How is the AI 'AlphaFold' that predicts the three-dimensional structure of proteins changing the world of biology?
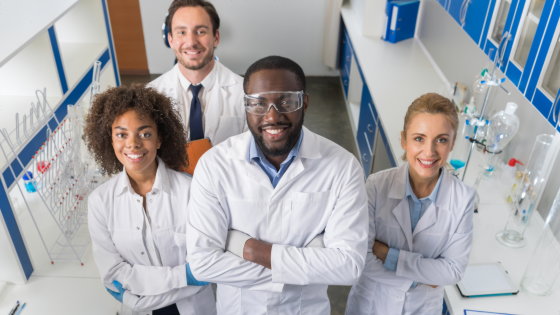
In 2018,
What's next for AlphaFold and the AI protein-folding revolution
https://www.nature.com/articles/d41586-022-00997-5
Proteins are substances involved in almost all biological processes such as muscle contraction, blood transport, light sensing, and food energy transformation. Such a protein is a three-dimensional polymer compound in which a large number of 20 types of L-amino acids are linked in a chain, and how amino acid units called amino acid residues are connected can be understood from one-dimensional sequence information. I only know.
Although more than 200 million proteins have been discovered by humans, most of them have only known amino acid sequences, and few have identified the three-dimensional structure of proteins. Since the three-dimensional structure of a protein is closely related to its behavior and function, inferring the three-dimensional structure from an amino acid sequence is called the 'protein folding problem ' and has been a major biological problem for many years. ..
In previous studies, experimental methods such as cryo-electron microscopy , nuclear magnetic resonance , and X-ray crystallography have been used to clarify the three-dimensional structure of proteins, but this is time-consuming and costly. Therefore, in recent years, it has been expected that AI will solve the folding problem. Developed by DeepMind in 2018, AlphaFold won the 2018 International Protein Structure Prediction Contest (CASP), and the latest version of AlphaFold at 2020 CASP is at the same level as the experimental method. It is said that it attracted more attention by recording the accuracy .
Before AlphaFold became open source, researchers developed a unique AI tool called ' RoseTTAFold ' based on lectures by John Jumper and others who lead DeepMind's AlphaFold team. Finally, in July 2021, AlphaFold was released as open source, making AlphaFold widely available to researchers.
Artificial intelligence company DeepMind releases protein structure analysis algorithm 'AlphaFold' as open source, making it available to anyone --GIGAZINE
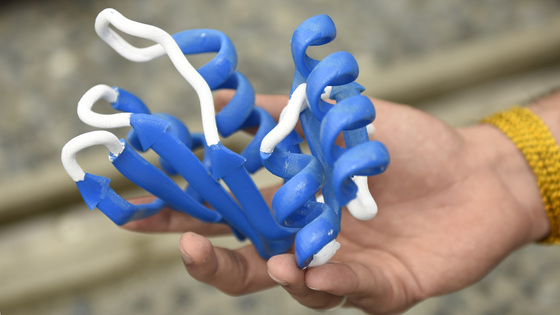
by OIST
'AlphaFold changes the game. It's like an earthquake. You can see its effects everywhere,' said Ora Schueler-Furman, a protein researcher at the Hebrew University of Jerusalem. .. 'At every conference I attend, people are saying,'Why don't you try AlphaFold,'' said Christine Orengo, a computational biologist at University College London. ..
In fact, there are ongoing attempts to apply AlphaFold to protein-related research. A research team led by Martin Beck, a molecular biologist at the Max Planck Institute for Biophysics in Germany, has learned about the nuclear pore complex through which substances enter and exit the cell's nucleus and the protein family called nucleoporin that composes it. In a 2016 study , we published a model that covers about 30% of the nuclear pore complex. After that, when the model was adjusted using AlphaFold, which was made open source in 2021, it was possible to announce a model that covers about 60% of the nuclear pore complex in October 2021.
DeepMind also plans to publish a total of more than 100 million protein three-dimensional structure predictions by 2022. The number of 100 million is about half of the known proteins, and it is said that it is hundreds of times as many as the proteins whose three-dimensional structure has been identified by the experimental method contained in the structural repository of the Protein Data Bank (PDB).
The graph below shows the number of 'papers using AlphaFold' published by researchers, with a light orange color published in a scientific journal and a dark orange color uploaded to a preprint server. Is shown. It can be seen that the number of papers has increased sharply since AlphaFold became open source in July 2021.
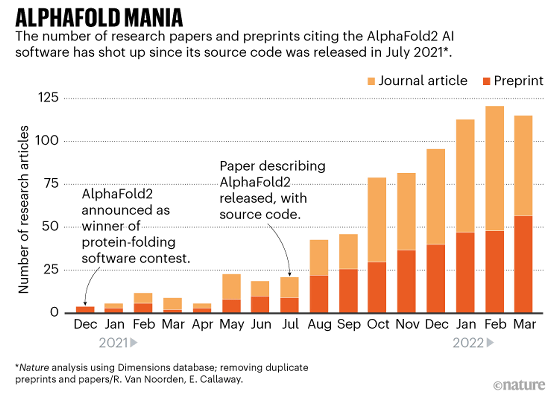
AlphaFold is trained on experimentally identified protein data from PBDs and other databases. Given the new amino acid sequence, AlphaFold first looks for related sequences in the database to identify amino acids that tend to have similar conformations. The structure of existing related proteins also helps to estimate the distance between amino acids in the new amino acid sequence. Based on these various clues, AlphaFold predicts the three-dimensional structure of proteins.
According to DeepMind, more than 400,000 people have accessed the AlphaFold database managed by the
Many biologists are impressed with the accuracy of AlphaFold. Thomas Boesen, a structural biologist at Aarhus University in Denmark, has conducted a test using AlphaFold to predict the three-dimensional structure of proteins that have not been made public yet, although his research team has elucidated the three-dimensional structure experimentally. It was said that. As a result, AlphaFold was able to accurately predict the three-dimensional structure, 'This is a big verification from my side.' 'I have a lot of trust in AlphaFold based on what I saw.' Boesen said.
It is also expected to be useful for research on the evolution of proteins and the origin of life by applying the mechanism of predicting the three-dimensional structure from the gene sequence of AlphaFold proteins. Researchers usually compare gene sequences to determine how the genes of an organism are related between species, but for genes with fairly old relationships, the sequence changes are too great for both. It may be difficult to see the relationship. However, by comparing protein structures that change slower than gene sequences, it may be possible to discover old relationships that have been overlooked so far. 'This opens up a great opportunity to study the evolution of proteins and the origin of life,' said Pedro Beltrao, a computational biologist at the Swiss Federal Institute of Technology.
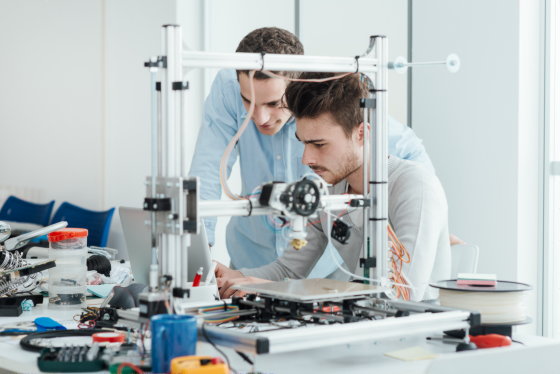
On the other hand, AlphaFold is not an immediate solution for researchers who want to understand the detailed three-dimensional structure of a specific protein, and ultimately requires an experimental decision. However, AlphaFold's three-dimensional structure prediction is an approximate value that is useful when interpreting data obtained by experimental methods, and it is said that it has led to speeding up research. '(AlphaFold) has completely changed the focus of our research,' said Randy Read, a structural biologist at the University of Cambridge, saying that combining X-ray crystallography data with AlphaFold changed the approach. Said.
AlphaFold was also designed to predict the shape of a single peptide chain, but just days after the open source of AlphaFold, a protein researcher at the University of Tokyo,
AlphaFold2 can also predict heterocomplexes. All you have to do is input the two sequences you want to predict and connect them with a long linker. Pic.twitter.com/BhmWcnlQed
— Yoshitaka Moriwaki (@Ag_smith) July 19, 2021
Of course, AlphaFold can't always predict the exact 3D structure, and it also has the ability to label the reliability of the prediction. The following three three-dimensional structure prediction diagrams are for 'Good' when the three-dimensional structure prediction is successful, when 'Bad' is not so successful, and when 'Ugly' is almost unpredictable. As for the color coding of the three-dimensional structure, purple is the three-dimensional structure existing in PBD, blue is the highly reliable three-dimensional structure, light blue is the highly reliable three-dimensional structure, yellow is the unreliable three-dimensional structure, and orange is the fairly reliable three-dimensional structure. Shows a low three-dimensional structure. It can be seen that the less reliable the three-dimensional structure prediction, the more chaotic spaghetti-like shapes, and the more yellow and orange parts.
One of the limitations of AlphaFold is that it is difficult to predict the effect of mutations on the conformation because it relies on existing protein information in the database. It is also difficult for AlphaFold to predict how proteins will change shape due to the presence of other interacting proteins or molecules such as drugs.
Bryan Roth, a structural biologist at the University of North Carolina at Chapel Hill, says AlphaFold did make accurate predictions about about half of the proteins called G protein-coupled receptors , saving research time, but the rest. He pointed out that half did not help. In addition, it seems that there were cases where prediction failed even if the reliability of labeling was quite high. Roth, a drug discovery researcher, wonders how useful AlphaFold can be in drug discovery research, as it may not be possible to predict the three-dimensional structure when it binds to a ligand or drug.
Although there are still problems with AlphaFold, it is expected that research using AlphaFold will continue to accelerate and various discoveries will be made. 'Things are changing rapidly, and we'll see a really big breakthrough with AlphaFold next year,' said David Baker, a biochemist at the University of Washington. Janet Thornton, a computational biologist at the European Molecular Biology Laboratory, said that one of the biggest impacts of AlphaFold has prompted biologists to change their insights from a computational and theoretical approach. Claims to be.
Jan Kosinski, a structural biologist at the European Molecular Biology Laboratory, said AlphaFold-inspired tools will enable modeling of individual proteins and complexes, as well as whole cell organs and protein molecules. Imagine. “This is a dream we will pursue over the next few decades,” said Kosinski.
Related Posts:
in Science, Posted by log1h_ik