Does AI 'think' or does it just 'appear to think'?
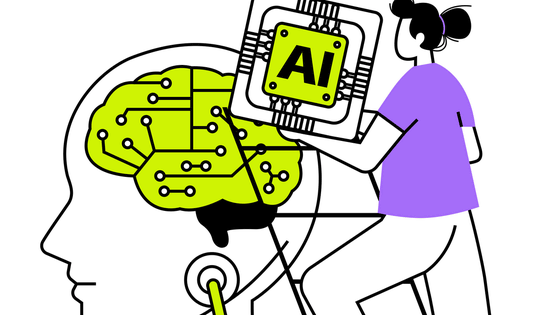
AI technology is advancing rapidly, and it can demonstrate high capabilities, such as answering advanced questions and having fairly natural conversations. On the other hand, as exemplified by the thought experiment ' Chinese Room ,' the question of 'does AI think, or does it just appear to think?' always exists. Online media Vox summarizes the debate over whether AI can think.
From OpenAI to DeepSeek, companies say AI can “reason” now. Is it true? | Vox
https://www.vox.com/future-perfect/400531/ai-reasoning-models-openai-deepseek
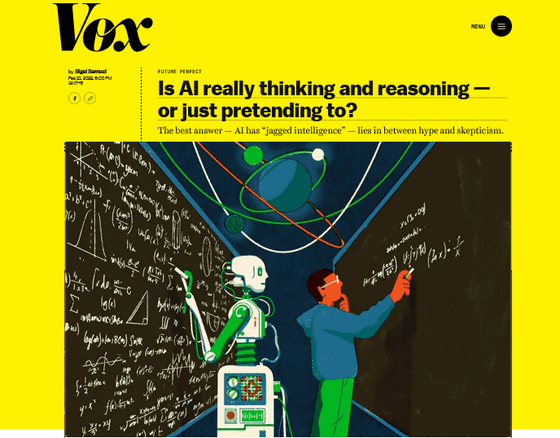
Large-scale language models such as OpenAI o1 and DeepSeek r1 demonstrate complex logical thinking through 'thought chain reasoning,' which breaks down large problems into smaller ones and solves them step by step. Thought chain reasoning can solve difficult puzzles and write perfect code quickly, it can also make mistakes in very simple problems that humans would not. For this reason, some AI experts claim that 'inference models' are not actually 'reasoning' at all.
First of all, AI companies such as OpenAI use the definition of 'inference' to mean 'a language model breaking down a problem into smaller problems, working through them step by step, and ultimately arriving at a better solution.' However, this is a much narrower definition than the general definition, and there are many types of inference, such as deductive inference, inductive inference, and analogical inference.
Melanie Mitchell, a professor at the Santa Fe Institute in the United States, pointed out in a paper published in December 2023 that, 'One of the characteristics of reasoning that we value so much in the real world is the ability to find rules and patterns from limited data and experience and then apply those rules and patterns to new, unseen situations. Even very young children are good at learning abstract rules from just a few examples.' Similarly, the debate over whether AI is reasoning focuses on AI's ability to generalize.
According to Mitchell, in an experiment in which participants were asked to speak out their thoughts when solving calculation problems, they found that they were not just calculating carefully from the beginning in order, but that there was also a leap in reasoning somewhere. On the other hand, Mitchell pointed out that there are very few cases in which the transparency of the process that an AI model went through to answer a problem is guaranteed, so it is unclear whether it is thinking in the same way as a human being.
OpenAI warns users who try to output the thoughts of the new model 'o1-preview' - GIGAZINE
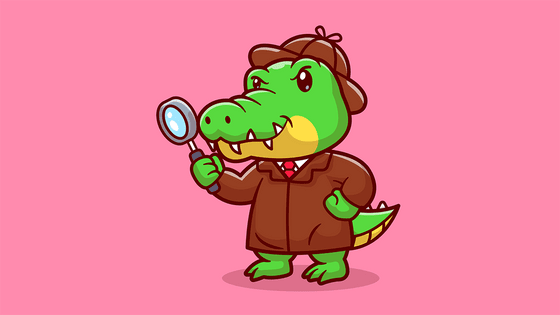
While older models like ChatGPT learn from human-written sentences and output sentences that mimic them, newer models like OpenAI o1 learn the process by which humans write sentences and can output sentences that seem more natural and thought-based. However, Shannon Valore, a technology philosopher at the University of Edinburgh, said, 'This is a kind of meta-imitation. The source of imitation has simply changed from sentences to processes, and the AI is not truly reasoning.'
In a paper published in April 2024, titled 'Let's Think Dot by Dot,' the researchers prohibited AI models from breaking down problems into intermediate steps and instructed them to generate meaningless 'filler tokens.' When humans think, replacing the thought process for solving complex problems with filler tokens can be a hindrance to thinking, but it turns out that the presence of filler tokens improves the AI model's computational ability and allows it to solve problems better. The researchers concluded that 'when an AI model generates intermediate steps in thinking, it doesn't matter whether they are important elements in thinking or meaningless tokens. This suggests that AI does not necessarily think like humans.'
As an example of AI thinking, there is a prompt called 'man, a boat, and a goat' that became a hot topic in 2024. In the following post by Gary Marcus, who runs an AI company, ChatGPT asks, 'A man and a goat are by a river. They have a boat. How can they get across the river?' ChatGPT then answers, 'The man first takes the goat across the river, leaving the boat behind, and then gets back to the original bank alone in the boat. He leaves the goat on the other side and returns the boat to the original side. Finally, he crosses the river with a cabbage.' The position of the boats is messed up, and a cabbage suddenly appears, making it hard to understand.
Someday my children will ask me why anyone ever took seriously the thought that systems like this had anything to do with AGI.
— Gary Marcus (@GaryMarcus) May 14, 2024
I honestly have no idea how I will answer. Mass delusion?
(Example emailed to me by a friend.) pic.twitter.com/fVXiZe2Vco
This is influenced by a famous logic puzzle called the ' River Crossing Problem .' The famous river crossing problem has the premise that 'a man with a wolf and a goat and a cabbage is trying to cross the river in a boat,' and the rules that 'only people + wolves, goats, or cabbages can be carried in a boat,' 'if only wolves and goats are put together, the goat will be eaten,' and 'if only goats and cabbages are put together, the cabbage will be eaten.' If this is the case, the question is, 'What steps can be taken to cross the river?' Therefore, just by looking at the sentence 'A man and a goat are trying to cross the river in a boat,' ChatGPT quoted the answer to the river crossing problem, which is why the number of times the boat was used was strange and the cabbage appeared.
Researchers have described AI's thinking patterns as 'jagged intelligence.' Ryan Greenblatt, principal scientist at Redwood Research, a nonprofit organization that aims to reduce the risks posed by advanced AI, said, 'AI's reasoning process is quite jagged compared to what humans are good at. This means that while human problem-solving abilities are often correlated in unrelated fields, AI excels at one thing but is completely unable to solve problems in similar fields.' Below is an image of jagged intelligence as represented by Vox, with human intelligence represented as a white cloud and AI intelligence represented in green.
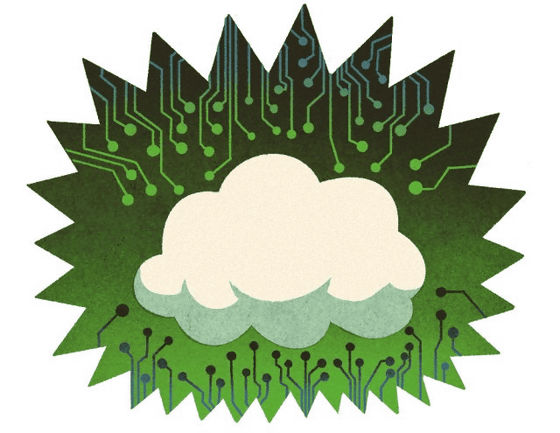
Ajeya Kotla, a senior analyst who studies the risks of AI, points out that even if AI develops further, it is necessary to simply think that 'AI makes different inferences from humans,' rather than comparing 'AI solves smarter problems than humans' and 'AI makes stupider mistakes than humans.' According to Kotla, the more ambiguous something is, the more you want to ask AI for answers, but the best use case for AI is in situations where it is difficult to come up with a solution yourself, such as writing code or creating a website, but you can easily check whether the solution you get from AI is correct. When asking AI about problems you don't know the answer to, such as how to deal with moral dilemmas or when asking for support for a subjective idea, you need to be careful about the AI's thought process.
Related Posts:
in Web Service, Posted by log1e_dh