Meta announces a heap of new information, including 'Meta Video Seal,' an AI tool that applies invisible watermarks to AI-generated videos, and 'Meta Motivo,' which controls the movement of humanoid models
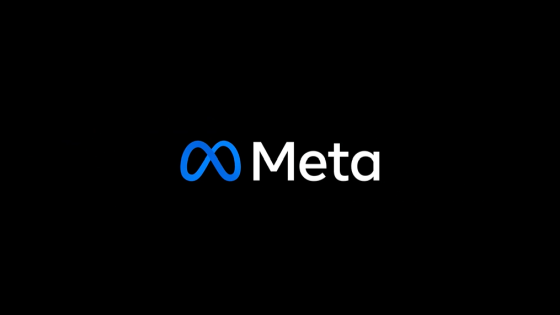
On December 12, 2024, Meta announced several research results on advances in AI and machine learning. They released tools that allow users to identify the origin of AI-generated videos by adding watermarks that are invisible to the naked eye.
Sharing new research, models, and datasets from Meta FAIR
◆Meta Video Seal
Meta Video Seal is a 'state-of-the-art, comprehensive framework for neural video watermarking,' a tool that adds watermarks invisible to the naked eye to videos and allows them to be viewed later. The watermarks are resistant to video editing such as 'blurring' and cropping, and to compression processing when uploading online, so the watermarks remain intact. In addition to simple watermarks, it is said that you can also add hidden messages to videos.
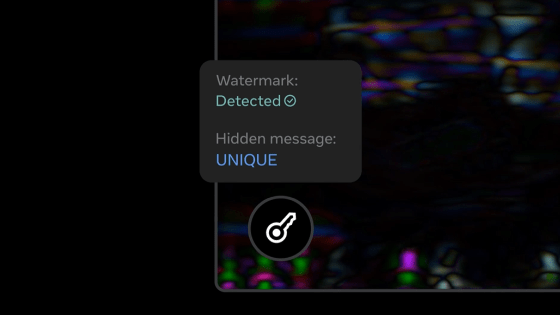
Along with Video Seal, the company is also releasing a watermark-specific leaderboard called the Meta Omni Seal Bench to make it easier for researchers to participate in the community, and it is re-releasing its AI model that can add watermarks, Meta Watermark Anything, with a 'more permissive' license.
Meta said, 'We hope other researchers and developers will join our efforts by integrating watermarking capabilities when building their generative AI models. Watermark Anything, Video Seal, and our previously published work, Audio Seal, are all available for download and ready to be integrated.'
Video Seal: Open and Efficient Video Watermarking | Research - AI at Meta
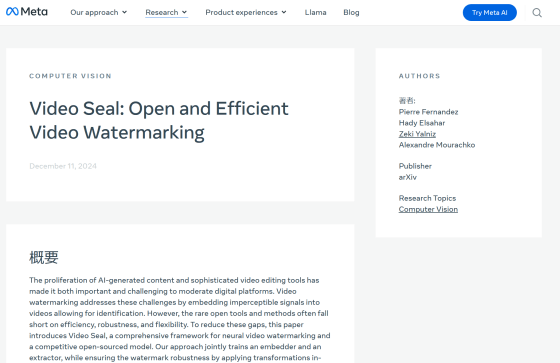
◆Meta Motivo
Meta Motivo is 'the world's first behavior-based model for controlling the movements of virtually embodied humanoid agents to perform complex tasks.' It solves tasks such as motion tracking, reaching goal poses, and reward optimization, and is able to achieve natural, lifelike movements that mimic human movements.
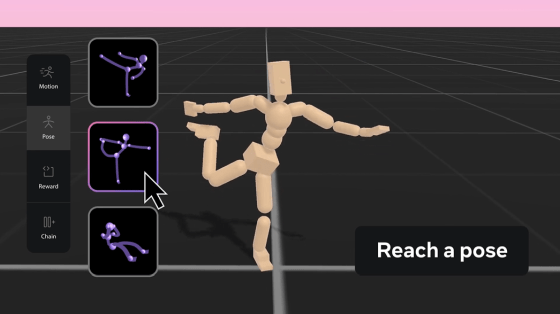
Many of the techniques known as 'unsupervised reinforcement learning' in machine learning use highly curated datasets and loss functions that are weakly related to the task. In contrast, Meta Motivo uses a new algorithm to learn representations that embed states, actions, and rewards in the same space. This makes it possible to solve tasks that require learning human-like actions without additional training or planning.
Meta Motivo
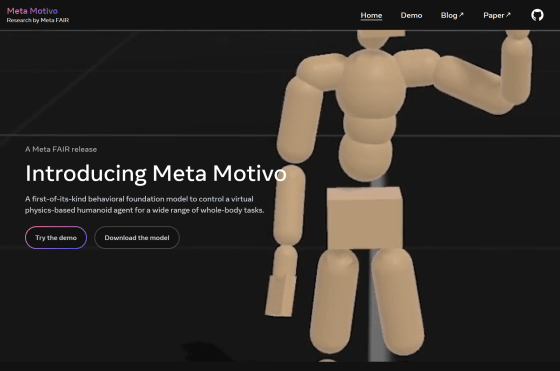
◆Flow matching
Flow Matching is a tool that helps generate images, videos, audio, music, and 3D structures of proteins. Flow Matching provides a simple yet flexible generative AI framework that improves performance and efficiency. Meta has published several papers and codes, as well as state-of-the-art training scripts, to make Flow Matching easy to use and accessible to the research community.
Flow Matching Guide and Code | Research - AI at Meta
https://ai.meta.com/research/publications/flow-matching-guide-and-code/
◆Meta Explore Theory-of-Mind
There is a research field called Theory-of-Mind that explores the ability of AI to understand and model the thoughts, intentions, and emotions of humans, other AIs, and other agents. Meta says that the existing Theory-of-Mind dataset has limitations, and in order to improve this and get closer to realizing advanced intelligence, they are releasing the new Meta Explore Theory-of-Mind to advance the research field.
Explore Theory-of-Mind: Program-Guided Adversarial Data Generation for Theory of Mind Reasoning | Research - AI at Meta
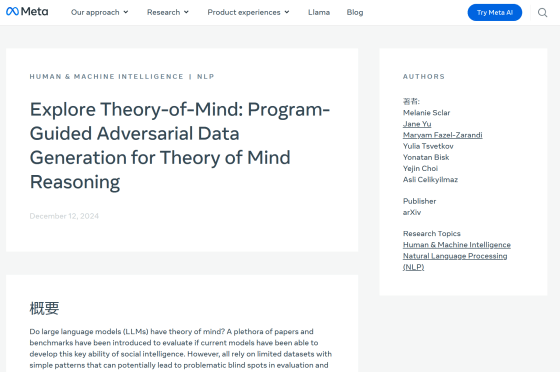
◆Meta Large Concept Models
To realize advanced artificial intelligence, there is a need for AI that excels in the ability to generate long texts that require advanced reasoning and hierarchical thinking, such as writing an essay. Large Concept Models are introduced to enhance hierarchical thinking, and they are said to be able to make predictions that are significantly different from ordinary LLMs.
Large Concept Models: Language Modeling in a Sentence Representation Space | Research - AI at Meta
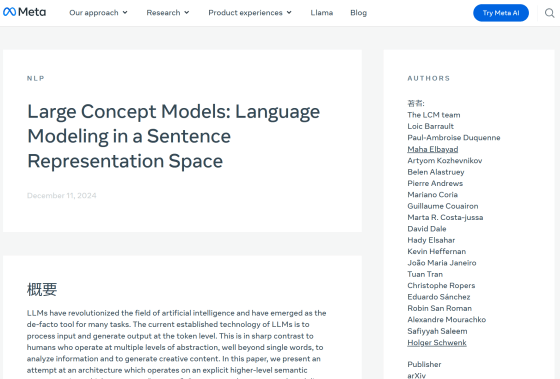
◆Meta Dynamic Byte Latent Transformer
Existing language models assume that words are divided into small pieces for easy processing. Meta says that this is a hurdle to achieving end-to-end learning and that it is difficult to optimize. To address this issue, the Dynamic Byte Latent Transformer, which can process on a byte-by-byte basis, has been introduced, and continuing this research will accelerate the ability to solve problems important to advanced AI, such as low-resource languages.
Byte Latent Transformer: Patches Scale Better Than Tokens | Research - AI at Meta
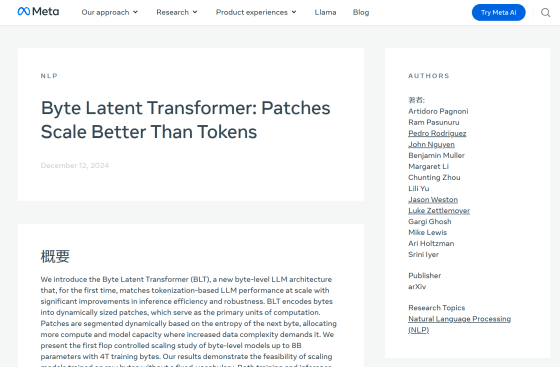
◆Meta Memory Layers
As it has been
Memory Layers at Scale | Research - AI at Meta
https://ai.meta.com/research/publications/memory-layers-at-scale/
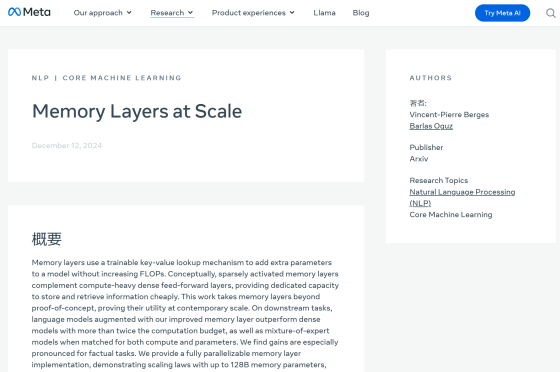
◆Meta Image Diversity Modeling
A comprehensive evaluation toolbox for generative text-to-image models, which will be open-sourced in the future.
EvalGIM: A Library for Evaluating Generative Image Models | Research - AI at Meta
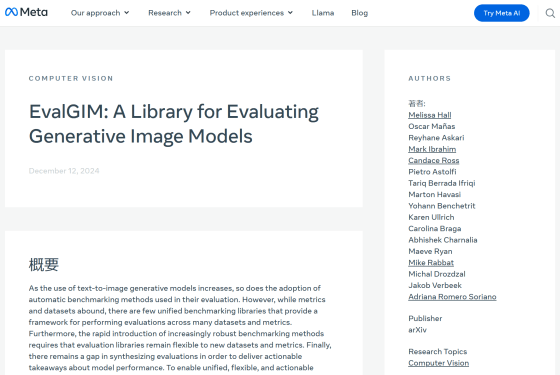
◆Meta CLIP 1.2
Meta CLIP 1.2 is Meta's 'effort to build large-scale, high-quality, and diverse datasets and foundational models.' Meta seeks to develop algorithms that effectively curate data from vast data pools and align them with human knowledge, and to make available foundational models trained on curated datasets to support new research and production use cases.
Meta CLIP 1.2 | Research - AI at Meta
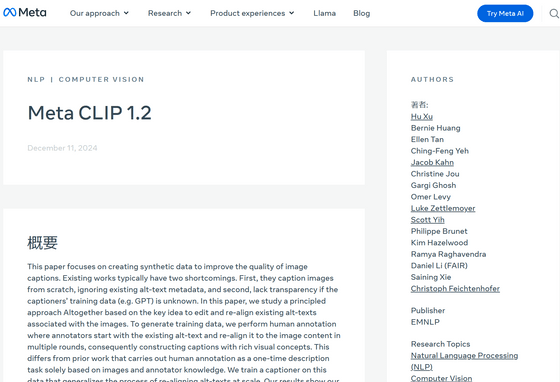
'This work confirms our long-standing track record of sharing open, reproducible science with the community,' said Meta. 'We hope that by publicly sharing our early findings, we can ultimately help advance AI in a responsible way.'
Related Posts:
in Software, Posted by log1p_kr