DeepMind who developed the world's strongest Go AI developed AI of 'FPS player transcending human being'
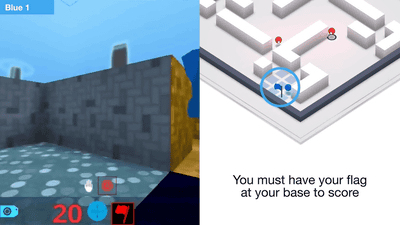
DeepMind , an artificial intelligence (AI) development company that developed the world's strongest go AI " AlphaGo Zero " with the same Alphabet as Google, launches a winning rate beyond humans in the first person shooter game (FPS) " For the Win (FTW)" developed. It is not just to defeat enemies, but also to be able to play games advantageously in cooperation with human teammates.
Capture the Flag: the emergence of complex cooperative agents | Deep Mind
https://deepmind.com/blog/capture-the-flag/
AI's study of playing games with humans has become a hot topic in 2017, with the result that AI of OpenAI development won the human with "Dota 2" . Also, Deep Mind has been studying AI to play "StarCraft 2" .
This time, "FTW" developed by Google's Deep Mind plays " Quake III Arena " released in 1999. "Quake III Arena" is an FPS for multiplayer, and it is a title that is popular enough to hold the tournament even now. I played a flag-taking game called "Capture the Frag (CTF)" in this "Quake III Arena" and learned with the aim of playing with a team with a team.
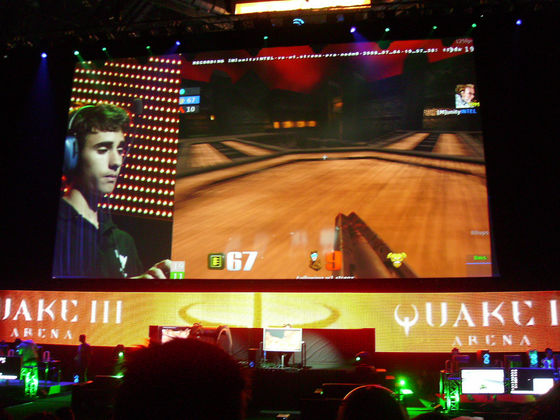
by gamebouille
CTF is divided into two teams to play against each other, and points are added when they take the flag at the opponent team's position and return to their own team. Although it looks like a simple rule, you must change the opponent and movement you want according to the situation, such as having to defeat the player who has the flag if you take the flag on the opponent team, at CTF The research team evaluates that the required movement is complicated.
The map which will be the stage of the battle does not keep using the same thing, it said that the map was changed for each match. By doing this, the FTW has to learn a versatile strategy rather than memorizing the layout of the map. Furthermore, in order to grow AI in the same way as humans, instead of reading the parameters in the game directly like the conventional game AI, let us recognize the pixels on the screen just like humans, I am operating with a rate controller.
Deep Mind's research team randomly matched 40 human players and 30 FTW agents and let them play over 450,000 CTFs. Recurrent (regression type) neural networks are formed between each agent, and learn to do endogenous motivation from game points furthermore. This will allow you to play CTF at a higher level.
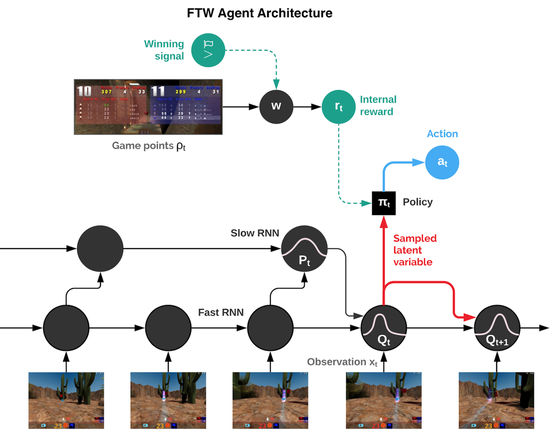
In the following movie, you can see how the FTW agent actually played the CTF with human beings together.
Human-level in first-person multiplayer games with population-based deep RL - YouTube
The graph below shows growth of FTW. The horizontal axis is the number of games, and the vertical axis is the number indicating the strength of the player called Elo rate, the higher the higher the player is. The light blue line shows the Elo rate of the FTW, but when the agent of the FTW has already played the CTF 150,000 times, it already exceeds the "average human player's Elo rate (dotted line written as Average Human)" Before reaching 200,000 times, it exceeds the "strong human player's Elo rate (dotted line written as Strong Human)". By the time I play CTF 450 thousand times, I record the top score.
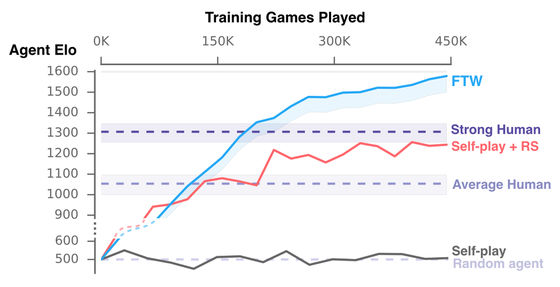
Also, from the graph part "Agent population" displayed in the upper right of the following movie, you can see how each agent of FTW gradually gives up the Elo rate according to the number of times.
Capture the Flag: FTW agents training progression - YouTube
In order to handle a large amount of CTF, FTW not only covers the part of "to defend your team's position" and "to control opponent's position", but also to "cooperate with human beings" He was also successful in acquiring behavior. These actions that collaborate to support each other are said to be obtained in the course of reinforcement learning and evolution at the group level, the FTW development team said that reinforcement learning will further improve collective-level training methods, We are commenting that we aim to develop an AI agent that can eventually form a team with people stably.
Related Posts: