Artificial intelligence is growing dozens of times faster than Moore's Law
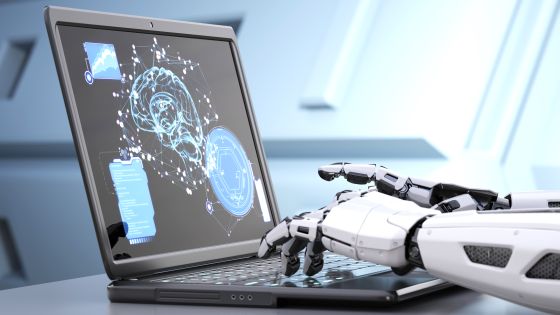
AI Training Costs Are Improving at 50x the Speed of Moore's Law
https://ark-invest.com/analyst-research/ai-training/
From 2010 to 2020, the computer processing power spent on AI training models has increased rapidly. In the graph below, the vertical axis is the processing capacity, and “Petaflop/Days” means that 4 trillion operations are performed per second per day. The horizontal axis is the Christian era.
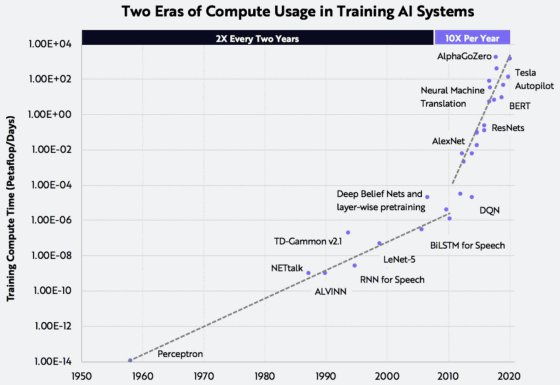
Since the introduction of the
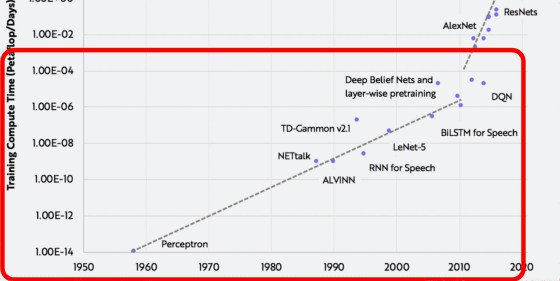
However, processing capacity from 2010 to 2020 is growing about 10 times per year as shown in the graph below, more than 5 times faster than Moore's law, Wan says ..
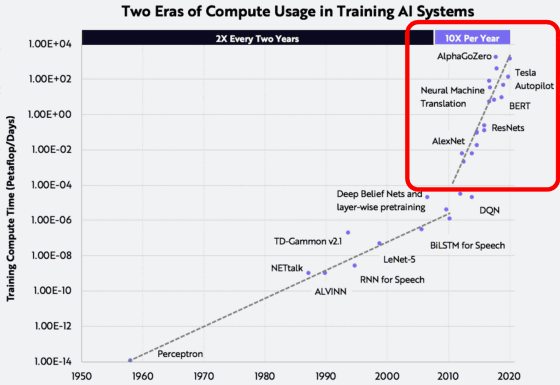
Large companies, such as Google's
Another important point is that training costs for deep learning in AI have dropped by a factor of 10 each year. For example, around 2017, it took about $1000 (about 108,000 yen) to train an image recognition network like ResNet-50 on the public cloud , but in 2019, the required cost is about $10. It is down to (about 1080 yen). In the graph below, the vertical axis is cost, the horizontal axis is year and month, and each point is Legacy GPU (black) that refers to the old-generation NVIDIA GPU, NVIDIA's Volta GPU (blue), Google's Cloud TPU (red).
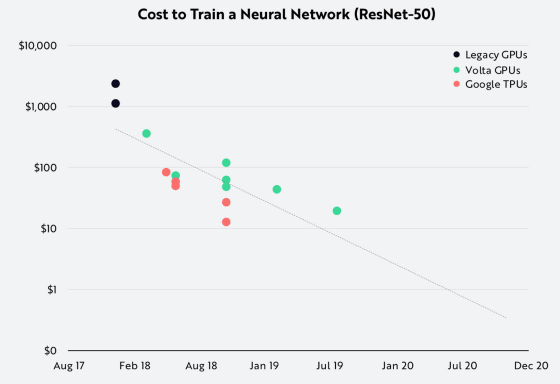
If the downward trend of costs from 2017 to 2019 is maintained, it is possible that the cost will drop to about $1 (about 108 yen) by 2020, but Mr. Wang said, ' Can't be trusted.'
In the graph below, the vertical axis represents cost and the horizontal axis represents year and month. The cost for classifying about 1 billion images was about $ 10,000 (about 1.08 million yen) in 2017, but it will be only about 0.03 dollars (about 3 yen) in 2020 Has dropped to.
Comparing the rate of change in costs associated with deep learning with Moore's Law, Mr. Wang argues that it changes by 10 to 100 times Moore's Law. Breakthroughs in both hardware and software made it possible to drastically reduce costs. Between 2017 and 2020, the technology of IC chips and system design has evolved dramatically, and dedicated hardware for deep learning has also appeared. doing.
The graph below shows the performance evaluation of
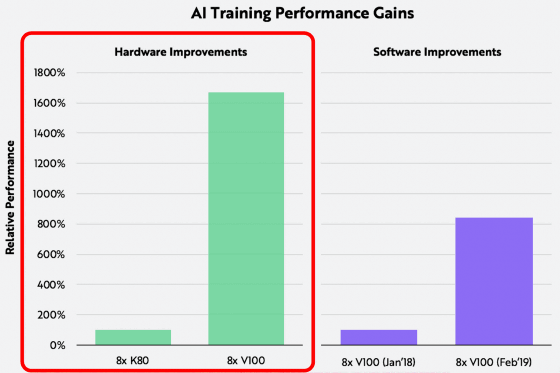
In addition, a framework of deep learning in V100 Tesla
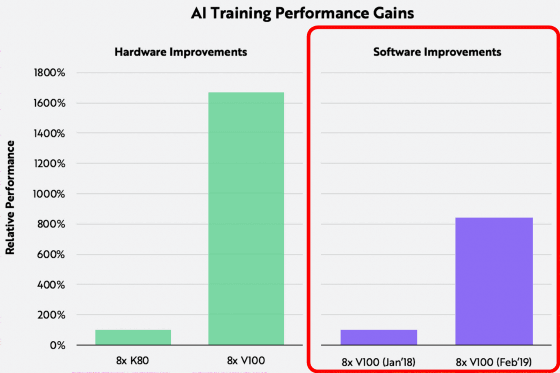
Meanwhile, hardware costs haven't fallen and have remained high over the years, Wang said. For example, the price of NVIDIA GPUs for data centers has tripled compared to the past three generations. Since Amazon Web Services introduced NVIDIA's Tesla V100 in 2017, the price of the service has remained constant.
Mr. Wang also argues that if AI technology continues to improve, it will significantly increase the market capitalization of AI-related companies in the global stock market. Below is a graph showing the market capitalization of information technology (black), internet (blue), artificial intelligence and deep learning (green) related companies. The vertical axis represents the share of the global stock market, and the horizontal axis represents the year. As of 2019, AI has a market capitalization of about 1 trillion dollars (about 107 trillion yen) in the global stock market, but it will expand to 30 trillion dollars (about 321 trillion yen) by 2037. Wang speculates that it will have a higher market capitalization than Internet companies.
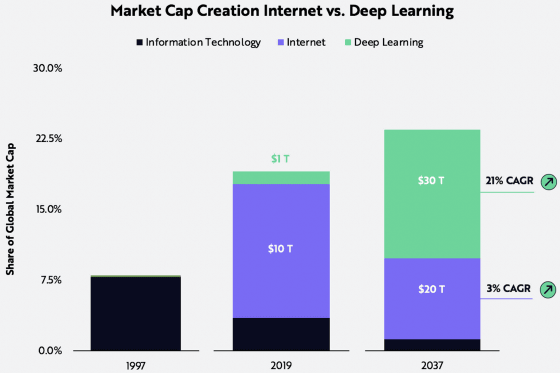
'The AI is still developing and will likely grow sustainably for decades,' said Wang, given the increasing computing power of AI, the declining cost of deep learning, and market capitalization in the stock market. I predicted.
Related Posts: