A system for predicting the three-dimensional structure of proteins has made great progress by machine learning
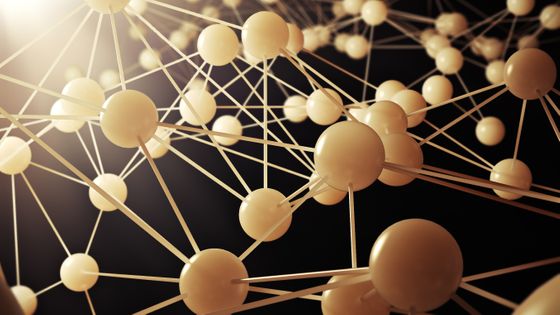
by
Proteins are large molecules formed by linking a large number of 22 amino acids in a chain, and are deeply involved in intracellular chemical and mechanical processes. It is said that the development of a system to understand the three-dimensional structure of such proteins has been greatly advanced by machine learning.
Improved protein structure prediction using potentials from deep learning | Nature
https://www.nature.com/articles/s41586-019-1923-7
A watershed moment for protein structure prediction
https://www.nature.com/articles/d41586-019-03951-0
The phenomenon that a protein folds into a specific three-dimensional structure is called folding, and it is important to understand the three-dimensional structure of a protein in order to understand the behavior of each protein. However, most proteins only know the sequence information of the constituent amino acids, and it is said that most proteins do not know the actual three-dimensional structure.
Since determining the three-dimensional structure of a protein by experiment requires a huge amount of time and cost, developing a technology for predicting the three-dimensional structure of a protein is an important research topic. However, the three-dimensional structure of proteins has very diverse patterns, and the problem is that the larger the protein structure, the greater the interaction between amino acids and the more difficult it is to model the structure. If we can calculate the exact energy of a protein molecule using quantum theory, we can predict the most energetically favorable structure, but we cannot perform quantum calculations on proteins using existing computers.
Also, the three-dimensional pattern that a protein can take is an astronomical figure, and even if you try to determine the correct three-dimensional structure of a protein brute force, the life of the universe will expire before calculating all the patterns. thing. This issue is called ' Levinsol's paradox ' from the name of the advocate, molecular biologist Cyrus Levinsol .
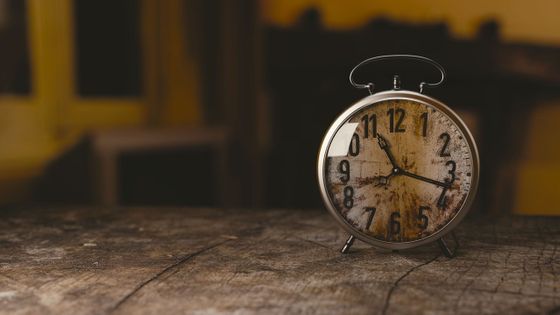
by
Researchers have been developing algorithms to predict the three-dimensional structure of proteins from their amino acid sequences to solve these problems. This and methods to adapt the three-dimensional structure of the protein to the terrain before, amino acid residue such Prediction mutation is based on the co-evolution information such cause mutation of the corresponding amino acid residues, many techniques have been developed Was. In particular, predictions based on co-evolution information using amino acid residues have made great progress because available amino acid sequence data has rapidly increased in the 2010s.
Meanwhile, DeepMind , an artificial intelligence developer under Google, has developed an algorithm called AlphaFold that predicts the three-dimensional structure of proteins. AlphaFold is an algorithm that predicts the three-dimensional structure of a protein using machine learning. First, it predicts the distance and angle taken by a pair of amino acid residues. Mathematically, the researchers say that the relative position can be determined by the position between each amino acid residue, so by predicting the distance between amino acid residues, the three-dimensional structure of the protein can be specified.
In addition, AlphaFold has continuously improved the accuracy of protein 3D structure prediction using the gradient descent method (steepest descent method) . As a result, AlphaFold achieved the highest accuracy and won the first place in the “ International Contest of Protein 3D Structure Prediction (CASP13) ” held in 2018, in which 207 teams participated.
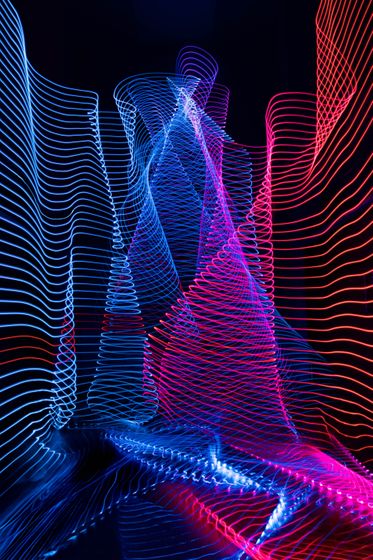
by Zak Sakata
In CASP13, AlphaFold's prediction was only 0.66 nanometers (6.6 angstroms ) from the correct structure. However, AlphaFold is still not applicable to applications that require 2-3 Å resolution, such as elucidation of how drugs bind to proteins and elucidation of the catalytic mechanism of enzymes. Another problem is that it takes tens to hundreds of hours to specify the three-dimensional structure of one protein.
Nevertheless, it is said that the protein three-dimensional structure can be predicted with a resolution of 4 angstroms by 2025, which is a very significant advancement when considering the three-dimensional structure of proteins by cryo-electron microscopy, etc. It can be said. The team said that the emergence of a method that uses machine learning to predict the three-dimensional structure of proteins could be a major turning point in changing the life sciences.
Related Posts:
in Science, Posted by log1h_ik