An attempt to count the crowding rate of university cafeterias using smartphone Bluetooth
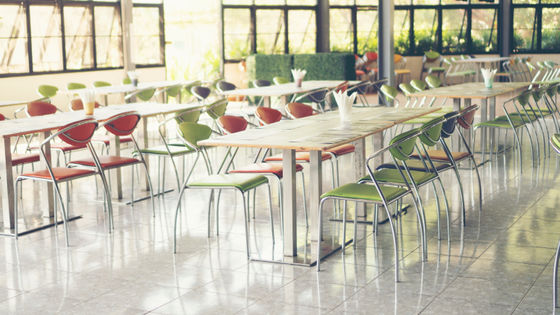
The world is full of devices that communicate using the Bluetooth standard, such as smartphones, smart watches, and headphones. An attempt was made by Matthew Esposito of the College of William & Mary to detect the signals of these terminals and measure the congestion rate.
Building an occupancy sensor with a $5 ESP32 and a serverless DB
The College of William & Mary originally partnered with user monitoring service Bluefox to release a congestion monitoring system using Bluetooth. The monitoring method is simple: counting the MAC addresses of smartphones that have Bluetooth turned on. However, because the university terminated its contract with Bluefox, it was no longer possible to monitor users.
The idea of using Bluetooth to track crowding is still simmering in Esposito's mind, and he hopes to revive his own crowd-monitoring system to detect crowding rates everywhere on campus. I tried.
For the time being, Mr. Esposito wrote a code, carried his laptop around and actually tried it, and he said that he was able to count quite accurately in areas such as small cafes. They were able to count to some extent even in large areas like the dining room, but there were also numbers that seemed to be picking up signals from people on the terrace through the wall.
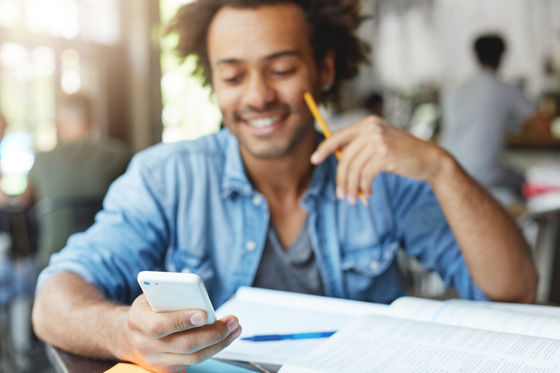
With this method, it cannot be detected in places where there is no laptop, so Mr. Esposito purchased an OLED developer board '
The result was successful for the time being, but it seems that ESP32 crashed when about 250 devices were counted. This is because the amount of information obtained from one terminal is too large, and Mr. Esposito counters this by reducing the amount of information. Also, the ESP32 was replaced with another one and tested repeatedly.
As a result, the only device that worked reliably for one month was the 'XIAO ESP32C3/S3'. With this device and Esposito's code, it seems to work fine without slowing down.
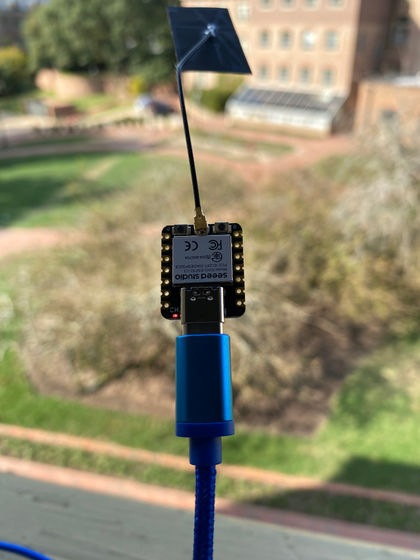
Below is an example of the results measured for one day. The part where the value rises rapidly is the break time between jobs, which is the time when there is the most traffic.
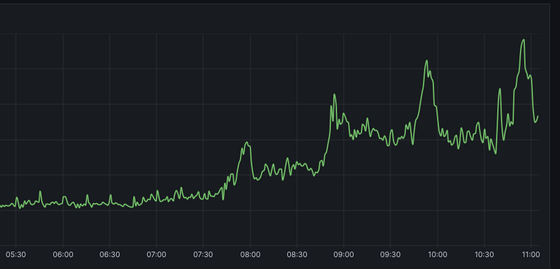
The method that Mr. Esposito used this time would also detect smart watches and headphones, resulting in double detection of people with two or more Bluetooth devices. Also, it is impossible to measure people who have turned off Bluetooth on their devices.
Mr. Esposito is considering ways to improve accuracy by filtering by manufacturer ID such as Apple or Google, and is talking with several professors about whether it is worthy of academic study. They are also considering selling it to brick-and-mortar businesses that want to measure occupancy trends, and will continue to develop it.
Related Posts: