Make AI Make AI
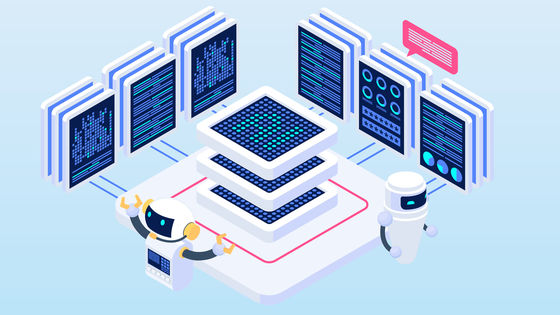
Artificial intelligence (AI) identifies and learns patterns of data, so it requires sufficient data and processing power. The demand for processing power in the AI development field is increasing year by year, and AI developers must carefully adjust the parameters of millions to billions.
Researchers Build AI That Builds AI
https://www.quantamagazine.org/researchers-build-ai-that-builds-ai-20220125/
It is important to find values that are as close to ideal as possible in a process called 'optimization' for building artificial intelligence, but it is not easy to train the network to reach this point. Regarding such a esoteric process, Peter Berikovic , a researcher at DeepMind , an artificial intelligence company under the same Alphabet as Google, said, 'This difficulty may change soon. Boris Nayazev of Gerf University in Canada and others. Designed by 'HyperNetwork,' it can speed up new training processes and, in theory, predict parameters in fractions of a second, eliminating the need for training. ' Is stated.
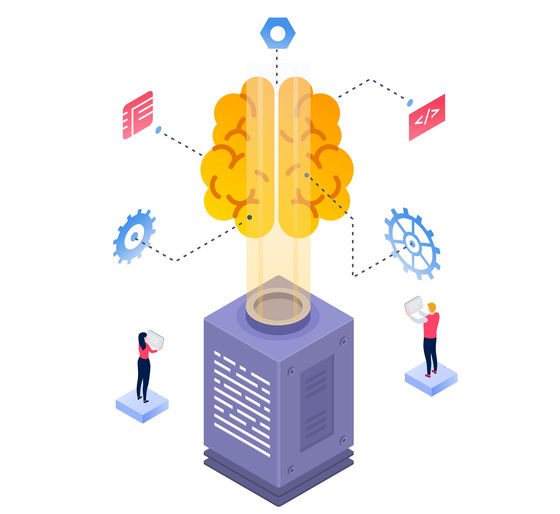
As of January 2022, Stochastic Gradient Descent (SGD) is the best way to train and optimize deep-neutral networks, according to Ananthus Whammy. SGD is the process of adjusting network parameters to collect large amounts of data to reduce errors and losses and iterate to minimize losses.
However, SGD only works once you have an optimized network, so you need to rely on the intuition and heuristics of your engineers when building new early neural networks. So, in
Mr. Naiyazev's hypernetwork is also built on the idea of GHN, and in the paper published by Mr. Naiyazev et al. In October 2021, not only finding the optimum architecture from the prepared candidates, but also the optimum in the absolute sense. How to predict various parameters was shown. Ren praises Naiyazev's paper as 'it contains far more experiments than we did.' Naiyazev's team calls this hypernetwork 'GHN-2'.
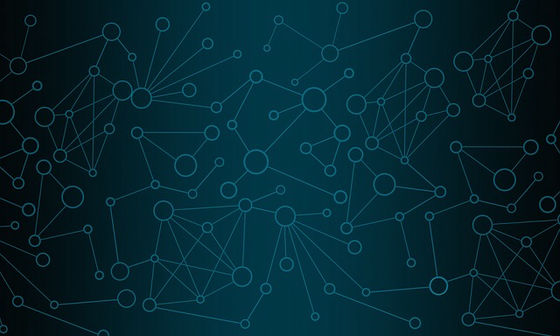
For an image dataset, the average accuracy of the in-distribution architecture predicted and derived by GHN-2 is 66.9%, which is close to the average accuracy achieved by SGD 2500 iteratively trained networks of 69.2%. He said he did. It seems that GHN-2 does not work well as the data set grows, but it is still not inferior to the accuracy obtained by training SGD, and most importantly, GHN-2 makes predictions at an overwhelming speed. I am. 'GHN-2 has achieved surprisingly good results,' said Naiyazev. 'GHN-2 significantly reduces energy costs.'
Mr. Naiyazev says that GHN-2 still has room for improvement and it is difficult to adopt it immediately, but with the spread of hypernetworks, designing and developing new deep neutral networks other than companies with big data. When it is possible, it shows the possibility of 'democratization of deep learning' as a long-term future prospect.
Related Posts:
in Science, Posted by log1e_dh