Does AI 'understand' the meaning of words like humans do?
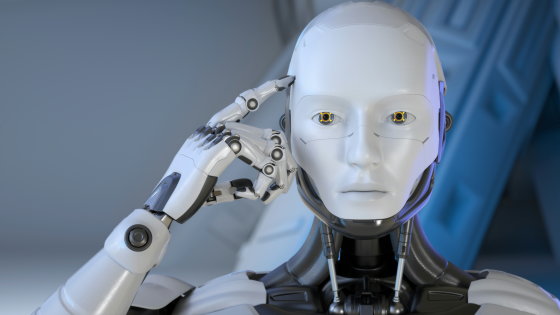
In recent years, research on artificial intelligence (AI) has been progressing at a rapid pace, and 'AI that generates natural sentences' represented by '
What Does It Mean for AI to Understand? | Quanta Magazine
https://www.quantamagazine.org/what-does-it-mean-for-ai-to-understand-20211216/
'Natural language understanding has been a goal of AI research for many years,' says Mitchell, who is working hard to build an AI that can understand and read and write languages like humans. I've been. Initially, researchers were looking for ways to manually program the 'all elements and rules' needed to understand a news article or fiction text, but write down everything needed to understand the text. That was practically impossible. Therefore, in recent years, a method of 'learning a huge amount of text data and making AI itself understand the language' has been established.
AI built on a huge amount of text data is called a 'language model', and a large-scale neural network like GPT-3 can generate sentences that are seemingly indistinguishable from humans. However, Mitchell said, 'Does AI really understand the seemingly logical sentences that it produces?', Saying that AI's understanding of language remains questionable.
One way to determine if a machine understands the meaning of a word is the ' Turing test ' proposed by Alan Turing, a leading computer science expert in 1950. The Turing test states that 'a machine has the intelligence to think about things when a human and a machine are made to interact with each other only by a written conversation, and the judge who sees the conversation cannot correctly distinguish between the human and the machine.' But Mitchell said, 'Unfortunately Turing underestimated the tendency of humans to be fooled by machines.' In fact, even early relatively simple chatbots such as ELIZA, developed in the 1960s, were able to achieve some success in the Turing test.
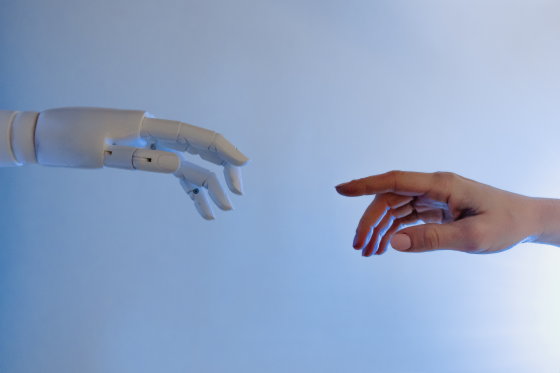
Sentence 1: I poured water from the bottle into the cup until it was full.
Question 1: What was full, the bottle or the cup?
Sentence 2: I poured water from the bottle into the cup until it was empty.
Question 2: What was empty, the bottle or the cup?
Sentence 1: Joe's uncle can still beat him at tennis, even though he is 30 years older.
Question 1: Who is older, Joe or Joe's uncle? (Which is older, Joe or Joe's uncle?)
Sentence 2: Joe's uncle can still beat him at tennis, even though he is 30 years younger. (Joe's uncle can beat him by tennis even though he is 30 years younger)
Question 2: Who is younger, Joe or Joe's uncle? (Which is younger, Joe or Joe's uncle?)
A 'common sense understanding' is considered necessary to correctly answer these sentences and questions that contain pronouns. The Winograd Schema Challenge is said to be able to test AI comprehension more quantitatively rather than relying on human vague judgments, and the author of the paper said, 'Do not find the answer to your question on Google search. The question is designed with 'to do' in mind. At the competition held in 2016, even AI with the highest correct answer rate of Winograd Schema Challenge could answer only 58%, which is not much different from the case of randomly answering.
However, in recent years, with the advent of large-scale neural networks, the percentage of AI answering the Winograd Schema Challenge has increased dramatically. A 2020 paper reports that GPT-3 recorded a correct answer rate of nearly 90% for the Winograd Schema Challenge, with other language models producing comparable or better results. At the time of writing, the state-of-the-art language model boasts a rate of correct answers comparable to humans in the Winograd Schema Challenge, but Mitchell still says, 'The language model does not understand the language like humans.' Claims.
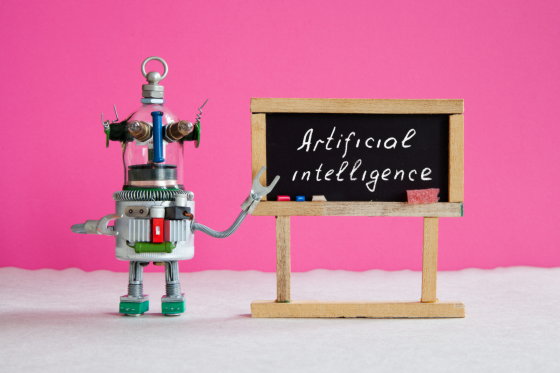
Mitchell points out that the problem with AI is that 'AI can create shortcuts in the Winograd Schema Challenge to reach the answer without understanding the meaning of the sentence.' For example, 'The sports car passed the mail truck because it was going faster.' 'The sports car passed the mail truck because it was going slower.' (Sports cars overtook postal cars because they were slow) '.
Humans can imagine sports cars, postman cars, roads, their speeds, etc. in their heads. However, AI is based on the huge amount of text data in English-speaking countries, and the correlation between 'sports car' and 'fast' and the correlation between 'mail truck' and 'slow'. It just absorbs the relationship and gives the correct answer based on the correlation. In other words, Mitchell argues that the AI process of answering based solely on the correlation of text data would be different from human 'understanding.'
To solve these problems with the Winograd Schema Challenge,
But still, Mitchell said, 'I repeat, it's unlikely' when it comes to the question of whether AI has gained a human-like common-sense understanding. In fact, in a follow-up survey on WinoGrande, AI was tested by the method of 'making a set of'two pairs of sentences'composed of almost the same words, and if both are correct, it is considered to be the correct answer.' However, the percentage of correct answers was much lower than that of humans.
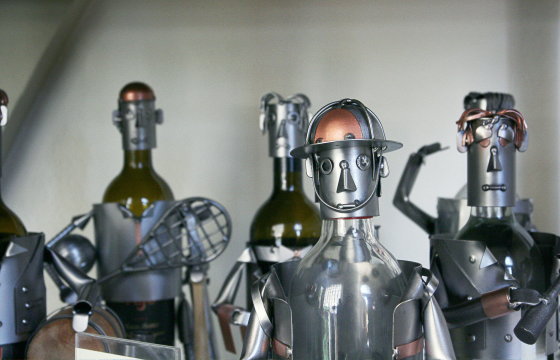
A lesson learned from a series of efforts to test AI is that 'it's hard to tell from the performance of a given task whether an AI system really makes sense.' This is because neural network language models often answer based on statistical correlations rather than understanding the meaning of sentences like humans do.
'In my view, the heart of the problem is that'to understand language, we need to understand the world, and machines exposed only to language cannot get that understanding.'' It is pointed out. To understand the sentence, 'Sports cars overtook postal vehicles because of their speed,' 'What are sports cars and postal vehicles?' 'Cars can overtake or overtake each other.' It is necessary to know the fundamental common sense and concept of the world, such as 'a car is an object that is operated by humans, exists in the world, and interacts with each other.'
'Some cognitive scientists need to rely on
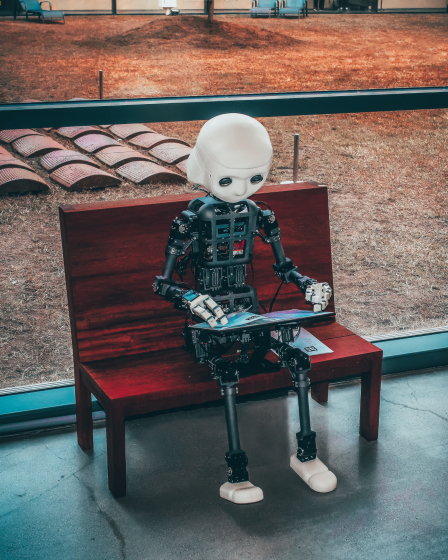
Related Posts: