Facebook develops new machine translation system that can translate 100 languages directly without going through English
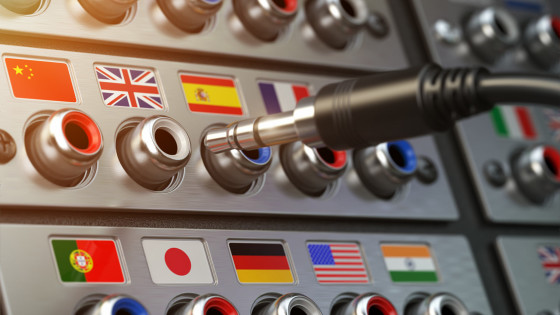
Facebook, which has users all over the world, can use automatic machine translation to translate almost all content published on the platform into the language used by users. Facebook has announced that it has developed a machine translation system ' M2M-100 ' that can directly translate 100 languages as an intermediate language without going through English.
Introducing the First AI Model That Translates 100 Languages Without Relying on English --About Facebook
Facebook's new AI can translate languages directly into one another | Engadget
https://www.engadget.com/facebooks-ai-can-translate-languages-directly-into-one-another-150029679.html
Facebook provides 20 billion translations per day on its news feed alone, but the translation system typically uses English as its intermediate language. For example, when translating from Chinese to French, we first translate Chinese into English, and then translate that English into French to make Chinese into French.
This method is used because of the huge translation datasets for English and other languages, but it is said that inserting English in between reduces the overall accuracy of the translation. Angela Fan of Facebook AI points out that it is important for machine translation systems to meet the demands of non-English people, as there are many regions around the world that speak languages other than English. Billions of posts are posted daily on the Facebook platform, but each post uses 160 languages, and more than two-thirds of the posts are in languages other than English. Fan said.
Therefore, Facebook has developed a new machine translation system 'M2M-100' that can directly translate two languages without using English as an intermediate language. Facebook claims that the M2M-100 is the first multilingual machine translation model that can translate directly from a total of 100 language sets in any direction.
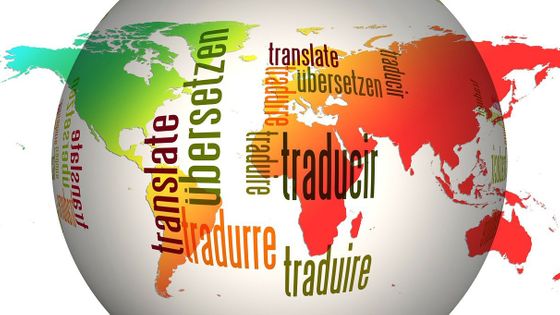
In developing the M2M-100, Facebook built a huge dataset of 7.5 billion sentences in 100 languages. First, he used
Although translation data is often created using human translators, it is much better to find 'French and Tamil-speaking translators' than to find 'English and Tamil- speaking translators'. Fan points out that it is difficult. In order to obtain data for directly translating languages other than English, the research team used a tool called ' LASER (Language-Agnostic SEntence Representations) ' that maps multilingual sentences based on their meaning. ..
Facebook has also introduced a strategy to classify languages into 14 groups based on language classification, geography, and cultural similarities. Facebook says it has higher quality two-way translation data because languages that belong to the same group tend to communicate more often.
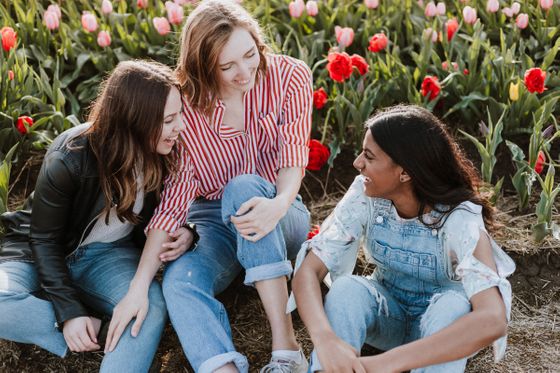
Of course, not all languages have a large amount of text available on the Internet, so the research team focused on 'data written in a single language.' Taking the Chinese-to-French translation as an example, Fan said, 'If our goal is to translate from Chinese to French, but for some reason we don't get enough accurate data, we'll do this. Use French single language data to improve, and train the reverse of the system, 'Translate from French to Chinese'. For example, get all the French data from Wikipedia and use it. I will translate it into Chinese. '
By adding new text obtained by performing reverse translation to the dataset, the data available on both the input side and the output side will increase, so the machine translation system will become more powerful.
Facebook states that the M2M-100 developed in this way outperforms machine translation systems that use English as an intermediate language in the BLEU (Bilingual Evaluation Understudy) score, which measures the accuracy of machine translation.
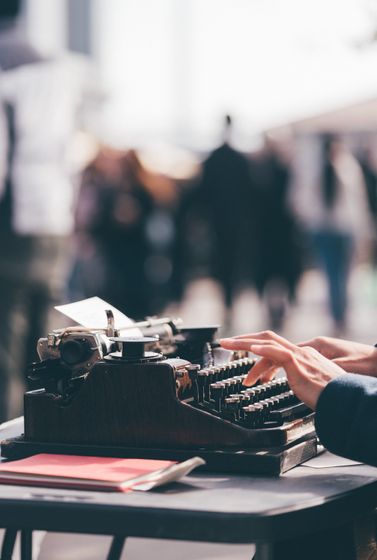
The number of languages that the M2M-100 cannot cover is enormous, and it is unclear at the time of writing the article whether it will eventually lead to the development of a system that can directly translate all the languages that exist in the world. Fan pointed out that the success of machine translation systems depends on the amount of data that AI can utilize, and said there are additional research challenges in languages with very little available data.
Related Posts:
in Software, Posted by log1h_ik