'Vertex AI', which can be easily developed with less code and introduced into the production environment, is now generally available on Google Cloud, and I actually tried using it.
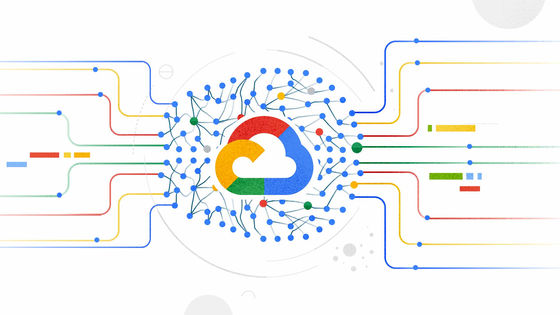
At the online event ' Google I / O 2021 ', Google announced 'Vertex AI ', a machine learning platform that makes it easy to develop and operate AI.
Vertex Ai | Vertex AI | Google Cloud
Google Cloud launches Vertex AI, unified platform for MLOps | Google Cloud Blog
https://cloud.google.com/blog/products/ai-machine-learning/google-cloud-launches-vertex-ai-unified-platform-for-mlops
According to Google, Vertex AI makes it possible to perform machine learning with nearly 80% less code than competitors. Not only does it reduce the number of lines of code, but it also simplifies the process of building a large-scale machine learning model, training, and releasing it to a new model so that users can respond more quickly to changes in the market. Can be released.
Also, in machine learning projects, the difficulty of securing human resources is often taken up as a problem, but with Vertex AI, actions such as data management, prototype creation, experiments, model deployment and interpretation, monitoring in the production environment, etc. are performed. It can be done even by people who have not received machine learning training, and there is no problem even if there is no machine learning engineer. On the contrary, it is possible to import a model tuned by a first-class machine learning engineer, making it a platform for engineers of all levels.
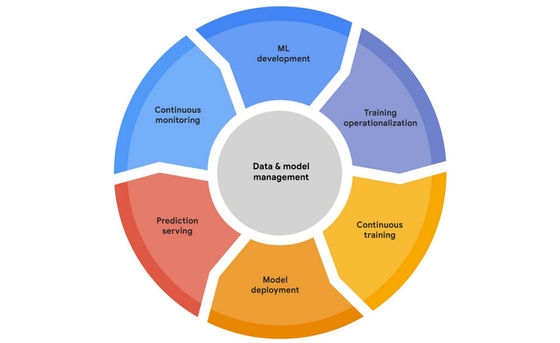
Vertex AI is already available on Google Cloud Platform, and for those who are thinking about using it, '
◆ I actually used it
I happened to have the cutouts and label data of the characters that appeared in the Cygames smartphone game 'Uma Musume Pretty Derby', so I immediately tried the usability.
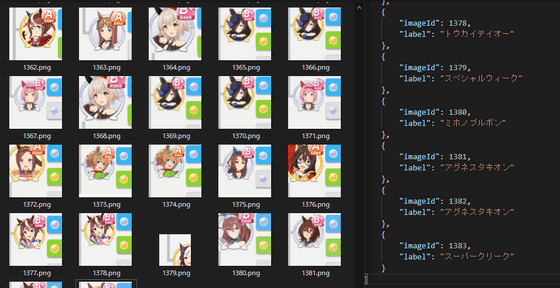
Vertex AI was at the top of the 'Artificial Intelligence' section of the Google Cloud Platform menu. First, open the dashboard, click 'ENABLE VERTEX AI API' to enable Vertex AI, and then proceed to 'Create Dataset'.
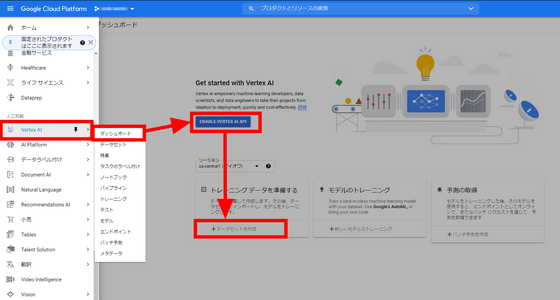
Random numbers were set by default in the dataset name. For the data type and goal, select 'Image classification (single label)' in 'Image' this time. Since the region was either Iowa or the Netherlands, set it to Iowa and click 'Create'.
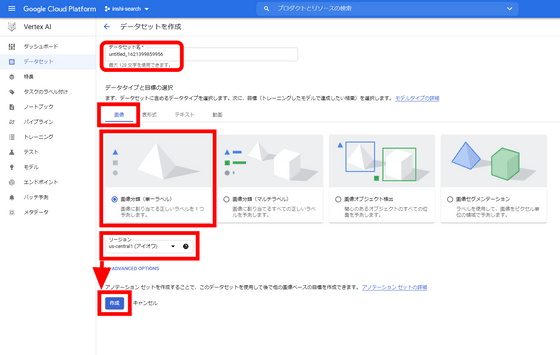
The dataset has been created. You will then be prompted to add teacher data. Since I want to utilize the existing label, I decided to prepare an import file without selecting upload from the computer.
With the method using the import file, it seems that only the images in Cloud Storage can be used, so create a bucket first and upload it.
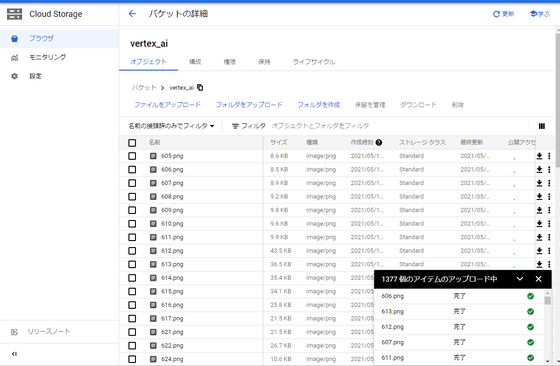
We have also prepared an import file that lists the file paths and labels in CSV format.
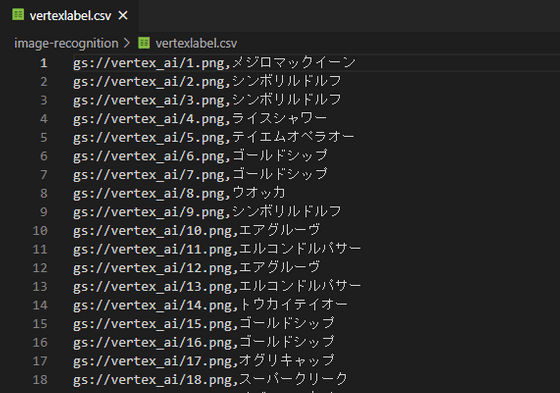
Return to the dataset screen and select 'Upload import file from computer' to upload the prepared import file.
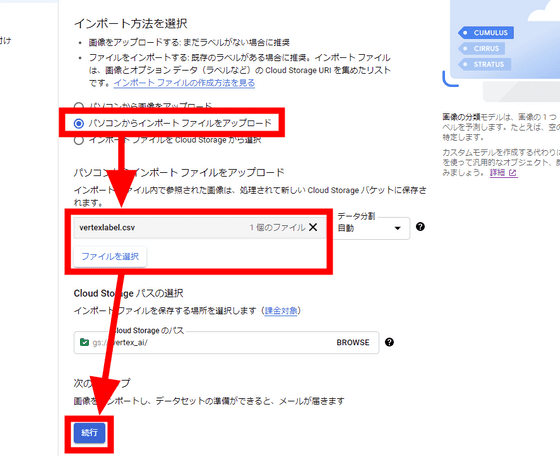
Importing.
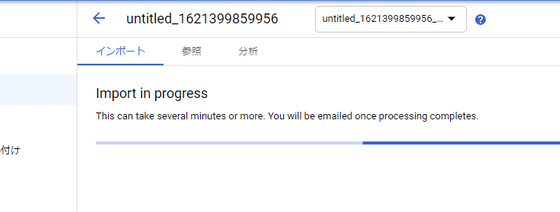
After a while, I received an email saying that the import was completed.
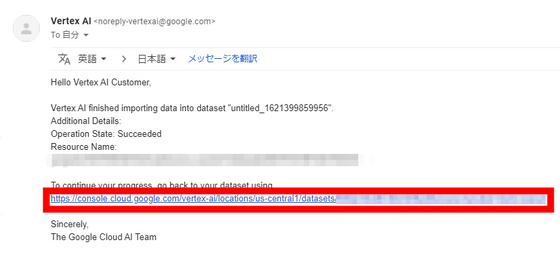
You can see how the image is labeled.
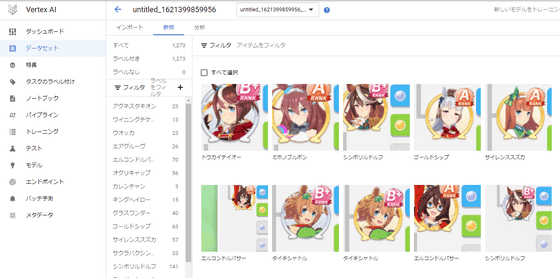
I wanted to train as it is, but when I checked the analysis tab, it was said that 10 or more images were required for each label in order to proceed to training. Image data was not enough for some labels.
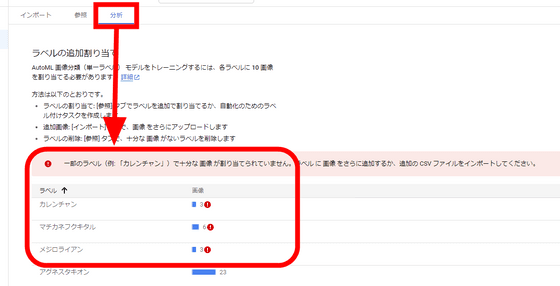
Normally, you should upload additional data, but this time we will remove the label for test review.
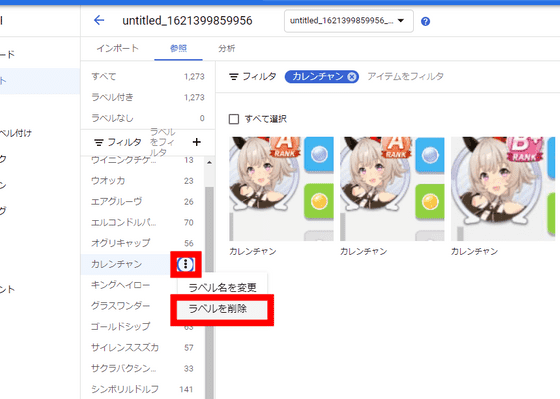
Then, the 'Train new model' button appears. Click.
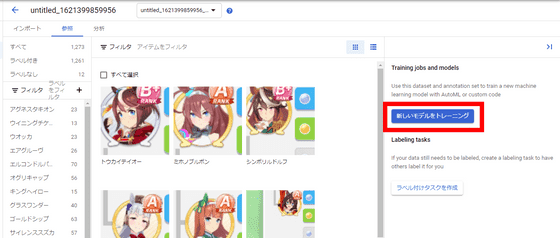
You can change the training settings, but this time we will use all the default values.
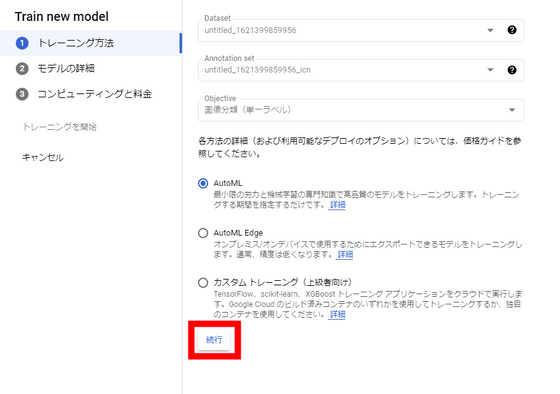
No particular changes to the model details.
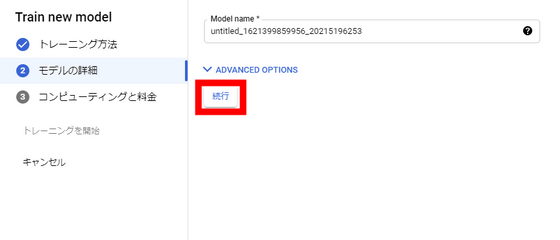
Compute and Pricing allows you to set a budget for training your model. Since the minimum 8 node time was the maximum 800 node time, this time we will set the lowest 8 node time.
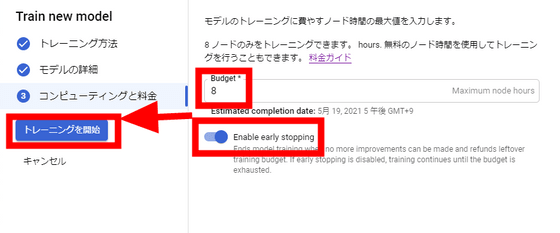
You can check the training status on the 'Training' tab. This time, the training was completed in 23 minutes and 39 seconds. Click the model name to see the details.
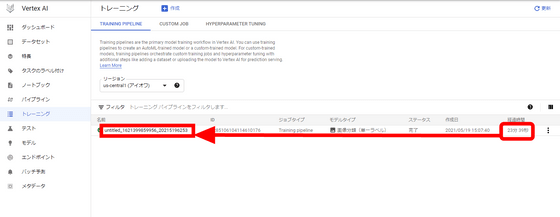
The detail screen looks like this. You can see various indicators of the model.
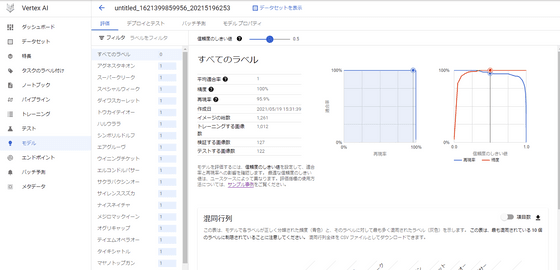
By clicking on the label, I was able to individually check what kind of data I made a mistake.
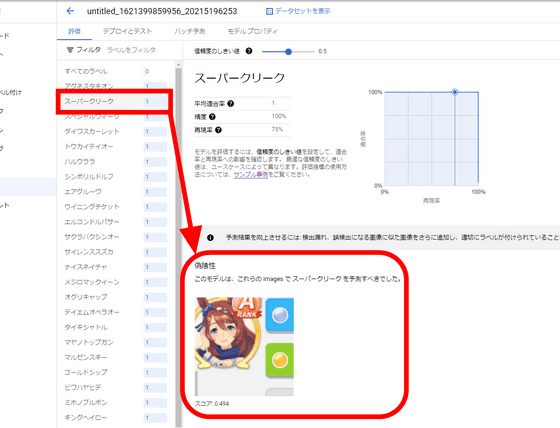
Click 'Deploy to Endpoint' on the 'Deploy and Test' tab, set the name and number of nodes, and click 'Deploy' to complete the deployment.
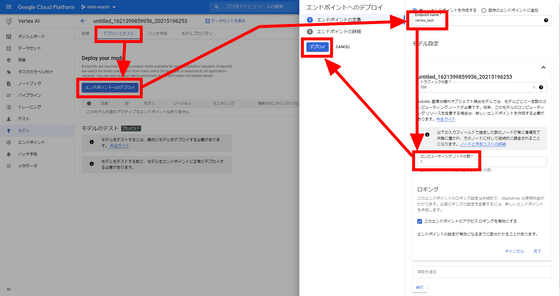
After that, just send the image data to the endpoint and AI will classify the image. After all, this time I was able to create a classification AI with a certain degree of accuracy without writing a single line of code. Even if you don't have any knowledge about machine learning, you can do something by clicking a button, so the threshold for 'doing something with machine learning' is considerably lower.
In Vertex AI, you can save the flow of 'training and deploying the model with the uploaded data' as a pipeline, and set it so that the model is automatically updated every time the data is updated. It seems that there is no doubt that it is a fairly high-performance platform, such as being able to use it and being able to use your own custom model in addition to the prepared models.
Related Posts:
in Review, Web Service, Web Application, Posted by log1d_ts