Tips on implementing the experimental method “A / B test” that has dramatically improved the profitability of all sites
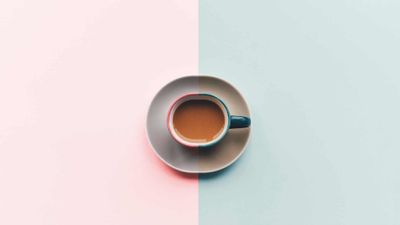
" A / B test " is an experimental method that can collect highly reliable data by scientifically backed experiments. The Harvard Business Review provides helpful tips for introducing A / B tests based on the experience of Ron Kohabie, general manager of Microsoft's experimental and analytical team.
A / B Testing: How to Get It Right
https://hbr.org/2017/09/the-surprising-power-of-online-experiments
◆ What is an A / B test?
A / B testing is a method to identify multiple sentences and images to identify which performance is high in order to increase conversion rate. When Microsoft engineers introduced it into Bing's search ad in 2012, they increased revenue by as much as 12 percent without affecting key user experience metrics.
Today, not only Microsoft, but also Amazon, Booking.com, Facebook, Google, and many other companies are testing A / B for millions of users. A / B test is active in various situations, but if you try to do A / B test actually, how to design the experiment, how to keep integration, how to interpret the result, etc. The problem of
◆ Small changes can have a major impact <br> Generally, it is often thought that the high cost of development costs is related to success or failure, but it is not about making major changes to get to success You need to know that it is important to stack many small changes. In the case of Microsoft, the click-through rate increased by 8.9%, simply by changing the page transition when clicking the link to Hotmail on the MSN site to open in a new tab instead of opening in the current tab. Did.
If you try similar changes in the search results of MSN whether "Open in a new tab" is effective, the number of clicks on search results per user has increased by 5%. Opening links in a new tab is one of the best ways to get the user's attention, and is now used on a variety of sites such as Facebook and Twitter.
Also, according to Amazon's experiments, moving credit card offers from the home screen to the shopping cart page has increased billions of dollars in revenue annually. While such minor changes can make a big difference, the project of displaying Facebook and Twitter content in Bing's search results cost billions of dollars, but it has a significant impact on engagement and revenue. It did not seem to be.
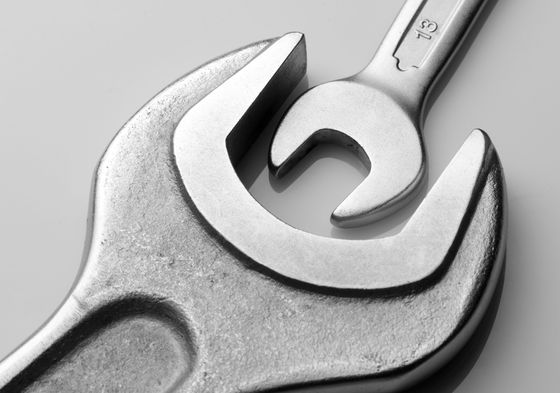
◆ It helps to decide which field to invest in
A / B testing can also help determine what to improve. For example, in the case of Bing, it was possible to quantify the value of shortening the time taken to display search results. Specifically, as a result of deliberately examining the delay, the revenue decreased 0.6% every 100 ms of delay time. With Bing's annual sales of over 300 billion yen, you can see that you can spend up to 1.8 billion yen for speed improvement of 100 milliseconds. Such quantitative information has helped Bing to make decisions when faced with the tradeoff between search results relevance and response speed.
◆ Prepare the infrastructure for testing <br> Of the new ideas, it is a difficult task to decide which ideas succeed and which fail. Looking at all of Microsoft's experiments, it turns out that about one third is effective, the other third has no effect, and the last third is a negative result. You are You need to do a lot of experiments to get better results. Bing says that 80% of the proposed changes will be tested first.
To test scientifically the proposed changes one after another requires an infrastructure to collect and analyze data. With over 80 members at Microsoft's experimental and analytical team, you can run hundreds of experiments at any time with products such as Bing, MSN, Office, Windows, Xbox and more. As testing is performed, statistical analysis is performed on the results, and scorecards are generated automatically to make it easier to find significant effects.
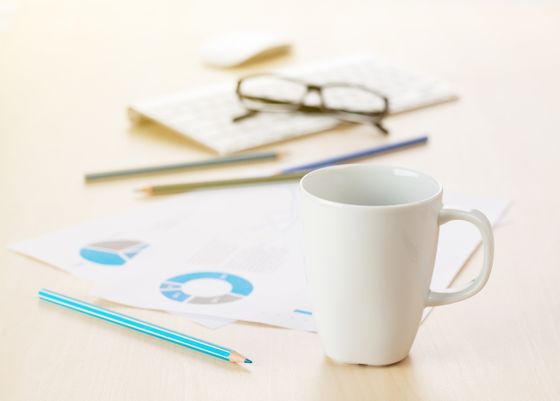
◆ Define Success <br> To evaluate your experiment, you need to decide which metric you want to target. It may seem simple at first glance, but it is difficult to look at the short-term changes of several indicators and decide which changes can be made to make a long-term forecast. It is said that it is good to review evaluation criteria once after deciding once.
Bing is a search engine, but less relevant search results will increase revenue in the short run as users search more often. But, of course, in the long run, users are expected to switch to another search engine, which is a factor that reduces revenue. Bing seems to minimize the number of user searches while maximizing the number of tasks and sessions performed by the user.
◆ Maintaining data quality <br> No matter how good the evaluation criteria are, it makes no sense if the data obtained from the experiment is unreliable. Having said that, it's also a difficult task to make sure that the data is reliable.
One way is to do an A / A test. Test the exact same thing, and if it is detected that there is any difference, it means that you have misconfigured something. It's a simplistic approach, but at Microsoft A / A testing seems to have found hundreds of invalid experiments and incorrect formulas.
Also, Bing found that more than 50% of search requests came from bots, so it was necessary to remove such data to reduce noise. In addition, management of outliers is also important, as some users such as the library place a large number of orders on Amazon, which greatly affects A / B testing.
Some segments may have a major impact. In Bing, a bug in JavaScript prevented Internet Explorer 7 users from clicking on the search results, and the results were supposed to be good, and the results were sometimes displayed badly. These bugs can be overlooked if you only look at the average result.
Also, be careful if the ratio of people randomly assigned in the A / B test deviates. For example, if the ratio of people randomly assigned to A / B is “82,588 vs. 81,5482”, then the ratio is “50.2% vs. 49.8%”, but such a bias has happened by chance. There is only a half a million chance of occurrence, and there is a high possibility that some kind of mistake has occurred.
_m.jpg)
◆ Don't estimate causality <br> Even if you estimate causality by observing it, it is difficult to judge whether it is correct. In order to establish a causal relationship, it is not sufficient to simply observe, but it is necessary to carry out appropriate experiments, for example, in the medical field, randomized comparisons by the US Food and Drug Administration to ensure that the drug is safe and effective. It is mandatory to conduct an exam.
Although A / B testing is a powerful tool to prove causality, it may not be clear why you understand causality. It seems that Bing's profit has increased greatly by just changing the color scheme of the screen slightly, but I do not know the reason for its clarity. However, the results of the A / B test show up as evidence, so we can move in the right direction in the rough, swirling online world.
Related Posts:
in Note, Software, Web Service, Posted by log1d_ts