What is 'AI's self-eating disorder' that causes AI to collapse when learning from data output by AI?
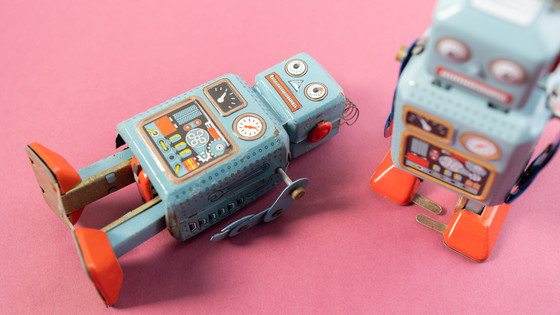
AI training requires huge amounts of data, but the Digital Signal Processing Group at Rice University has announced research results showing that using AI-generated data for this data could have serious negative effects in the future.
Breaking MAD: Generative AI could break the internet | Rice News | News and Media Relations | Rice University
Cannibal AIs Could Risk Digital 'Mad Cow Disease' Without Fresh Data : ScienceAlert
https://www.sciencealert.com/cannibal-ais-could-risk-digital-mad-cow-disease-without-fresh-data
Generative AI such as GPT-4 and Stable Diffusion require huge amounts of data to train, and developers are already running into data supply limitations.
Data needed to develop AI is rapidly depleting, with a quarter of high-quality data becoming unusable in just one year - GIGAZINE
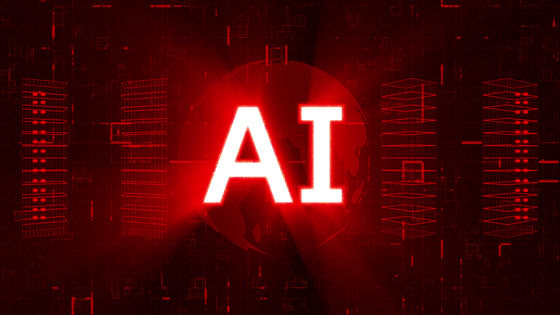
In this context, the use of AI-generated data has emerged as an option, but a research team led by Professor Richard Baranyuk of Rice University warns that this could have dangerous consequences.
The research team focused on the long-term effects of using AI-generated data to train generative AI models. As a result, it was found that as the AI repeats 'self-consuming training' in which it learns from its own generated data, the quality and diversity of the output results gradually declines. The research team has named this phenomenon ' Model Autophagy Disorder (MAD) '.
The research team prepared three patterns: 'Training only with the output results of the previous generation,' 'Training with a dataset that combines the output results of the previous generation and fixed real data,' and 'Training with a dataset that combines the output results of the previous generation and new real data.' We verified MAD by using these patterns.
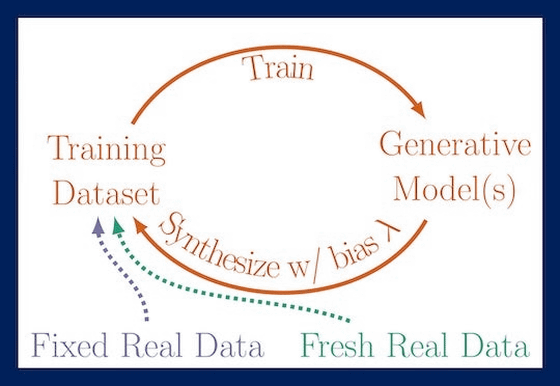
It became clear that in two cases where new real data was not available, the model output gradually became distorted, and the quality and diversity of the output was lost. For example, when generating a human face as shown in the image below, as generations (t) were repeated, noise like lattice scratches appeared on the face.
Next, the research team conducted an experiment to replicate 'cherry-picking,' a process in which high-quality data for self-consumable training is selected from the output of the previous generation of AI.
First, without cherry-picking, each generation of AI was trained on fixed real data and data generated by the previous generation, and the results are shown below. From the fifth generation, the characters began to crumble and lose their original shape, and by the 20th generation, the results looked like mere stains, and the quality rapidly deteriorated. The figures on the bottom row visually show how the distribution of real data (red) and AI-generated data (green) changes over the generations.
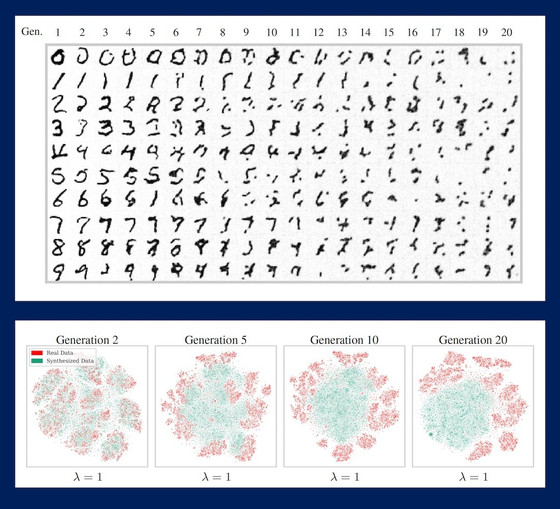
On the other hand, the results of an AI trained on a data set that prioritizes high-quality results through cherry-picking are shown below. High-quality data has been maintained over a long period of time, and even in the 20th generation, most of the numbers are still readable. However, the output results are almost the same for all generations, and it can be seen that diversity has been greatly lost.
'If left unchecked for generations, MAD could undermine data quality and diversity across the Internet,' Professor Baranyuk warned. 'It is inevitable that we will see unintended and unanticipated consequences of AI auto-eating disorder in the near future.'
Related Posts: