Finally, data for AI training is running out, and AI companies lacking datasets will likely be forced to move from large, general-purpose LLMs to smaller, more specialized models.
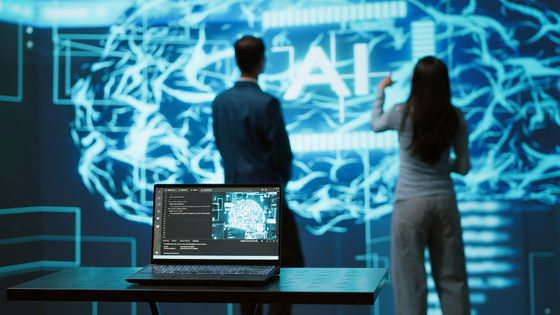
A dataset is essential for developing an AI model as learning material, but large-scale models are already eating up most of the data they can access, and it has been pointed out that they may run out of data by 2028. The academic journal Nature summarizes the current state of AI and datasets.
The AI revolution is running out of data. What can researchers do?
Synthetic data has its limits — why human-sourced data can help prevent AI model collapse | VentureBeat
https://venturebeat.com/ai/synthetic-data-has-its-limits-why-human-sourced-data-can-help-prevent-ai-model-collapse/
OpenAI cofounder Ilya Sutskever predicts the end of AI pre-training - The Verge
https://www.theverge.com/2024/12/13/24320811/what-ilya-sutskever-sees-openai-model-data-training
AI has seen explosive growth over the past decade, particularly in its ability to analyze human text and return plausible sentences. However, all of this ability has been built by learning from a variety of data, including existing texts on the internet.
There's no doubt that there's a huge amount of data on the internet, but according to research institute Epoch AI, AI is learning from that data at an astonishing rate and could potentially eat up most of it.
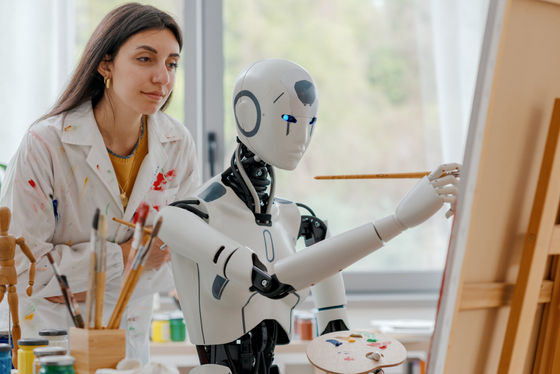
Epoch AI predicts that 'by 2028, the size of the datasets used to train AI models will be equal to the total stock of text on the Internet.' This means that AI will likely run out of training data by around 2028. In addition to the scarcity of datasets, another hurdle for AI researchers is that data owners such as newspapers are beginning to crack down on content use, making access even more difficult.
These problems are well known among AI researchers, and there is a theory that the scaling of learning approaches its limits as traditional data sets run out. To compensate for the lack of data sets, AI researchers are exploring ways to improve AI performance, such as by changing the learning method.
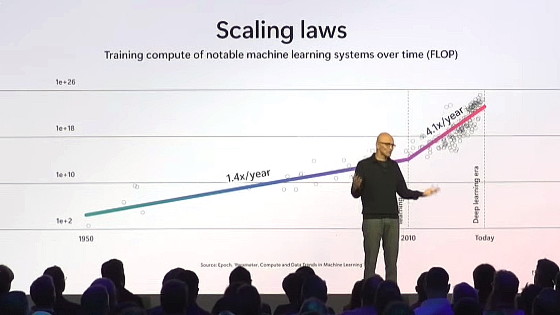
For example, prominent AI companies like OpenAI and Anthropic have publicly acknowledged the problem of lack of datasets, but have suggested they have plans to get around it, such as generating new data or finding unconventional data sources. An OpenAI spokesperson said, 'We use many sources of data, including public data, private data from partnerships, synthetic data from our generation, and data from our AI trainers.'
'Eventually, we will be forced to shift the way existing models learn,' said Ilya Satskivar, who left OpenAI to start Safe Superintelligence, a company working to improve AI safety. 'Next-generation models will be able to solve problems incrementally in a way that is more like 'thinking,' rather than pattern-matching based on what they've seen before.'
A lack of datasets could prevent AI from incorporating new information, slowing down AI progress, but ingesting AI-generated data raises questions about the reliability of the data.
'Although various workarounds are being explored, the lack of data may nonetheless force changes in AI models, perhaps shifting the situation away from large, all-purpose LLMs towards smaller, more specialised models,' Nature noted.
It is possible to scale up the computational power and number of parameters of a model without scaling up the data set, but this tends to slow down the AI processing speed and increase costs. One option is to train specialized data sets, such as astronomy or genomic data, which are rapidly increasing with the advancement of AI, but this may only produce models that are extremely specialized.
'Some models can already be trained to some extent on unlabeled videos and images. Expanding and improving their ability to learn from such data could open the door to richer data,' Nature said.
Related Posts:
in Software, Posted by log1p_kr