``MatX'', a startup that creates customized hardware that increases the computational power of large-scale language models by an order of magnitude
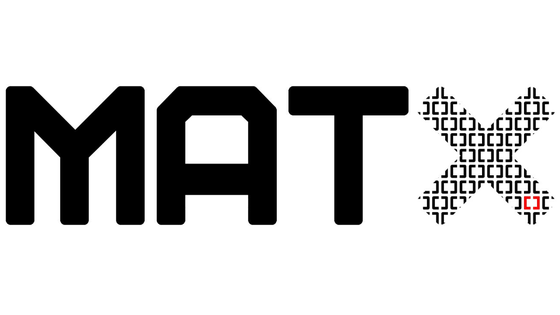
Former Google engineers Reiner Pope and Mike Gunter founded MatX , a startup that designs hardware with the aim of 'specializing in large AI models and further improving performance.' .
MatX | MatX: high throughput chips for LLMs
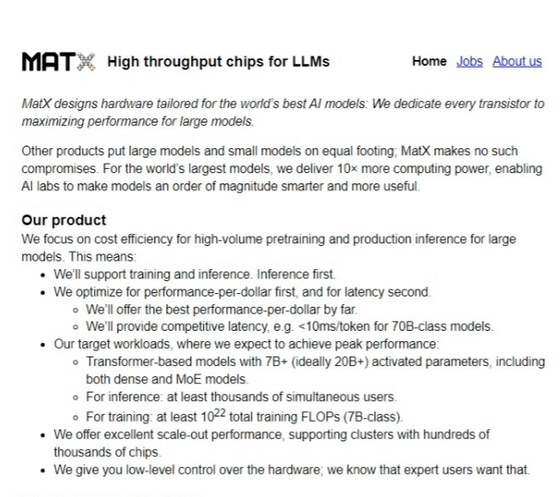
Introducing MatX: we design hardware tailored for LLMs, to deliver an order of magnitude more computing power so AI labs can make their models an order of magnitude smarter.
— MatX (@MatXComputing) March 26, 2024
Our hardware would make it possible to train GPT-4 and run ChatGPT, but on the budget of a small startup.… pic.twitter.com/6CbDYwLYvT
AI Chip Startups Like MatX Storm Silicon Valley - Bloomberg
https://www.bloomberg.com/news/articles/2024-03-26/ai-chip-startups-like-matx-storm-silicon-valley
Mr. Pope, who is also CEO, has been involved in building a wide range of software and hardware at Google for over 10 years, from machine learning chips to distributed system infrastructure and large-scale language models. CTO Gunter has been in charge of hardware architecture for 28 years and was the chief architect of machine learning chips at Google.
MatX's banner is 'high-performance through-chip manufacturer.' That's because while other chipmakers treat small and large AI models equally, MatX dedicates every chip to maximizing the performance of the world's largest AI models. .
MatX's goal is to ``make the world's best AI models operate as efficiently as possible in reality, and lead the world in AI quality and availability for years to come.''
In particular, the chip design focused on high-capacity pre-training for large-scale models and cost-efficiency for production inference. In terms of support priorities, inference comes first, training comes second, and performance per cost is optimized first, followed by latency.
The target workload that is expected to achieve peak performance is a large Transformer-based model with 7 billion (ideally 20 billion or more) parameters, including both dense and MoE (mixed expert) models. In the case of inference, the number of simultaneous users is at least several thousand, and in the case of training, it is a minimum of 10ZFLOPS (10²²) with a 7B (7 billion parameters) class LLM.
It provides excellent scale-out performance to support clusters with hundreds of thousands of chips, as well as the low-level control over the hardware that expert users require.
MatX's chips will bring the world's best AI models three to five years earlier, and allow researchers to train 7B-class models daily and 70B-class models multiple times a month. MatX says that it will be. Additionally, even seed-stage startups can afford to train a GPT-4 class model from scratch and serve it with ChatGPT-level traffic.
Related Posts:
in Hardware, Posted by logc_nt