Microsoft Research develops AI 'EvoDiff' that generates new proteins from amino acid sequences, a major breakthrough in protein engineering
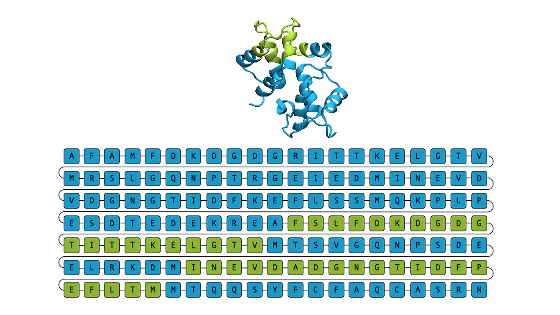
A team at
Protein generation with evolutionary diffusion: sequence is all you need | bioRxiv
https://www.biorxiv.org/content/10.1101/2023.09.11.556673v1
Abstracts: September 13, 2023 - Microsoft Research
https://www.microsoft.com/en-us/research/podcast/abstracts-september-13-2023/
Microsoft open sources EvoDiff, a novel protein-generating AI | TechCrunch
https://techcrunch.com/2023/09/14/microsoft-open-sources-evodiff-a-novel-protein-generating-ai/
Proteins are molecules that are involved in various cellular processes in the body, such as hemoglobin , which transports oxygen in the blood, and insulin , which regulates blood sugar levels. Proteins are involved in the mechanisms of various diseases and are often used for treatment, so creating new useful proteins is important in medical research.
Furthermore, proteins are used not only for their activities within living organisms, but also for industrial purposes, such as acting as catalysts and enzymes for manufacturing chemical substances. By increasing the ability to produce proteins with specific functions, it is possible to create enzymes that decompose plastic waste, enzymes that make photosynthesis more efficient, etc., and be able to address the various problems facing modern society. .
Therefore, a research team at Microsoft Research developed an AI 'EvoDiff' that generates new proteins. Approaches to generate proteins using AI have existed for some time, but ``first, we consider a three-dimensional structure of a protein that can perform a specific task in the body, and then we calculate the amino acid sequence of a protein that can be folded into that three-dimensional structure.'' The traditional approach of ``discovering'' has the problem of requiring high costs in terms of both computing and human resources.
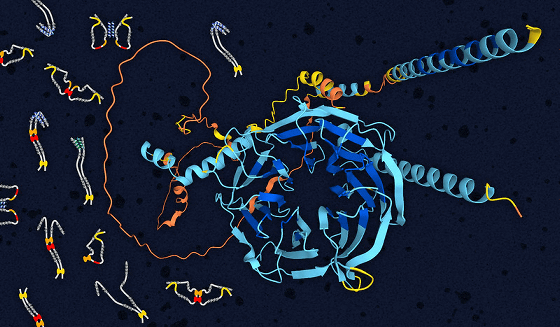
by
Therefore, the research team developed an approach that generates new proteins based only on the protein's amino acid sequence, rather than generating new proteins starting from the protein's three-dimensional structure. In the first place, approaches based on the three-dimensional structure of proteins have the problem that the range of training data is significantly limited because the number of three-dimensional structures that can be used as a data set is limited. By focusing on amino acid sequences, the researchers say they were able to obtain a large and diverse evolutionary dataset to train the AI.
'We are excited that EvoDiff will extend the power of protein engineering beyond the structure-function paradigm to programmable arrays,' Kaishu Yang , a researcher at Microsoft Research and senior author of the paper, told TechCrunch in an email interview. We are looking to expand to prioritized design.With EvoDiff, we are discovering that in order to design new proteins in a controllable way, it is not actually the 3D structure that is needed, but rather 'the sequence of the protein'. We are demonstrating that this is possible.'
In his X post, Yang posted a GIF video showing how to reconstruct a three-dimensional structure from a protein's amino acid sequence.
EvoDiff combines evolutionary-scale data with diffusion models for controllable protein sequence generation.
— Kevin K. Yang Yang Kaichuang (@KevinKaichuang) September 13, 2023
In addition to generating plausible proteins, we can scaffold structural motifs in sequence space!
Preprint: https://t.co/zd1zPoKOWY
Code: https://t.co/vD3CXSUY5V pic.twitter.com/fVLM39jBRr
At the core of the EvoDiff framework is a 640 million parameter model trained on a massive dataset of protein amino acid sequences and functional information. EvoDiff uses the same diffusion model as Stable Diffusion, an image generation AI, and gradually reduces noise from the starting protein sequence, which is mostly composed of noise, gradually approaching the protein sequence. thing.
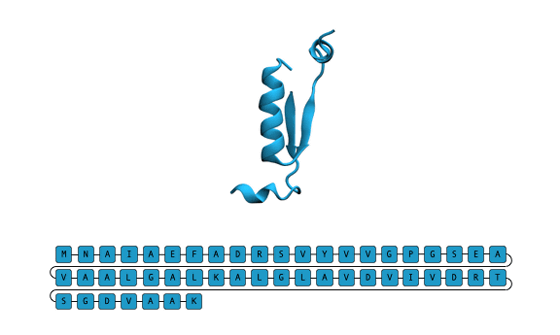
Conventional three-dimensional structure-based approaches have had the problem of not being able to synthesize naturally denatured proteins that do not have a well-defined three-dimensional structure, but EvoDiff, which is sequence-based, can also generate naturally denatured proteins. These naturally denatured proteins play important roles in biology and disease mechanisms, including enhancing or decreasing the activity of other proteins.
It is also possible to maintain a structural motif , which is a group of proteins with a specific function or structure, and create a new protein by complementing the surrounding motif.
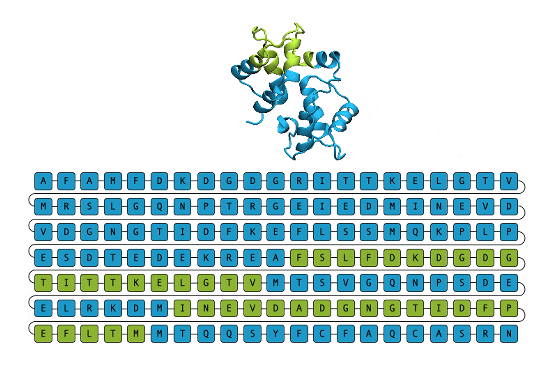
The research team claims that the protein amino acid sequences generated by EvoDiff cover the entire structural, functional, and sequence spatial characteristics of proteins that exist in nature. In the future, the research team says that they plan to test the proteins actually produced by EvoDiff in the laboratory to find out whether they really work.
The EvoDiff code is available on GitHub.
GitHub - microsoft/evodiff: Generation of protein sequences and evolutionary alignments via discrete diffusion models
https://github.com/microsoft/evodiff
Related Posts: