Succeeded in automatically generating images with the technology of the text generation tool 'GPT-2' which was regarded as 'too dangerous'
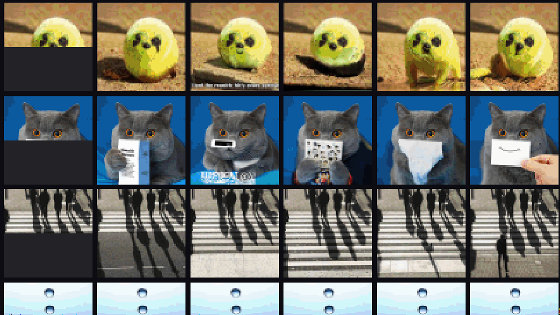
AI's text generation tool ' GPT-2 ' developed by
Image GPT
https://openai.com/blog/image-gpt/
Transfer learning is a technique for transferring a model learned in one area to another area, and GPT-2 has achieved great success with this transfer learning model. In addition to GPT-2, unsupervised learning that does not involve humans has made remarkable progress with Google's ' BERT ' and Facebook's ' RoBERTa .' On the other hand, in the field of natural language, the success of transfer learning models is remarkable, but until now, no powerful function has been generated in unsupervised and transfer learning models in images.
Therefore, OpenAI made a new attempt to 'train the pixel sequence so that the sample and the finished product have image consistency with the same transfer learning model as GPT-2'.
As a result, a technology has been developed that allows the model to complete the image just by giving 'half the image' by humans. In the images below, the left end is the input image, the right end is the original image, and the middle four are the images generated by the model. The image in the second column from the left is the 'favorite' selected by the researchers.
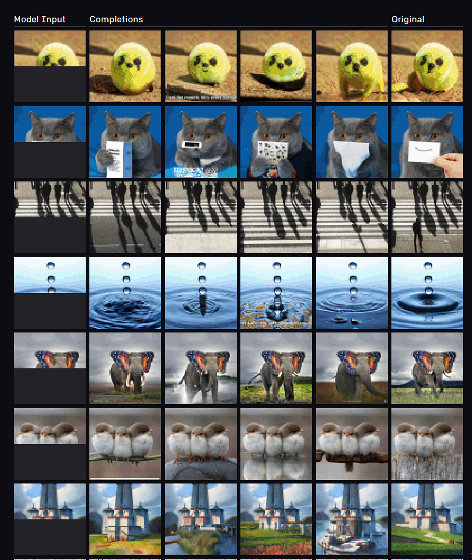
Besides this, the image generated by the model looks like this. We're not just talking about successful image generation, it's all at this level.
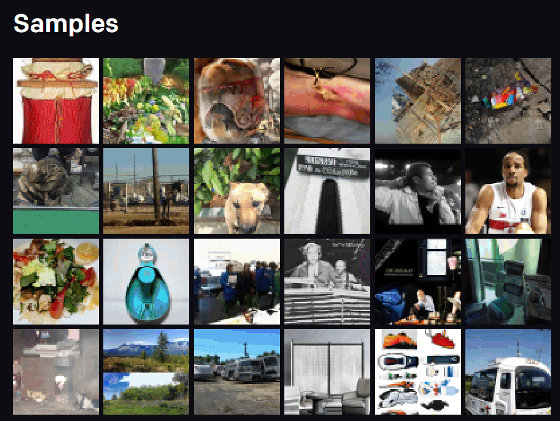
OpenAI has three versions of the GPT-2 model called 'iGPT-S' with 76 million parameters, 'iGPT-M' with 455 million parameters and 'iGPT-L' with 1.4 billion parameters ImageNet Training in. At the same time, I trained the “iGPT-XL” with 6.8 billion parameters using ImageNet and images on the Internet. Each model then reduced the image resolution and created its own 9-bit palette representing the pixels, producing an input sequence that was three times shorter than the standard RGB spectral standard without compromising accuracy.
According to OpenAI, the quality of the generated images increased sharply with increasing depth, and then gradually declined. This is because the transfer learning model consists of two phases: 'gathering information from the environment to create image features' and 'a contextual feature is used to predict the next pixel'. The researchers explain.
It has also been shown that increasing the model size and doing more iterative training improves the image quality and gives better benchmark results than other supervised and unsupervised models.
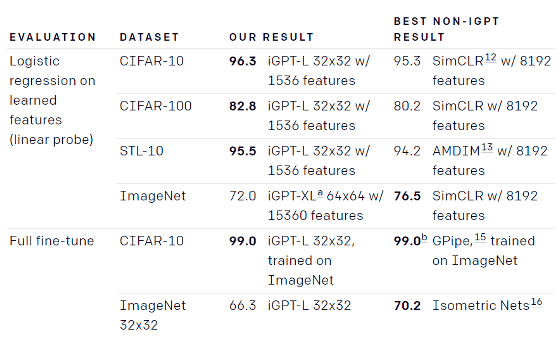
However, this iGPT model is limited in that it will be a low-quality image generation and that it will be biased by training data, and it will only function as a proof of concept demonstration. On the other hand, researchers say that the research results are a 'small but important step' that bridges the gap between 'computer vision' and 'language understanding technology.'
Related Posts: