Google announces new technology 'SMERF' that realizes high-precision rendering in real time
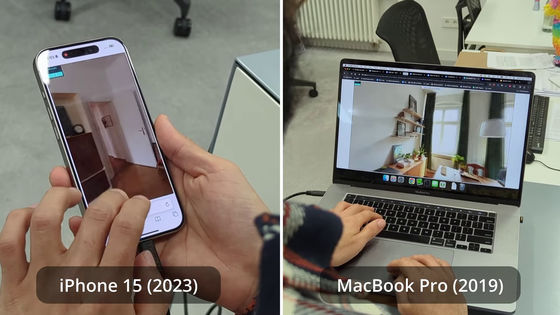
We have further developed NeRF (Neural Radiance Fields) and
SMERF
https://smerf-3d.github.io/
You can see what kind of technology it is by watching the video below.
SMERF: Streamable Memory Efficient Radiance Fields for Real-Time Large-Scene Exploration - YouTube
SMERF was developed by Daniel Duckworth of Google DeepMind and others. SMERF is an acronym for ``Streamable Memory Efficient Radiance Fields'', which refers to ``streamable, memory-efficient luminance fields.'' Regarding what is output by SMERF, Mr. Duckworth said on the social news site Hacker News, ``Images are not an appropriate expression.In the previous MERF, feature vectors were stored in PNG images, We're replacing it with a binary array.'
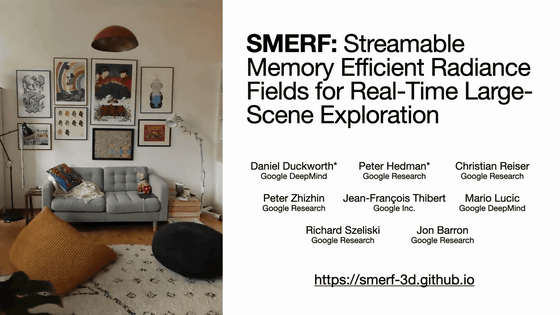
NeRF can output near-realistic images in large-scale, borderless 3D landscapes. However, Zip-NeRF, which has improved accuracy, has the disadvantage that it requires too much calculation for real-time rendering.
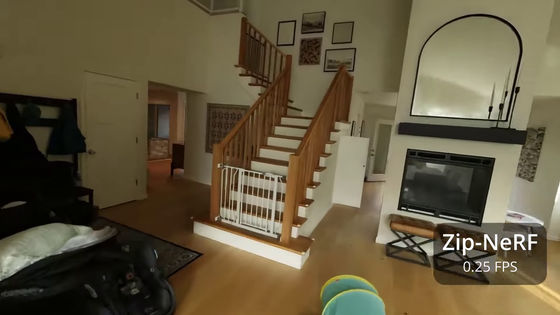
SMERF is similar to Zip-NeRF in that it renders details down to just a few centimeters, while its computational complexity and memory requirements are comparable to existing real-time rendering technology MERF.
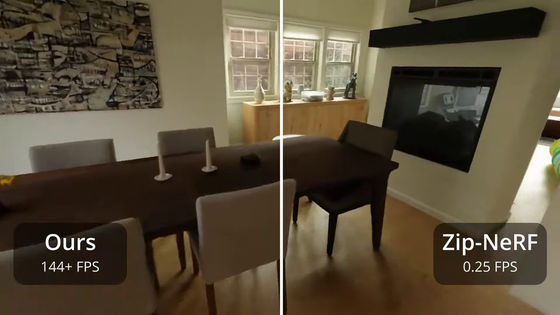
As a result, SMERF is capable of rendering at 60 frames per second through a web browser on a typical laptop or smartphone. This is achieved by streaming landscape depictions at appropriate locations based on camera position.
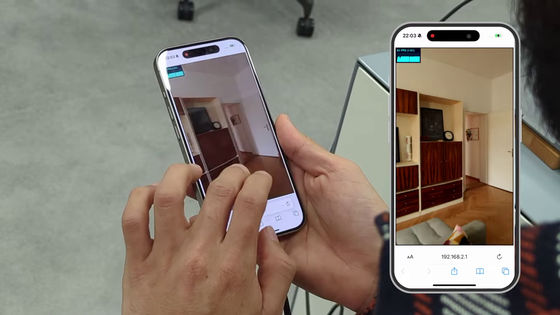
There are several points to realize SMERF. The first is the real-time representation of smaller, borderless 3D landscapes. Duckworth et al. built on MERF and significantly improved the visual fidelity and quality of geometric reconstruction.
The second is a new extraction learning method for luminance fields. Rather than training from posed photographs, Duckworth and colleagues slowly extracted geometric and photometric information from a high-fidelity 'teacher.' It is thanks to this method that it surpasses the quality of other real-time viewpoint synthesis technologies such as 3D Gaussian Splatting technology.
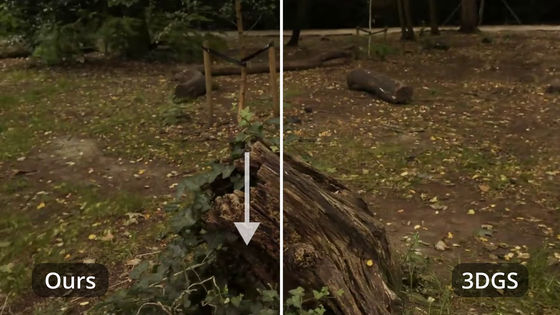
The representation size of these artifacts changes depending on the size of the depicted scene, so it takes time to thoroughly capture them, but in the case of SMERF, the landscape is divided into multiple regions based on the camera position. This avoids rendering time issues. Each area is assigned sub-models supervised by a common teacher. At any point in time, a single submodel is sufficient to depict the target view, reducing computational power and memory requirements without compromising visual fidelity.
Example of 3D Gaussian Splatting where the area around the window is not depicted correctly
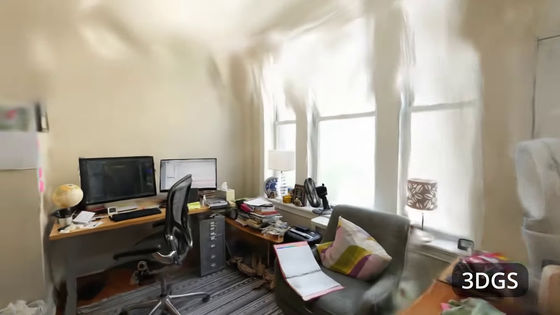
SMERF solves this problem to a large extent
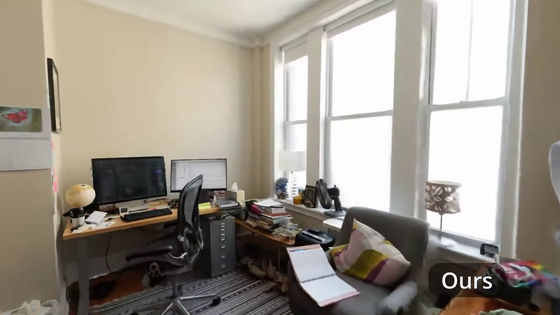
Compared to 3D Gaussian Splatting, SMERF can better express mirror surfaces and reflections, and with a spatial resolution of 3.5 mm cube, it can reliably reconstruct landscapes up to 300 m square.
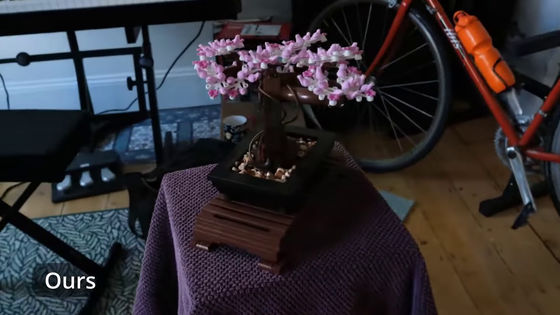
Also, unlike 3D Gaussian Splatting, it is compatible with natural lens models, so it is also possible to switch to a fisheye lens.
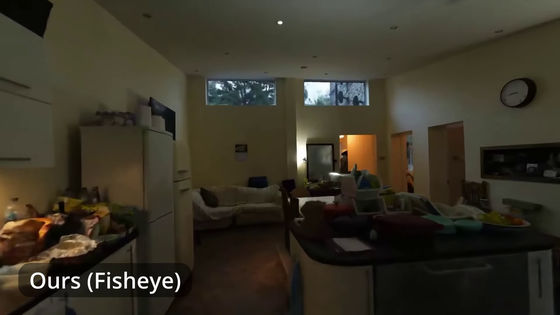
The most important point, Duckworth et al. points out is that SMERF can be easily used on PCs and smartphones. The amount of memory required is reduced by streaming the optimal sub-model parameters based on the user's position within the landscape.
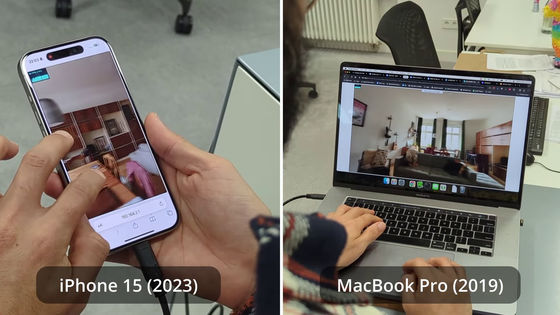
On Hacker News, Mr. Duckworth himself responded to comments to users, such as ``I think it would feel more natural if there was a mode that uses the mobile phone's compass and gyro for navigation.' ' I agree with you.The UX of movement could be improved further .”
Related Posts: