GPT-3 can demonstrate the ability to surpass college students in the 'analogous' test that finds trends and similarities and solves problems
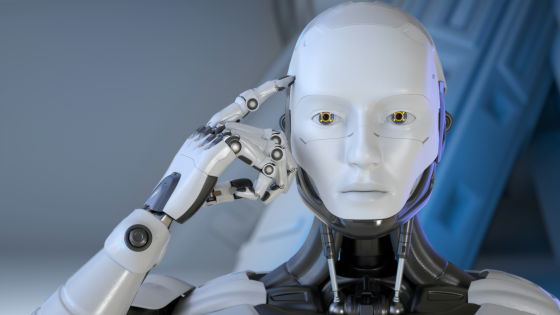
Large
Emergent analogical reasoning in large language models | Nature Human Behavior
http://dx.doi.org/10.1038/s41562-023-01659-w
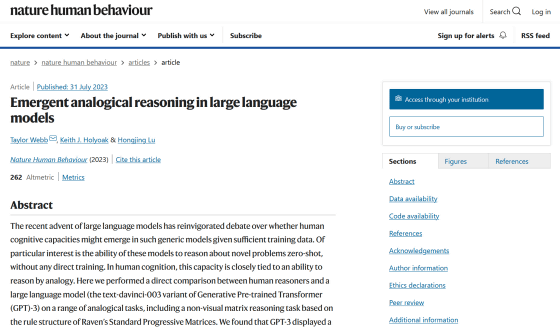
GPT-3 can reason about as well as a college student, psychologists report: But does the technology mimic human reasoning or is it using a fundamentally new cognitive process? -- ScienceDaily
https://www.sciencedaily.com/releases/2023/07/230731110750.htm
GPT-3 aces tests of reasoning by analogy | Ars Technica
https://arstechnica.com/science/2023/07/large-language-models-beat-undergrads-on-tests-of-reasoning-via-analogy/
Analogy is to guess the answer by applying specific information to another thing, and the classic form is 'If 'love-hate' and 'cold-hot', 'wealthy--' What goes in?” In this case, the two words are antonyms, so it can be inferred that the word 'poor' is included in ○○.
There is also a pattern such as ``If ``abcde-abcdf'' and ``fox-foy'' are included in ``earth-○○○○○'', what is included in ○○○○○? In this case, the alphabet at the end of the string is shifted one character backward, so 'earti' will be applicable after 'earth―○○○○○'.
A research team led by Taylor Webb , a postdoctoral researcher at the University of California, Los Angeles, conducted a graphical problem called the `` Raven Progressive Matrix '' on GPT-3 in order to investigate the analogy ability of GPT-3. I had them solve analogy questions in a variety of formats, including questions that were converted to text so that they could be processed, and questions included in the College Aptitude Test (SAT) .
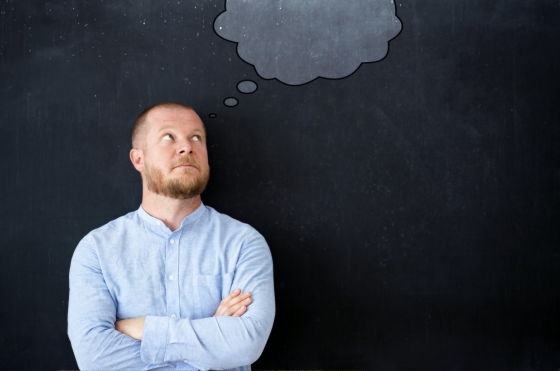
When GPT-3 and UCLA students answered the Raven Progressive Matrix, the average correct answer rate for college students was 60%, while GPT-3 scored 80% higher. bottom. GPT-3 also performed better when comparing SAT scores with those of college applicants. 'These results suggest that GPT-3 has developed an abstract concept of 'successorship' that can be flexibly generalized across different domains,' said the research team.
On the other hand, GPT-3 sometimes fails to recognize when a problem is presented, and has a high error rate when prompts for answers are not included or when questions are given numerically rather than textually. It is said that there was also a problem.
Also, extract the main points of the story, such as ``Read one short story and choose a story with similar content from two options'' and ``Read the lesson from the short story and answer the problem without a solution''. Humans outperformed GPT-3 on the task. GPT-3 also tended to give physically impossible answers in tasks that create physical solutions such as `` how to take out spherical gum using cardboard cores, scissors, tape, etc. ''. This suggests that although GPT-3 can make inferences within a given text, it is unable to draw analogies from knowledge in areas not included in the problem.
Nonetheless, in some preliminary tests using GPT-4, which has improved performance over GPT-3, it performed significantly better than GPT-3. Therefore, in the future, large-scale language models may outperform humans in reading stories.
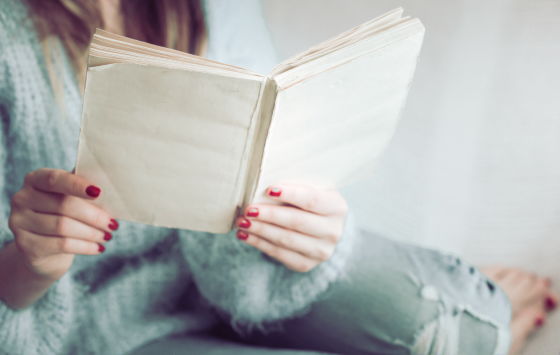
“It is important to stress that large-scale language models have significant limitations, no matter how impressive our results are,” says Webb. It is not possible for humans to do things that are very easy, such as solving problems that are difficult to solve,' he commented.
In the future, the research team plans to investigate whether the large-scale language model actually 'thinks' like a human being, or simply performs a completely different process that mimics human thinking. Keith Hollyoak , co-author of the paper, said, ``GPT-3 may think like humans.However, humans did not learn by ingesting all the content of the Internet, and training The method is completely different between GPT-3 and humans.We want to know whether GPT-3 really thinks in the same way as humans or in a completely new way.'
Related Posts:
in Software, Web Service, Science, Posted by log1h_ik