What is 'sentiment analysis' that determines people's emotions and the risk of depression from Twitter and Facebook posts?
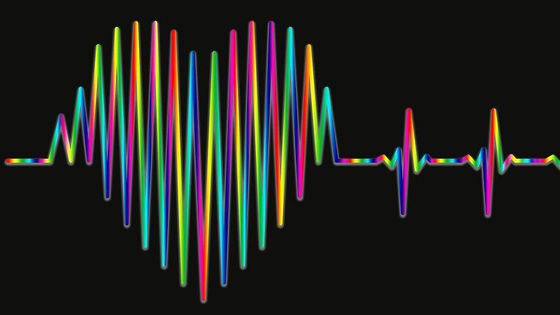
A research team at the University of Vermont in the United States examined tweets in 2020 with a '
How algorithms discern our mood from what we write online
https://www.knowablemagazine.org/article/technology/2020/how-algorithms-discern-our-mood-what-we-write-online
◆ Technology used in sentiment analysis
Below are the results of the August 2019-September 2020 hednometer analysis published by the University of Vermont. The gray graph below shows the total number of tweets, and the line graph above shows the feelings analyzed by the hednometer. I will. Looking at the part surrounded by the red frame, around March 2020 when the new coronavirus infection began to rage in earnest, and in 2020 when a black man died due to improper detention of police officers. At the end of May, we can see that people's emotions are very depressed.
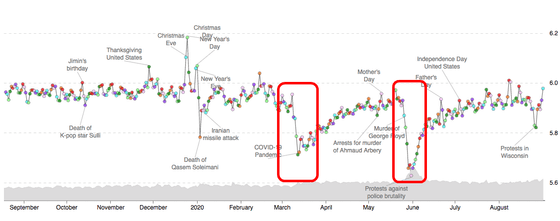
According to Mackenzie, the basic approach to sentiment analysis, such as a hednometer, is word count. The principle is very simple: count the number of positive words and subtract the number of negative words from it. However, the problem of 'ignoring the context' lies in the simple counting of words.
For example, one person said, 'I'm so happy that my iPhone is nothing like my old ugly Droid. (I'm happy because my iPhone is so different from my old terrible Android device).' Suppose you posted on Twitter.
Since humans can understand the connection of words, it is easy to understand that 'the poster of this tweet is happy', but in the sentiment analysis that simply counts the number of words, 'the poster is denied'. The result is 'feelings'. Because there are three negative words, 'nothing', 'old', and 'ugly', while there is only one positive word, 'happy'. is.
Researchers used
In 2013, AI researcher Tomas Mikolov developed a technique called word embedding using machine learning, which took further research in this field. Word embedding is also called 'distributed expression' and is expressed by converting words into 50 to 300 kinds of numbers called vectors. This allows machine learning models to predict the next word after a particular word with high accuracy, and to recognize synonyms such as 'money' and 'cash' to capture context. It is said that it has become.
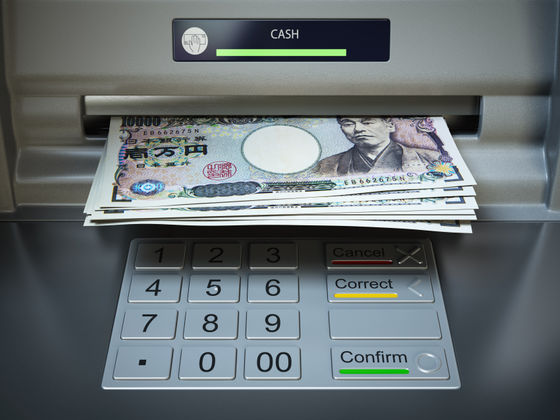
◆ Roots of sentiment analysis and application to SNS
As mentioned above, sentiment analysis is often talked about in the field of computer science, but historically it can be said that it is a research field deeply rooted in the field of psychology. In 1962, Harvard psychologist Professor Philip Stone developed the earliest text analysis program, General Inquirer . This confirms that patients diagnosed with depression tend to use words such as 'I' and 'me' and words that express negative emotions, especially those related to death. This is the root of sentiment analysis.
With the advancement of technology, sentiment analysis has been applied to SNS, and has achieved results such as detecting signs of depression and suicide. For example, in 2017, Facebook introduced an AI that detects posts that suggest suicide, gives users contact information for support groups, and reports posts to experts and police.
Efforts are also being made to assess the risk of depression using Twitter. The following is a graph that analyzes how the risk of depression changed before and after the diagnosis of depression, based on tweets provided by a total of 200 depressed patients and healthy people. Looking at the time point 200 days before the diagnosis of depression, which is surrounded by a red frame, there is already a considerable difference between the blue graph showing depressed patients and the green graph showing healthy people. You can see that.
Sentiment analysis remains a number of challenges, including privacy issues with analyzing social media posts, but it is hoped that this technology will enable early detection of signs of suicide and the risk of depression. I will.
◆ Analysis of 'mood' is also possible
Sentiment analysis in recent years has made it possible to quantify not only fairly strong emotions such as the urge to commit suicide, but also vague moods. For example, in a 2018
Also, in a 2017 study that analyzed the lyrics of songs of various genres, 'rock in the 1960s' and 'religious music' shown in red frames had the most positive tone lyrics, such as 'punk'. We also know that 'metal' has many lyrics with the most negative tones.
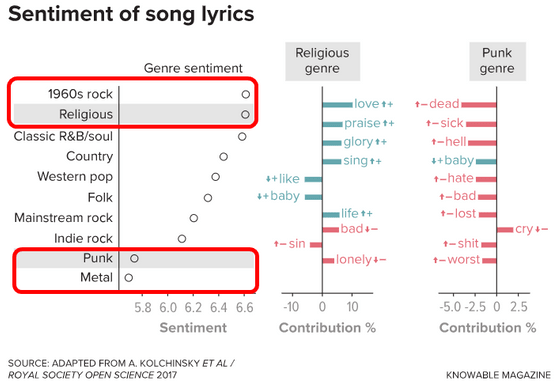
◆ Future of sentiment analysis
According to Bing Liu, a sentiment analysis researcher at the University of Illinois at Chicago, many companies such as Microsoft, Google, and Amazon are applying sentiment analysis to their businesses, in addition to the above-mentioned SNS such as Twitter and Facebook. .. For example, a 2018 (PDF file) study that scrutinized multiple sentiment analysis systems found that 28 sentiment analysis systems were already in use in industry and academia at that time.
These sentimental analyzes are primarily used to measure customer satisfaction, but like IBM's Social Pulse, a system that monitors the company's network to see what employees are dissatisfied with. Has also appeared. Regarding IBM's Social Pulse, Chris Dunnforce of the University of Vermont, who developed the Twitter-based hednometer, said, 'I'm concerned that employee privacy will be hazy in front of the company's interests. That's a pretty suspicious ethical thing, 'he said.
In addition, Mackenzie quoted Liu as saying, 'We don't even know what we know,' and said, 'As sentiment analysis becomes more common,'ethics' It's likely to continue to be a problem, 'he said, saying that sentiment analysis cannot avoid issues such as privacy and ethics in the future.
Related Posts:
in Software, Posted by log1l_ks