Who are the 'three visionaries who pursued unconventional ideas' that drove the deep learning boom that led to the development of AI?
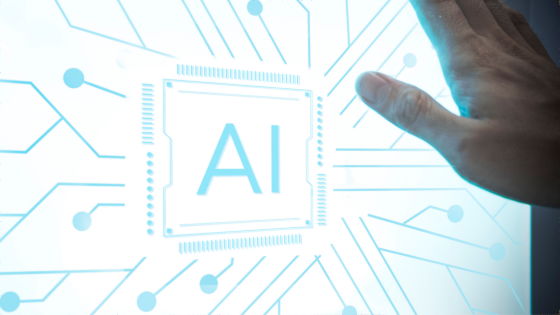
Why the deep learning boom caught almost everyone by surprise
https://www.understandingai.org/p/why-the-deep-learning-boom-caught
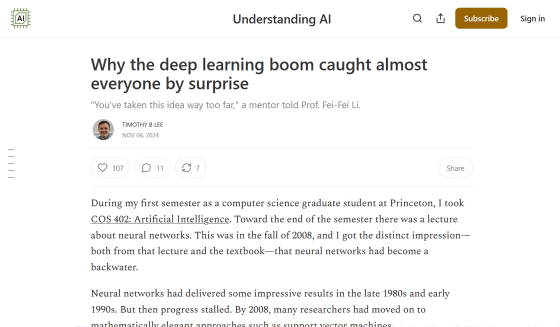
In recent years, AI cannot be discussed without deep learning, but it took a long time for the deep learning boom to occur in the AI field. Understanding AI lists three people who played a key role in the deep learning boom: computer scientist Geoffrey Hinton , who won the Nobel Prize in Physics in 2024, Jensen Huang , CEO of NVIDIA, a major GPU manufacturer, and computer scientist Fei-Fei Li , known as the godmother of AI, and introduces the contributions each of them made.
Geoffrey Hinton
A neural network is a network of millions of artificial neurons, each of which generates an output based on a weighted average of its inputs. For example, to create a network that can recognize handwritten digits like the one below, we take in the value of each pixel in the image and output a probability distribution of which digit it is: '0', '1', '2' ... If trained with enough sample images, the model should be able to output the correct digit with a high degree of accuracy.
Researchers had begun experimenting with single-layer neural networks in the late 1950s, but these were unable to output complex computational results, and it was unclear how to efficiently train multi-layer neural networks, so neural networks were on the decline by the time Hinton began his career in the 1970s.
Mr. Hinton
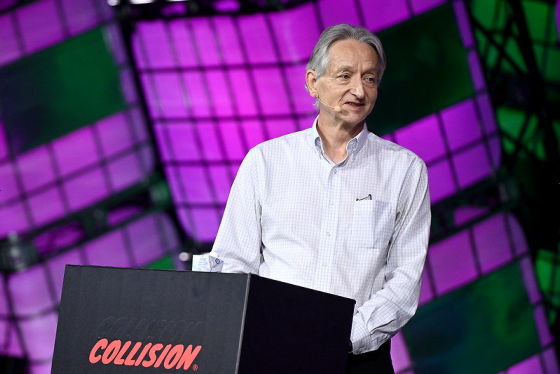
by
Nevertheless, Hinton continued his research on neural networks while moving from one affiliation to another, and in 1986 published a groundbreaking paper on ' backpropagation ,' a neural network learning algorithm. Backpropagation is a technique for adjusting the weights of each layer by transmitting the error from the output layer to the input layer in order to adjust the error between the output and the goal of a neural network.
Hinton's paper brought neural networks back into the spotlight, and Yann LeCun, a postdoctoral researcher at the University of Toronto under Hinton, succeeded in developing a model that could recognize handwritten characters. LeCun's model was used for processing bank checks, but at the time it was difficult to apply it to more complex images, and unfortunately neural networks reached a period of stagnation.
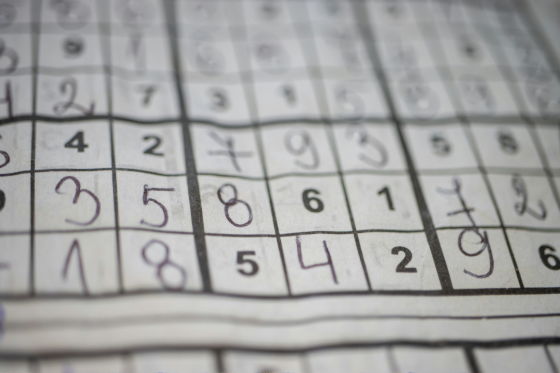
Jen-Hsun Huang
The CPU, the brain of your computer, is designed to perform calculations one step at a time, and for most software this works fine. But in some situations, like playing a game that renders a 3D world many times per second, the CPU just can't keep up. That's where gamers turn to the GPU. A GPU is a package of many execution units, and when rendering a game screen, different execution units can draw different areas in parallel, providing better image quality and frame rates than a CPU.
NVIDIA has dominated the market for a long time since developing the GPU in 1999. However, CEO Huang thought that the enormous computing power of GPUs could be applied to other computationally intensive fields such as weather simulation and oil exploration by scientists. So in 2006, NVIDIA introduced
Mr. Huang
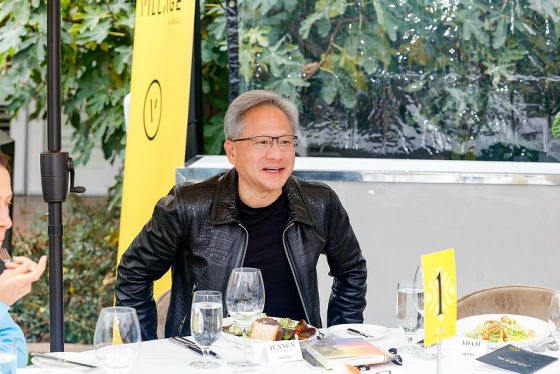
by Village Global
However, when CUDA was first announced, the reaction was weak, and there were criticisms of spending so much money on academic and scientific computing, which is not a very large market. Huang argued that CUDA would help grow the supercomputing sector, but this view was not widely accepted, and by the end of 2008, NVIDIA's stock price had fallen significantly. Some board members were concerned that the falling stock price would make NVIDIA a takeover target.
When Huang introduced CUDA, he didn't have AI or neural networks in mind. However, it soon became clear that Hinton's backpropagation algorithm was well suited to GPU parallel processing, and that neural networks would be CUDA's killer app. In 2009, Hinton's research team used the CUDA platform to train a neural network that recognizes human speech and published the results. After that, Hinton contacted NVIDIA to ask if they would provide GPUs for free, but unfortunately they declined.
◆ Fei Fei Li
While a doctoral student at California Institute of Technology, Li built a dataset called Caltech 101 , which consists of 9,000 images across 101 categories. Caltech 101 improved the accuracy of not only Li's own models but also those of other researchers, becoming a benchmark in the field of computer vision. From that experience, Li learned that computer vision algorithms tend to perform better when trained on larger and more diverse datasets.
So in January 2007, Li became an assistant professor of computer science at Princeton University and began thinking about building a 'truly comprehensive dataset that includes all the objects people encounter in the real world.' After hearing about the existence of the ' WordNet ' dataset, which contains 150,000 English words, Li created a list of 22,000 objects from WordNet, excluding shapeless words such as 'truth' and leaving only shapeless words such as 'ambulance' and 'zucchini.' He began using Google image search to find candidate images and label them.
A few months after the project began, Li said, his mentor advised him, 'I think this way of thinking is too much. The trick is to grow with the field. Don't jump into it all at once.' He said he was skeptical of others. In addition, even with the labeling process optimized, the initial assumption was that it would take more than 18 years to complete. However, by using Amazon's crowdsourcing platform, Amazon Mechanical Turk , the time required for completion was reduced to just two years.
Completed in 2009, ' ImageNet ' is a large-scale dataset with a total of 14 million images classified into approximately 22,000 categories, and Li presented ImageNet at an image recognition conference held in Miami. However, ImageNet was relegated to a poster session and did not receive the response that Li had initially hoped for. Still, Li decided to hold a competition for image recognition models using ImageNet to attract attention to ImageNet. In the competitions in 2010 and 2011, only models that were slightly improved from previous models were submitted. However, in 2012, Hinton's team submitted a deep learning-based neural network model, ' AlexNet ,' which significantly exceeded the accuracy of previous models, and other teams also submitted highly accurate models using neural networks.
The technology industry quickly recognized its usefulness after AlexNet won the ImageNet competition in 2012. In an interview in September 2024, Li said, 'That moment (when AlexNet won the competition) was very symbolic for the AI world because it was the first time that the three fundamental elements of modern AI converged. The first element was neural networks. The second element was big data with ImageNet. And the third element was GPU computing.'
CHM Live | Fei-Fei Li's AI Journey - YouTube
Understanding AI states that one lesson to be learned from the development of deep learning is that 'it is a mistake to cling too closely to conventional wisdom.' If the growth of AI loses momentum in the next few years, Understanding AI argues that a 'new generation of staunch nonconformists' will realize that old approaches are stagnating and need to try something different.
Related Posts: