Technology that allows you to convert photos to animated characters appeared
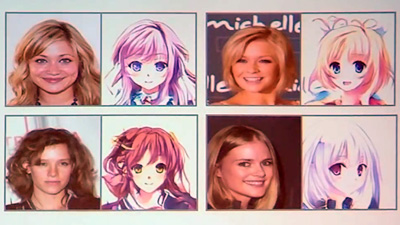
A method of creating an animated character from a person's photo using an algorithm called GAN (hostile generation network) is explained in Tokyo Deep Learning Workshop 2018, and the video has been uploaded to YouTube.
25. Yanghua Jin: Creating Anime Characters with GAN - YouTube
GAN is a mechanism that combines two neural networks and raises the accuracy of generating specific data by competing with each other. Ian Goodfellow, who invented GAN, explained GAN as counterfeiting of a police attempting to distinguish counterfeit from counterfeiters trying to make counterfeit notes. In other words, one neural network attempts to trick the "police" by making real counterfeit bill data, and the neural network on the "police" side attempts to find counterfeit data from the entered data.
By using this mechanism, you get a "counterfeit" neural network that generates realistic data. By using the real-life data generated by this "counterfeit" neural network, we can reduce the number of original teacher data necessary to train the neural network.
The cases of this GAN is being used, "to automatically generate a character in artificial intelligence (AI) MakeGirls.Moe " or "colored with automatic line drawings of illustrations PaintsChainer are present, such as", each service It is easy to understand what kind of things you read by reading the following article.
Artificial intelligence automatically generates beautiful girl characters "MakeGirls.moe" - GIGAZINE
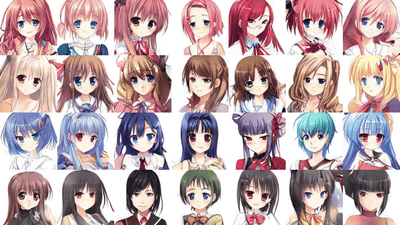
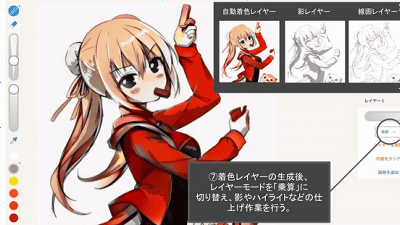
Yanghua Jin who entered Tokyo Deep Learning Workshop 2018 said that he thought "You can not create animated characters from photos." I tried to convert photos to animated characters by machine learning, using the image shown below as searching "anime vs reality" on Google as teacher data. The illustration below is an illustration of figures on the left with figures on the right and illustrations on figures on the right based on portraits, but the aim is to automatically generate the right image from this left photo.
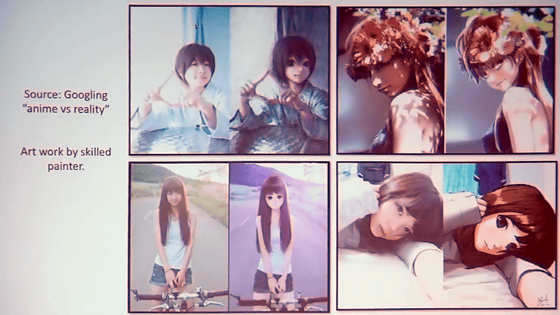
One of the technologies for that is called CycleGAN, which is a combination of two GANs. Below is an image extracted from the paper of CycleGAN (PDF), but I will briefly explain it using this.
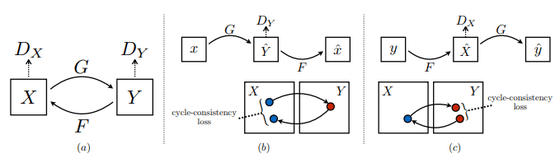
For example, if X is English and Y is Japanese, G is a neural network that translates English into Japanese. If you write Japanese translated through G as G (X), DY is a neural network that distinguishes between Y and G (X), which is automatically translated from Japanese or English written by native. By improving G based on feedback from DY, the quality of the translated Japanese G (X) approaches Y. This is the structure of GAN.
CycleGAN prepares a contrasting shape by preparing a neural network in the opposite direction from English → Japanese plus Japanese → English. And when we translate backwards like English → Japanese → English, Japanese → English → Japanese, we adjust it so that the output will be the same. By doing so, you can create a neural network that can freely move X and Y back and forth.
Here, I mentioned translation as an example, CycleGAN is actually an active technique in image processing, changing the style of the painter, changing the zebra to a horse, replacing the summer landscape with the winter landscape The research results to be published are published.
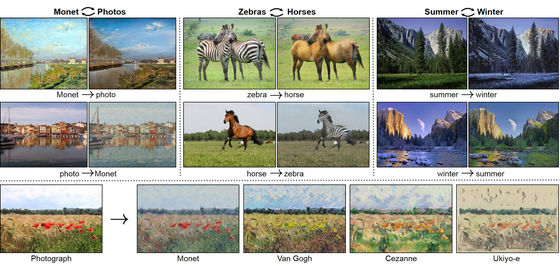
Also, the movie below is one of CycleGAN's deliverables. You can see that you can convert a horse appearing in a movie to a zebra.
Turning a horse video into a zebra video (by CycleGAN) - YouTube
However, while CycleGAN can perform "re-texturing" very well, it is not good to "conduct transformation that changes shape from the original image". In other words, when creating an animated image from a facial photograph, further ingenuity is necessary to convert such as enlarging eyes and reducing mouth and nose.
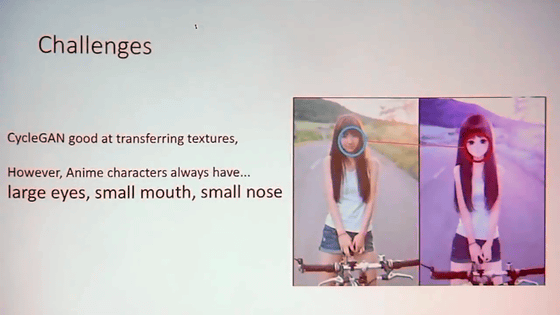
Yanghua Jin said that it succeeded in creating a network that can successfully perform the above conversion by introducing a technique called Stacking to the neural network model.
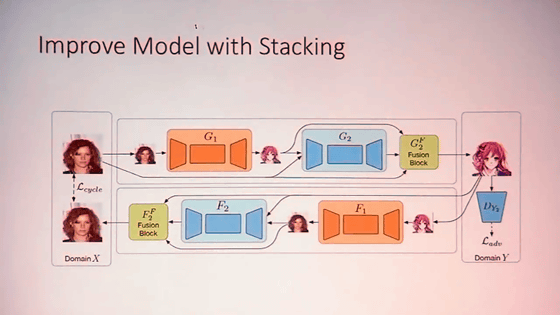
The image below is converted by Yanghua Jin's neural network. The left is an input animation character with right generated by the photo used for input. You can see that various apparent characters are generated with high resolution while capturing features of individuals such as facial expressions, facial angles, and hairstyle.
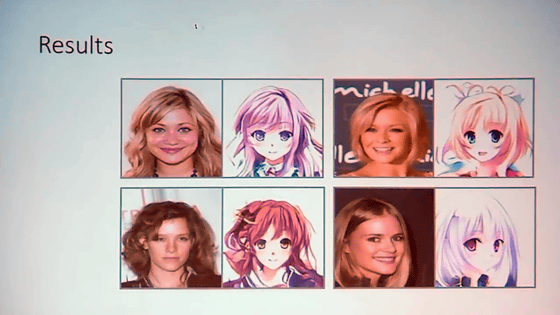
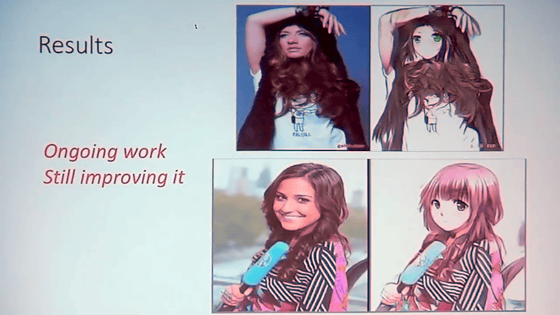
In recent years, virtual YouTuber has exceeded 3000 people, and live-action persons are becoming animated characters, such as being able to FaceTime using iOS 12 announced at WWDC 2018 using anime characters In the situation, Yanghua Jin's technology made us feel the possibility of repainting the industry chart.
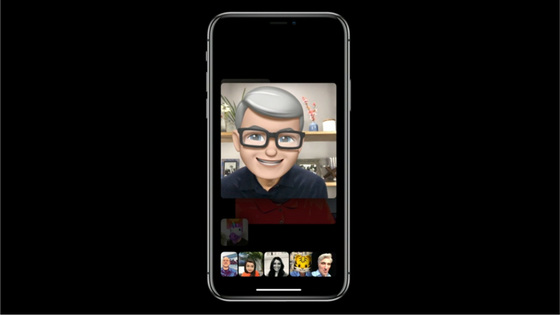
Related Posts: