Some studies that use Wi-Fi to identify people and objects behind walls are found to be flawed, warning against a research field where fraud is widespread.
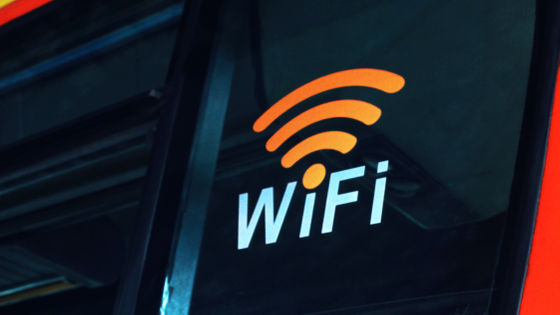
In recent years, there has been active development of technology that uses Wi-Fi radio waves
Researchers Misrepresenting the Capability of Human Pose Estimation from WiFi Channel Strength Indicators | by Andrew Walsh MD. PhD. | Medium
https://medium.com/@tsardoz/researchers-misrepresenting-the-capability-of-human-pose-estimation-from-wifi-channel-strength-4ec4d2f871a4
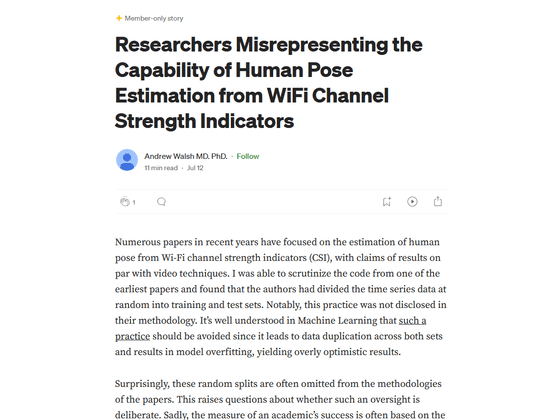
In recent years, many studies have been conducted to estimate human behavior and posture using Wi-Fi channel strength index (CSI), and some studies have found that ``results are as if you were watching footage shot with a camera.'' 'You can get it,' he claims.
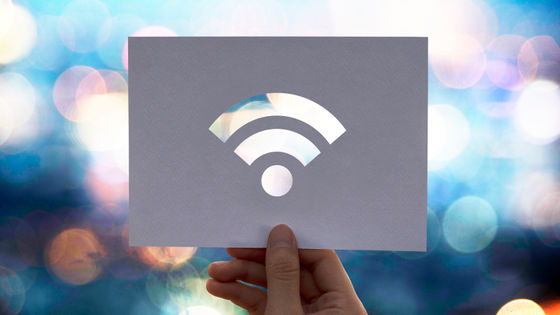
However, Mr. Walsh, who examined the data of a research paper on Wi-Fi CSI, reported that it was confirmed that the research team randomly distributed time series data into training set and test set. In general, in machine learning, it is considered that random distribution of time series data should be avoided, as overlapping data in both sets can lead to overfitting of the model and produce excessively good results. I am. Also, according to Mr. Walsh, in the paper he reviewed, there was no mention of the sorting of time-series data in the paper.
Furthermore, Walsh pointed out, ``Despite this random allocation, there is more than one paper that does not describe the method.'' 'This raises the question of whether this method omission was intentional,' Walsh said.
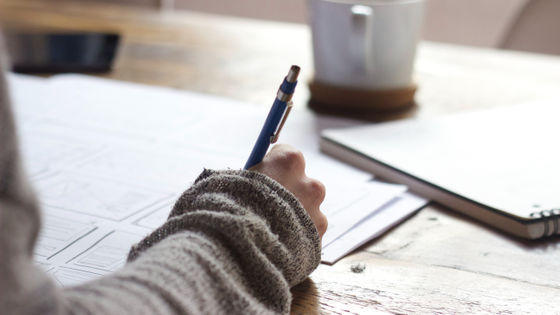
Regarding the omission of data in papers, Walsh said, ``Sadly, the measure of success for academics is based on the amount of papers published in prestigious journals. In order to increase the number, researchers may encourage sloppy experimental methods, fraud, and misrepresentation of methods.The number of papers using flawed approaches to machine learning, such as this one, has increased in recent years. 'Therefore, it is possible that this is not simply an omission in the research method, but rather an intentional strategy to surpass the quality of previous research.'
According to Mr. Walsh, the first paper that took this approach set a bad precedent, leading to a number of subsequent flawed papers.
In order to verify these papers, it is necessary to examine the codes used in the studies in question to determine whether random allocation occurred. However, it is extremely rare for research teams to release the source code of their experiments, making verification difficult.
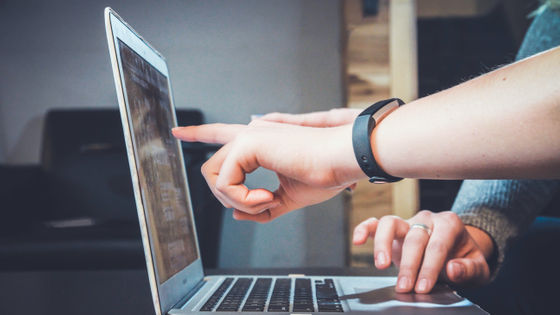
Furthermore, Mr. Walsh warns of the prevalence of fraud in certain fields of research. In fact, Mr. Walsh obtained the code used in a paper and verified it, and confirmed that the time series data was randomly distributed. Although they submitted counter-evidence to the academic research organization IEEE , they reported that the complaint was quickly withdrawn.
“Peer review is extremely important to gain the trust of other scientists. However, if misconduct is widespread in a field, it may be difficult for peer reviewers to do so because they are researchers in the same field. They may have intentionally overlooked fraud in order to dismiss criticism of their research,' Walsh said.
Related Posts:
in Science, Posted by log1r_ut