Google researchers claim that the processing order of large-scale language models is similar to neural activity in the human brain
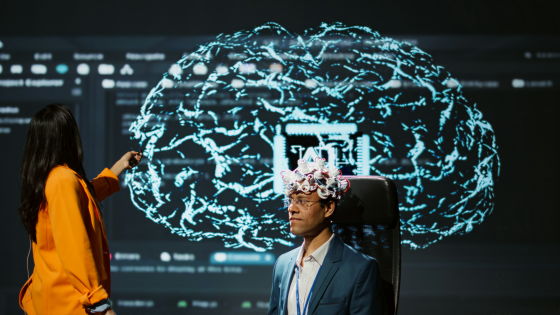
Recent chat AI based on large-scale language models can hold real-time conversations with accuracy comparable to that of humans, but its computational framework is completely different from that of the human brain. However, in a blog post in March 2025, Google researchers claimed that there is a remarkable similarity between
Deciphering language processing in the human brain through LLM representations
https://research.google/blog/deciphering-language-processing-in-the-human-brain-through-llm-representations/
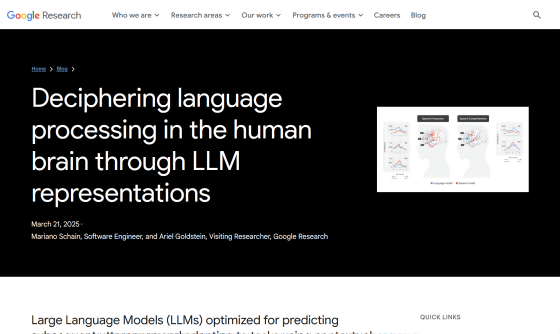
Although large-scale language models can process natural language with high accuracy, in theory they have a computational framework that is fundamentally different from the human brain. Large-scale language models do not rely on symbolic parts of speech or syntactic rules, but instead rely on machine learning to predict and generate next words, which allows them to generate context-sensitive words from real-world text corpora.
Inspired by the success of large-scale language models, a research team at Google Research has been investigating the similarities and differences between the human brain and large-scale language models in natural language processing. In a paper published in the academic journal Nature Human Behavior in March 2025, Google researchers used electrodes placed inside the subjects' skulls to analyze neural activity patterns in the brain during conversations and compared this with the internal embeddings generated by the speech-to-text model of OpenAI's transcription AI Whisper .
From the Whisper speech-to-text model, two types of embeddings were extracted for speech understanding and text generation: 'speech embeddings from the speech encoder' and 'word-based language embeddings from the decoder.' In addition, a linear transformation was estimated to predict the brain's neural signals from the speech-to-text embeddings of each word.
The results of the study revealed a remarkable consistency between the speech areas of the human brain and the speech embeddings of the large-scale language model, and between the language areas of the brain and the language embeddings of the large-scale language model. For example, when a subject hears the audio 'How are you doing?', cortical activity is generated in the speech areas of the brain.
A few hundred milliseconds later, as subjects begin to comprehend the meaning of the words, cortical activity arises in language areas of the brain that the researchers claim can be predicted by the speech and language embeddings of the large-scale language model.
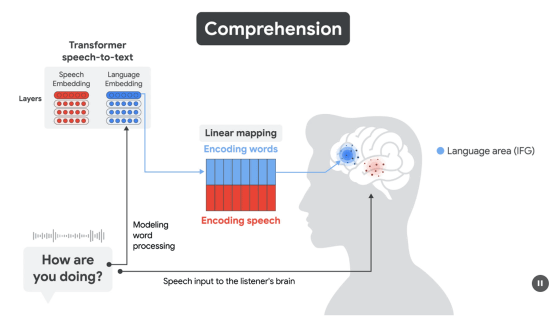
A similar phenomenon occurs when subjects try to speak: When they try to say 'feeling fantastic!', cortical activity in the language areas of the brain occurs about 500 milliseconds before the speech begins.
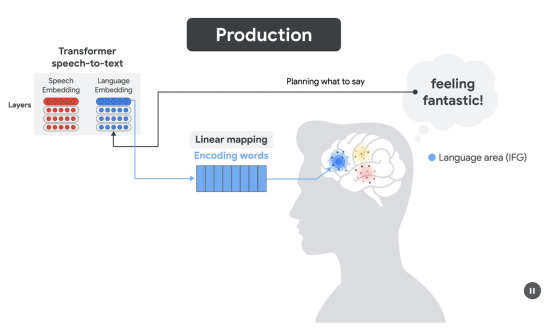
Then, when the subject produces words, brain activity occurs in the motor areas of the brain.
Finally, after the word is spoken, neural activity in the auditory cortex occurs when the word is heard. These neural activity patterns in the brain can also be predicted from the internal embedding of the large-scale language model.
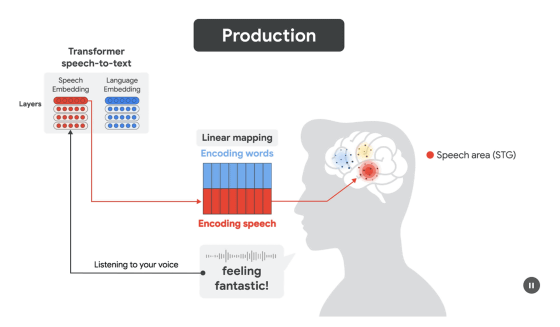
In addition, the figure below shows language embeddings and their corresponding brain regions in blue, and speech embeddings and their corresponding brain regions in red. The graph on the right shows the prediction accuracy of neural activity in each brain region, with the dotted line in the middle representing the 'moment when speech is heard.' The top is
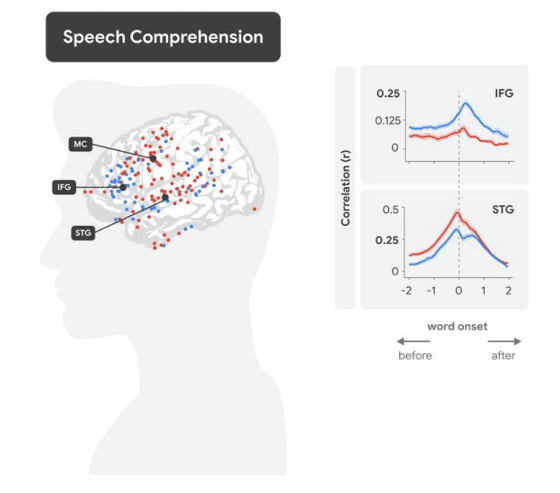
In the figure below, the dotted line in the center of the graph indicates the moment when a word is spoken, and the graph in the middle shows the prediction of neural activity in
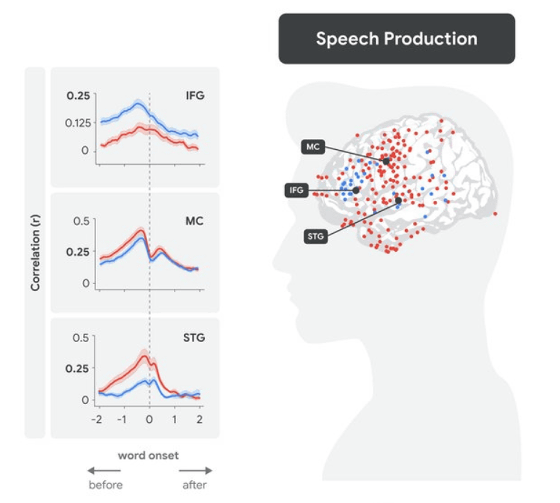
'Overall, our findings suggest that speech-to-text model embeddings provide a cohesive framework for understanding the neural underpinnings of language processing during natural conversations,' the researchers said.
The announcement has also been a hot topic on the social news site Hacker News.
Deciphering language processing in the human brain through LLM representations | Hacker News
A user named namaria pointed out that the paper on which the announcement was based had a small number of subjects, and that the research, which geometrically interpreted neural activity and sensor data, was unnatural, so while the announcement was interesting, it is problematic to take it at face value.
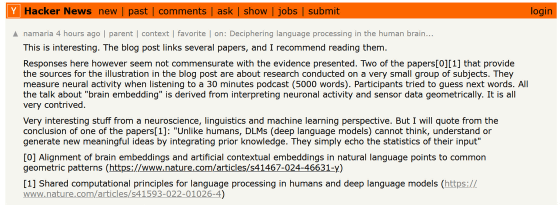
Related Posts:
in Software, Web Service, Science, Posted by log1h_ik