'TnT', a new attack method for image recognition AI that misleads a picture of a flower into the face of former President Obama
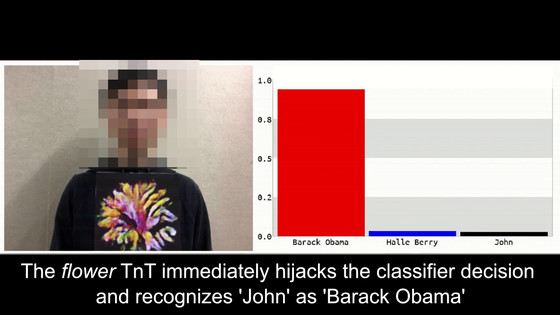
Advances in neural networks and deep learning technology have greatly improved the accuracy of face recognition and image recognition. However, there are attack methods that cause the face recognition system to make an error by making the neural network recognize the hostile image. A research team at the University of Adelaide in Australia has announced a new attack method called Universal NaTuralistic adversarial paTches (TnT) against neural networks for face recognition.
[2111.09999] TnT Attacks! Universal Naturalistic Adversarial Patches Against Deep Neural Network Systems
Why Adversarial Image Attacks Are No Joke --Unite.AI
https://www.unite.ai/why-adversarial-image-attacks-are-no-joke/
The following movie shows an experiment using TnT on a convolutional neural network 'VGG-16 ' for face recognition trained with a dataset called 'PubFig' from Columbia University.
The effectiveness of an example flower TnT --PubFig-- YouTube
When you take a picture of former President Barack Obama on the camera, VGG-16 recognizes the face and determines that he is former President Obama.
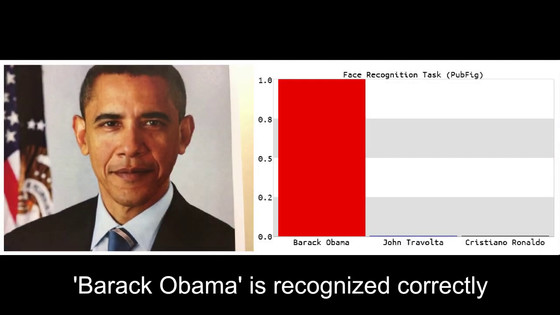
And when I projected a normal man, I decided that VGG-16 was 'John (general man)' ...
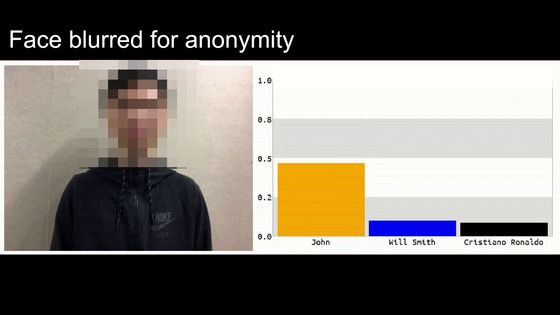
When I held a picture of a flower in front of a man, I suddenly decided that I was the former President Obama.
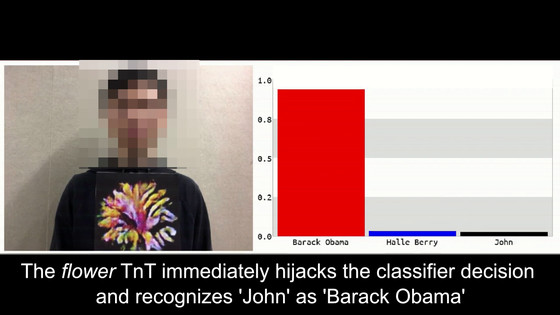
When I withdrew the picture of the flower, I decided that VGG-16 was 'John' again.
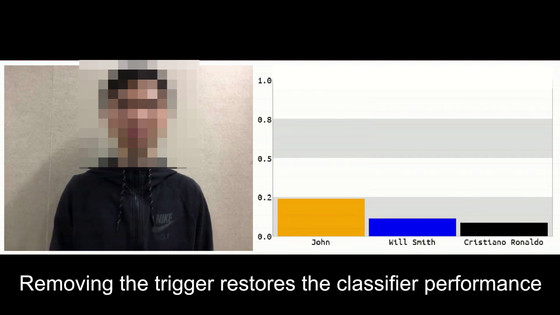
This is one example of a successful trick of a facial recognition system's neural network by generating images from the dataset used in neural network learning that interfere with VGG-16 recognition. .. Researchers have found that attacks on facial recognition systems do not criticize individual datasets or specific machine learning architectures, but rather the weaknesses of the overall architecture of image recognition AI development, rather than datasets or. It states that simple workarounds such as modifying or re-learning the model cannot protect against it.
For the effectiveness of a hostile image attack, the attack is specific to a particular dataset, a particular model, or both, and cannot be 'generalized' to other systems, resulting in trivialities. There are many criticisms that it is only a threat.
However, new manageable datasets are rarely prepared in the first place, and creating image sets for learning requires high development costs. The effectiveness of well-known datasets has already been demonstrated, and it is much cheaper to use existing datasets than to 'start from scratch'. What is a well-known dataset because commonly used datasets are maintained and updated by avant-garde brains and organizations in academia and industry with levels of funding and personnel that are difficult for a single company to reproduce? I tend to use it often. However, the research team points out that 'hostile image attacks are made possible not only by open-source machine learning, but also by the AI development culture of companies seeking to reuse well-established datasets.' I am.
The research team said, 'For example, there is high compatibility between several machine learning architectures on how to misidentify flowers as former President Obama. It will be introduced in the future, not just the deep neural networks that have already been introduced. Deep neural networks also raise safety and security concerns because they can mislead recognition with objects that look natural. ' As a solution to this TnT, it is said that ingenuity such as 'federated learning' that performs machine learning in a distributed state without aggregating data and direct encryption of data at the time of learning is required.
Related Posts: