Meta releases next-generation multimodal AI 'Llama 4,' adopting MoE architecture to boast high performance comparable to competing models
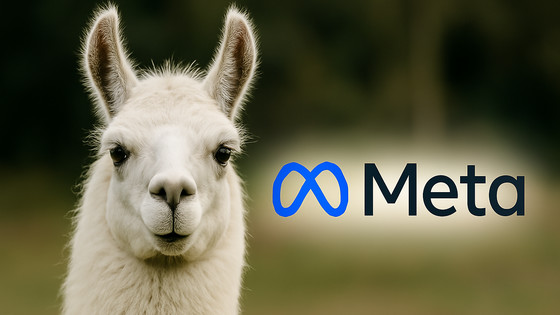
Meta has officially announced its next-generation AI model, the Llama 4 series. The Llama 4 series is composed of multiple models with different performance, scale, and range of applications, and is said to have achieved significant performance improvements from the previous generation and to be comparable to competing AI models. The biggest feature is the efficient model architecture called '
The Llama 4 herd: The beginning of a new era of natively multimodal AI innovation
https://ai.meta.com/blog/llama-4-multimodal-intelligence/
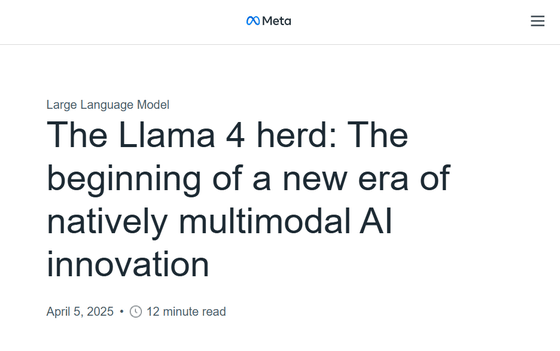
The Llama 4 series is a native multimodal model that is designed from the start to handle multiple information formats, including not only text but also images and videos, in an integrated manner. In addition, the MoE architecture selectively operates only the most suitable specialized models for each task, called 'experts,' to maintain high performance while reducing resource waste.
In addition, Llama 4's underlying technology includes many innovations, such as a new position embedding method called 'iRoPE (Improved Rotary Position Embeddings)' and a new pre-learning strategy called 'MetaP (Meta's Progressive Pretraining)'. Meta claims that these new technologies are designed to improve the scalability, accuracy, and stability of the model, and are the key to improving Llama 4's performance.
First, iRoPE is an improved version of the conventional RoPE (Rotary Position Embedding) that aims to alleviate the degradation of accuracy in long-text context processing. RoPE is used to incorporate token order information into the transformer, but it was known that its performance degrades with long inputs. To address this issue, iRoPE aims to stabilize scaling and inter-token correlation, which makes it possible to obtain highly accurate output even when dealing with very long codes, documents, and conversation histories.
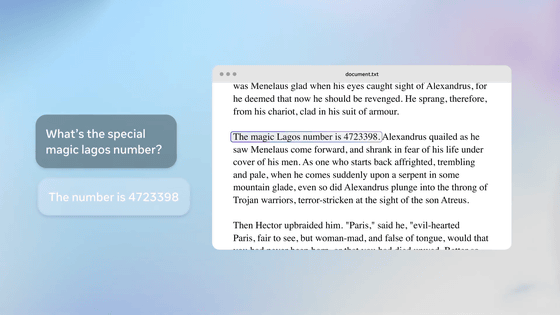
MetaP is a learning method that addresses the learning difficulties of model scaling in order to make pre-training for Llama 4 more stable and efficient. MetaP uses smaller models and simpler datasets in the early stages of learning, and gradually expands the model size and data complexity to achieve stable convergence and high-performance final models. Meta claims that MetaP has succeeded in realizing a multi-modal model capable of integrated understanding and inference.
In addition, while typical MoEs have the problem of bias in the selection of expert models, Llama 4 introduces a new routing mechanism that controls the diversity and balance in expert selection for each token, which is said to be the factor that achieves both high accuracy and efficiency.
At the time of writing, there are three models in the Llama 4 series: Llama 4 Scout, Llama 4 Maverick, and Llama 4 Behemoth.
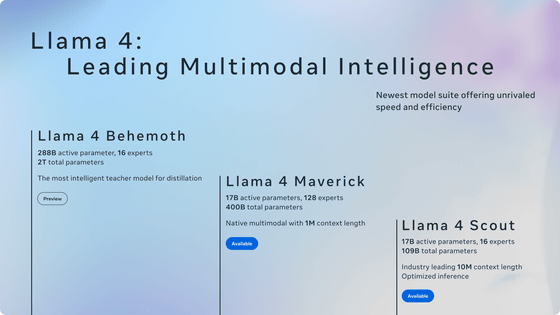
Of the three models, the smallest configuration, 'Llama 4 Scout,' has 17 billion active parameters and is equipped with 16 experts. With a total of 109 billion parameters, it is a lightweight model that can run on a single NVIDIA H100 GPU, but supports a very long context window of 10 million tokens. Meta also claims that it outperforms competing models such as Gemma 3, Gemini 2.0 Flash-Lite, and Mistral 3.1. Llama 4 Scout is particularly good at image recognition and text association.
'Llama 4 Maverick' has 17 billion active parameters and is equipped with 128 experts. The total number of parameters is 400 billion, and it can be run on a single
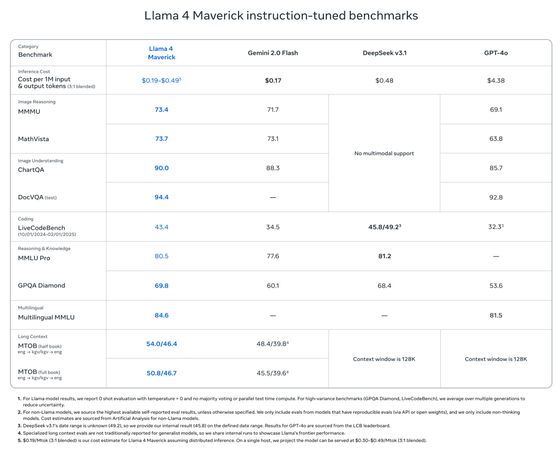
However, IT news site
Okay Llama 4 is def a littled cooked lol, what is this yap city pic.twitter.com/y3GvhbVz65
— Nathan Lambert (@natolambert) April 6, 2025
The top-of-the-line model, Llama 4 Behemoth, is a gigantic model with 288 billion active parameters and 16 experts, with a total of 2 trillion parameters. According to Meta, this model has outperformed GPT-4.5 and Claude 3 Sonnet in benchmarks in the STEM field, and is particularly accurate in mathematics, programming, and science-related tasks. However, at the time of writing, it is still in training and has not been released.
The Llama 4 series supports more than 200 languages, with 10 times more multilingual tokens than the previous generation, Llama 3. Meta also says that it has significantly reduced the rejection rate for topics with a lot of political and social debate, and is working to reduce bias.
These new models will be gradually incorporated into Meta's AI assistant, and at the time of writing, they are available on WhatsApp, Messenger, Instagram, and the web browser version of MetaAI . In addition, the models for Llama 4 Scout and Llama 4 Maverick are available on llama.com or Hugging Face , and model access for research purposes will be made available to the research community in the future.
Related Posts:
in Software, Posted by log1i_yk