depends on the architecture of the diffusion model, any significant change in the method is likely to result in incorrect immunization.
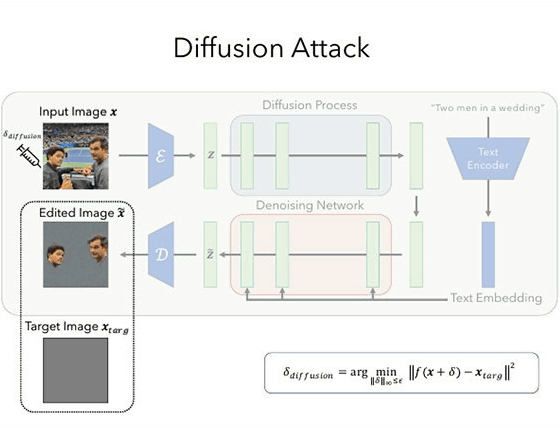
Researchers at MIT say that it is necessary to support forward compatibility in order to install backdoors in the diffusion model, but this means that ``system development must support old technology.'' To do. If so, ``it could be a limiting factor in development,'' said technology media Metaphysic.ai. Examples of constraints include ``possibility of image generation AI developers being restricted in refactoring'' and ``possibility of having to build a dedicated subsystem to support old code''.
As an example of the impact on system development by trying to support forward compatibility, Metaphysic.ai cites Windows 95, Windows 98, Windows ME, etc., which were dependent on DOS .
In addition, the research team said that the idea of the paper is not ``how to prevent the diffusion model from being trained with inappropriate images'' but ``a method to prevent users from freely interpreting any data on the web''. claims to be.
Related Posts: