Meta releases OpenEQA, a benchmark for AI agents that respond to text-based prompts using visual information
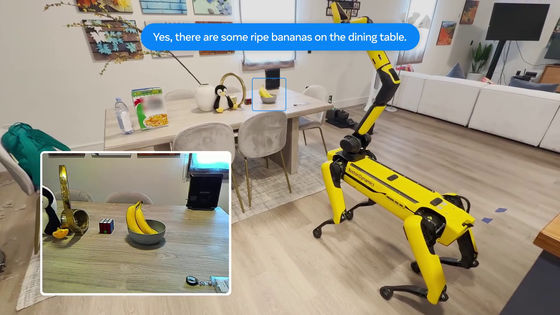
On April 11, 2024 local time, Meta released
OpenEQA: From word models to world models
https://ai.meta.com/blog/openeqa-embodied-question-answering-robotics-ar-glasses/
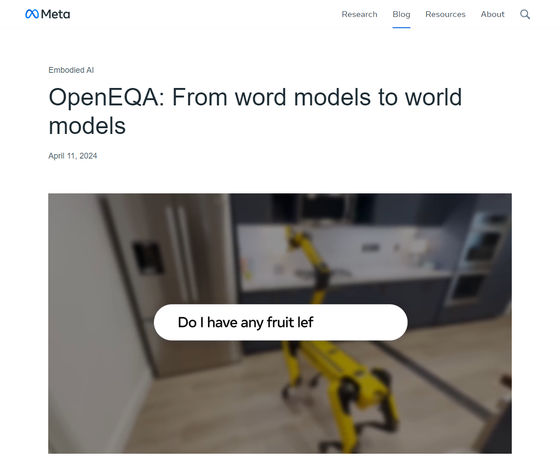
OpenEQA: Embodied Question Answering in the Era of Foundation Models
https://open-eqa.github.io/
Meta AI releases OpenEQA to spur 'embodied intelligence' in artificial agents | VentureBeat
https://venturebeat.com/ai/meta-ai-releases-openeqa-to-spur-embodied-intelligence-in-artificial-agents/
AI agents that act as the brains behind our domestic robots or stylish smart glasses need to be able to understand their surroundings using sensory modalities such as vision and communicate in clear everyday language to effectively assist people. This is like building a ' world model ' - an internal representation of the external world for the agent that can be queried through language. Making this a reality is a long-term vision and a daunting research challenge that Meta is actively pursuing.
As the latest result of this research, Meta has announced a framework called 'Open-Vocabulary Embodied Question Answering (OpenEQA)'. OpenEQA is a new benchmark that measures the understanding of an AI agent by exploring the environment with open vocabulary questions. OpenEQA contains more than 180 environmental datasets, including homes and offices, and more than 1,600 questions about these environments, allowing us to thoroughly verify how well the AI recognizes objects in these environments, how well it can reason spatially and functionally, and how much knowledge it has, such as common sense.
OpenEQA includes two tasks. The first is called 'episodic memory EQA,' in which an embodied AI agent answers questions based on its recollection of past experiences. This task asks whether a question can be answered from past experiences (such as a video recording).
For example, a text-based question might be asked, 'Do you know where I put my badge?'
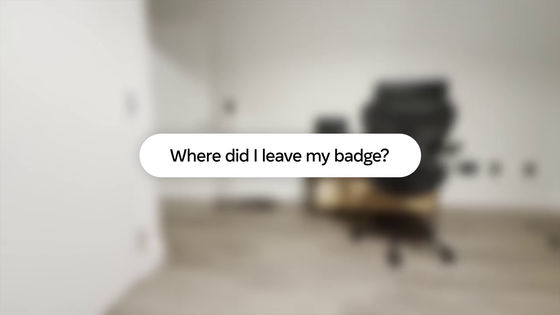
We will verify whether we can derive answers to the questions from previously recorded videos.
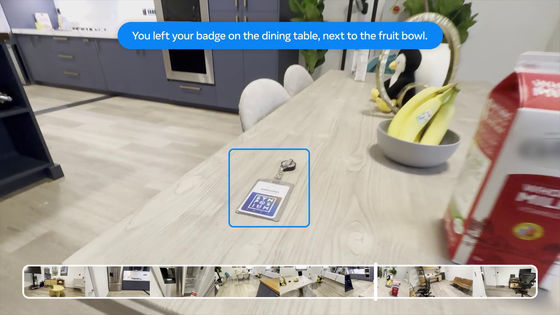
The other is called 'Active EQA,' a task in which the AI agent takes action in the environment to gather the required information and answer a question.
For example, a text-based question like 'Do you have any fruit at home?'
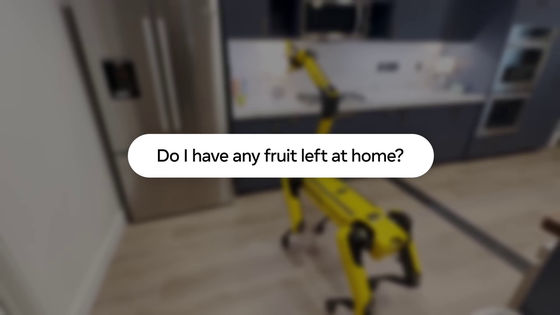
Instead of watching videos you've taken in the past, you move around the house and record videos looking for fruit.
The participants then answer whether or not they found fruit, and their accuracy is verified.
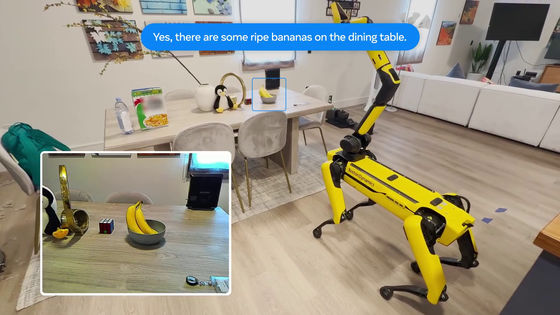
Using OpenEQA, we conducted a benchmark test of an AI model (
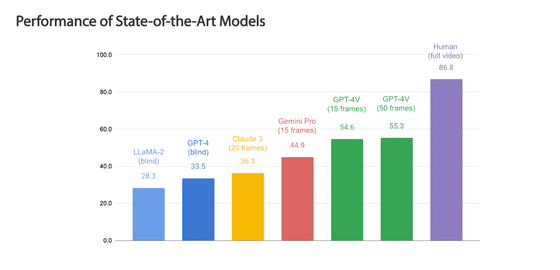
What's particularly interesting is that even the best VLMs are almost 'blindfolded' when it comes to questions that require spatial understanding. Despite being VLMs, the results show that performance is not much different from text-only AI models. Meta points out that this shows that models that use visual information do not benefit substantially from visual information. For example, if you ask, 'I'm sitting on the couch in the living room watching TV. Which room is directly behind me?' the AI model will basically guess the different rooms at random, without the benefit of visual episodic memory that would provide spatial understanding. Therefore, Meta pointed out that 'further improvements are needed in AI models in both perception and inference.'
OpenEQA combines challenging questions that can be vocabulary-free with the ability to answer in natural language. This allows for an easy benchmark that demonstrates deep understanding of the environment, and poses a significant challenge to existing underlying models. Meta wrote, 'We hope that OpenEQA will motivate further research into enabling AI to understand and communicate with the world it sees.'
Related Posts:
in Software, Posted by logu_ii